, Dilip R. Karnad2 and Snehal Kothari3
(1)
Cardiac Safety Services Quintiles, Durham, North Carolina, USA
(2)
Research Team, Cardiac Safety Services Quintiles, Mumbai, India
(3)
Cardiac Safety Services Global Head, Cardiac Safety Center of Excellence Quintiles, Mumbai, India
The analytical methodologies introduced in this chapter can be employed in the analysis of efficacy and safety data: our interest lies with their use in the domain of cardiovascular safety.
6.1 Introduction
This chapter introduces four analytical methodologies that can be used to analyze efficacy or safety data. Each is described in turn, and our interest then focuses on their employment in the domain of cardiovascular safety.
Meta-analysis is a statistical technique that brings together data from multiple studies addressing the same topic, thereby employing a (potentially much) larger database to answer a research question than was possible on the basis of data from each of the individual studies. Meta-analysis is useful in both clinical research and clinical practice. In the realm of clinical practice, physicians need to be familiar with the most recent and relevant research published in the medical literature to enable them to practice evidence-based medicine. While research reports of individual randomized clinical trials are helpful to physicians, it has become very difficult (and arguably impossible) for them to read every publication of relevance to their particular specialty. Two types of approaches that bring together the results from multiple studies into a single publication have therefore attracted increasing attention. The first is qualitative in nature, while the second is quantitative. Systematic reviews are descriptive in nature: they “collate, compare, discuss, and summarize the current results” in a particular field (Matthews 2006). Meta-analysis goes a step further, providing a statistical technique to combine results from multiple individual trials and then to use this data set to conduct a new analysis that could not be conducted on the basis of any of the individual trial’s data sets.
Group sequential designs facilitate interim analyses. As the name connotes, these analyses are performed during the conduct of a study, i.e., before the study has gone to the full term as outlined in its study protocol. Several interim analyses may be performed during an ongoing clinical trial at various pre-identified points. Each interim analysis conducted utilizes all of the data that have been collected to the point when a given analysis is conducted. This analytical approach has the strength that it may reveal compelling evidence that the clinical trial should be stopped (terminated) at the time of a particular interim analysis because there is already compelling evidence that the drug is effective, that it is toxic, or that, even if the trial were to carry on to its conclusion, there would very likely still not be compelling evidence of efficacy or toxicity.
The size and hence geographical distribution of investigational sites needed for the large cardiovascular safety outcome trials discussed in Chap. 13 mean that there can be a considerable degree of variability in the “identification” of cardiovascular endpoints of interest since classification of events as “actual” study endpoints is a partially subjective process based on the application of a complex set of medical endpoint criteria to an often complex clinical event. Regulatory agencies therefore require the centralized adjudication of these events to control for the impact of this variability in “identification” and thereby to generate data for use in statistical analyses that are as standardized as possible.
Composite endpoints are used in these cardiovascular outcome safety trials since the occurrence of individual events is typically low. The statistical rationale for employment of these endpoints is explained.
6.2 Meta-analysis
The underlying logic behind meta-analysis is straightforward: by creating a larger data set than existed for any of the individual trials included, increased power is provided to detect statistically significant treatment effects and to facilitate assessment of the magnitude of a treatment effect more precisely. For many reasons, there are likely to be more than one clinical trial that has addressed the same research question: combination of information from multiple smaller studies that are inconclusive sometimes paints a picture that is more compelling.
6.2.1 More Informative Nomenclature: The Term Meta-methodology
While the term meta-analysis is now well embedded in the medical literature, the term meta-methodology represents more informative nomenclature (Turner and Durham 2014). The name meta-analysis is typically used in the literature to refer to the entire process of conducting such an analysis: however, it does not adequately capture and emphasize the need for methodological rigor in the full array of actions required. Certainly, an analysis is conducted, and the term meta-analysis is entirely appropriate when discussing that segment of the process. However, determining the individual data sets from which the new data set is created, choosing the appropriate analytical model, and presenting the statistical results of the meta-analysis and their interpretation with scientific and clinical decorum are also critically important.
6.3 The Fundamentals of Meta-methodology
Meta-methodology facilitates a quantitative evaluation of the combined evidence provided by two or more individual clinical trials that have addressed the same research question (Turner and Durham 2014). Most frequently, it involves the statistical combination of summary statistics from each trial included in the analysis, i.e., mean treatment effect point estimates and the variances associated with those estimates. In these cases, study-level data are employed in the analysis. However, it can also involve analyses performed using the raw data from each participant in each trial contributing to the new data set. In such cases, the meta-analysis is said to be performed on participant-level data. While the latter is always preferable if possible, it can be challenging to access such data for a variety of reasons, including availability of participant-level data due to their proprietary nature and, in some cases, obtaining approval from an institutional review board.
The fundamental steps in study-level meta-methodology include the following (Turner and Durham 2014):
- 1.
Establishing rules for determining whether or not the data from an identified study report of potential relevance will be incorporated into the new data set
- 2.
Identification of all potentially relevant studies
- 3.
Data extraction, i.e., obtaining the treatment effect point estimate and its variance for each study to be included in the analysis
- 4.
Data analysis, i.e., conduct of the meta-analysis itself
- 5.
A visual inspection and quantitative test of homogeneity
- 6.
Evaluation of the robustness of the result obtained from the meta-analysis
- 7.
Dissemination of results, interpretations, and conclusions at scientific conferences and in medical and scientific publications
6.3.1 Determining the Studies to Be Included
One straightforward approach is to decide to include every study that can be identified, whether identified from a literature search or other routes. A counterargument to this approach, however, is that some of the studies that will be identified will almost certainly be “better” than others and that “less good” studies should perhaps not be included. In the latter case, strict a priori inclusion and exclusion criteria that operationally define entry into the analysis must be stated by the researchers conducting the meta-methodological investigation in advance of searching for studies. In this sense, while no data are being collected in a new clinical trial, it is highly advisable to write a “meta-methodology protocol” before executing a meta-methodology in exactly the same way that a study protocol is written ahead of conducting a new trial.
6.3.2 Identification of all Potentially Relevant Studies
Identification of all studies in the published medical and scientific literature that may potentially be included has become much easier with the advent of computer search engines and web-based tools, but it can still be a challenging task. One particular difficulty is looking for, and, when located, obtaining unpublished data. The issue of publication bias is a particularly noteworthy one in this context (Turner and Durham 2009). Piantadosi (2005, pp 582–3) defined publication bias as a “tendency for studies with positive results, namely those finding significant differences, to be published in journals in preference to those with negative findings.” In a similar vein, Steward and colleagues (2005, p 262) commented as follows:
Overt or subconscious pressures such as the wish to bolster research ratings, the need to sell journals or simply the desire to deliver good news can lead to results being presented in an over-favourable light, or to publishing only a message of progress of progress or improvement. This is of course potentially very damaging, and in the context of systematic review it is important that we do all we can to minimize sources of potential bias, including those associated with publication and reporting.
Additional factors of relevance here are that studies with positive results are more likely to be published in English language journals, and hence located more readily by some large computer search engines, and that some studies are never submitted for publication since those conducting the study determine subjectively that the results are unfavorable; however, “unfavorable” is operationally defined by the researchers. This means that it is very important for researchers conducting a meta-analysis (meta-analysts) to do everything possible to locate any unpublished study results. As Kay (2007) wryly observed, “to ensure that a meta-analysis is scientifically valid, it is necessary to plan and conduct the analysis in an appropriate way. It is not sufficient to retrospectively go to a bunch of studies that you like the look of and stick them together!”
6.3.3 Data Extraction and Acquisition
When conducting a study-level meta-analysis, this step is straightforward: two items of data are acquired from each study report:
A measure of the treatment effect in that study, represented by the treatment effect point estimate presented in the report
The variance associated with the treatment effect, often operationalized as a two-sided 95 % confidence interval placed around the treatment effect point estimate
6.3.4 Executing the Actual Meta-analysis
Meta-analysts must decide before conducting the analysis whether to employ a fixed-effects or a random-effects analysis model. These differ in the degree of influence each individual study’s treatment effect is allowed to exert mathematically on the new treatment effect point estimate calculated by the meta-analysis: this degree of influence is operationalized by the weight assigned to each treatment effect.
Each study included in the analysis contributes the same two pieces of information: the treatment effect point estimate found in that study and its associated variance. Therefore, if the analysis incorporates 100 studies, 100 treatment effect point estimates are included. However, each item of information does not necessarily impart the same influence (in statistical nomenclature, does not carry the same weight) when determining the result of the analysis: studies whose treatment effect point estimates are weighted more heavily will exert a greater influence on the final result of the analysis than those whose treatment effects are weighted less heavily. The weight accorded to each study-specific treatment effect is determined computationally according to the rules of the analysis model adopted. Both the fixed-effects model and the random-effects analysis model use the precision of each study’s treatment effect point estimate when assigning its weight: higher precision, conveyed by narrower confidence intervals around the treatment effect point estimate, affords more weight. However, and very importantly, when determining the weight assigned to each study’s treatment effect point estimate, the random-effects model also uses an estimate of how different from each other the studies are in various characteristics, such as the nature of the study population, number of participants in each treatment group, length of treatment periods, participants’ concomitant illnesses, and the quality of measurements made during the trial. In cases where the natures of the studies are very similar, the difference in the results generated by the two analysis models would be small: if there were no differences at all, the results would be the same. However, the greater the degree of difference between the studies incorporated in the analysis, the greater the difference between the results generated by the two models, and the more important it becomes to employ the random-effects model in situations where the studies included in the meta-analysis do indeed differ from each other.
Allowing for the existence of differences between studies included in the meta-analysis is an intuitively sensible feature of the random-effects model, since in most circumstances when comparing multiple studies conducted by multiple independent research teams, it would be very surprising if the studies incorporated did not vary from each other. While the precise methods of quantitatively estimating the differences between studies (i.e., the additional component of the determination of the weight assigned to each study’s treatment effect point estimate in addition to its precision) need not be discussed here, it is important to be aware of the consequences of employing a fixed-effects model when a random-effects model is the more appropriate choice. The random-effects model tends to generate wider confidence intervals around the newly created treatment effect point estimate, indicating less precision. If a fixed-effects model is employed when there are considerable differences between the studies included, the confidence interval will be narrower than it would have been had the random-effects model been used, and thus the confidence placed in the result of the analysis will be greater than it should be. Narrower confidence intervals also make it easier to achieve a statistically significant result, an outcome used by some meta-analysts to ascribe more gravitas to their results than they deserve: consideration of a result’s clinical significance is more important, and the clinical assessment of a treatment effect is “a completely separate assessment” from its statistical significance (Durham and Turner 2008).
An illuminating example can be found in a paper by DiNicolantonio and colleagues reporting a study-level analysis comparing the beta-blocker carvedilol with four β-1 selective beta-blockers (DiNicolantonio et al. 2013). As part of the overall meta-methodological approach, three trials including 644 participants with acute myocardial ischemia and evaluating relative reductions in all-cause mortality were combined for meta-analysis. Of particular interest here is that the authors reported results using both a fixed-effects model and a random-effects model. For the fixed-effects model, the result was as follows:
Of note is that both the lower and the upper limits of the confidence interval lie below zero. This result can therefore be interpreted in this manner:

The result from this meta-analysis indicates a statistically significant reduction in all-cause mortality associated with carvedilol in the general population. The result is compatible with a reduction as great as 68 % and as small as 6 %, and our best estimate is a reduction of 45 %.
The result for the random-effects model was as follows:
Of note in this case is that the lower and the upper limits of the confidence interval span zero. For this model, the result is therefore interpreted in this manner:

The result from this meta-analysis does not indicate a statistically significant reduction in all-cause mortality associated with carvedilol in the general population. The result is compatible with a reduction as great as 74 % but also compatible with an increase as great as 12 %. Our best estimate is a reduction of 44 %.
The important point made by this example is that the best estimates of the truth in the general population provided by the fixed-effects and random-effects models are essentially the same (relative reductions in all-cause mortality of 45 and 44 %), but the statements of the statistical significance of the results are completely different due to the widths of the respective confidence intervals. For the fixed-effects model, the lower and upper limits of the confidence interval (0.32 and 0.94, respectively) both fall below 1.0, hence the attainment of statistical significance. For the random-effects model, the lower and upper limits (0.26 and 1.12, respectively) of this wider confidence interval lie on either side of 1.0, hence the failure to attain statistical significance. The authors are to be commended for presenting the results generated by both analysis models.
6.3.5 Testing for Homogeneity
The statistical theory underpinning meta-analysis assumes that the study-specific estimates of the treatment effect are (relatively) homogenous. Homogeneity is present when the study-specific estimates are similar in magnitude and direction to the estimate of the treatment effect resulting from the combined analysis. Heterogeneity can arise from differences between studies, such as the possibilities noted in the previous section. Since the objective is to calculate a well-justified combined estimate of the treatment effect of interest, a formal evaluation of homogeneity following a visual graphic inspection of the combined effect against each individual effect is a recommended strategy.
This formal evaluation involves a statistical test, such as the Cochran Q test. Homogeneity (also expressed as lack of heterogeneity) is indicated by a statistically nonsignificant result. While general acceptance of the α = 0.05 criterion provides a “line in the sand” that is useful in certain circumstances, blind adherence to characterizing a result as either statistically significant or not statistically significant using the α = 0.05 level is not necessarily a clinically meaningful strategy. In this context, a statistically nonsignificant Cochran test can be (mis) interpreted to state that there is no heterogeneity present. That is, a fallacious argument can be made that the lack of statistically significant evidence of heterogeneity represents an all-or-none statement of its complete absence.
6.3.6 Evaluating Robustness
Having calculated the result of the analysis, it can be informative to assess its robustness. In any combined analysis, some of the studies included will be larger than others, and sometimes a small percentage of included studies can be considerably larger than the majority of others. The nature of the calculations performed here mean that the larger trials tend to influence the result more, since they tend to have greater precision.
It can therefore be helpful to assess the robustness of the overall conclusion by performing the analysis without the data from the largest study or studies to see if the results remain qualitatively the same. If they do, then the result of the primary overall analysis is deemed robust. If they do not, confidence in the overall result can be undermined. Moreover, if the results are considerably different, it simply may not be appropriate to present the combined result alone and make statements based on it.
6.3.7 Disseminating the Results, Interpretations, and Conclusions to Various Audiences
The results presented upon completion of the meta-analysis would typically include the following:
The treatment effect point estimate for each individual study included in the analysis and the confidence interval (often the two-sided 95 % CI) placed about each study’s estimate
The overall treatment effect point estimate calculated in the meta-analysis and its confidence interval (often a two-sided 95 % CI)
This information can be displayed in tabular form or in a graphical form called a confidence interval plot.
6.3.8 Additional Challenges in Meta-methodology
Meta-methodology has both strengths and weaknesses. As Turner and Durham (2009, p 254) commented:
As is true across all research methodology, if the correct study design has been employed and rigorous methodology has permitted the acquisition of optimum-quality data, the computational analysis is typically not difficult. What is more difficult is the interpretation of the results and the appropriate degree of restraint needed to disseminate one’s conclusions in a responsible manner. Given all of these considerations, the conduct and communication of a meta-analysis must be undertaken carefully, diligently, and responsibly.Stay updated, free articles. Join our Telegram channel
![]()
Full access? Get Clinical Tree
![]()
![]()
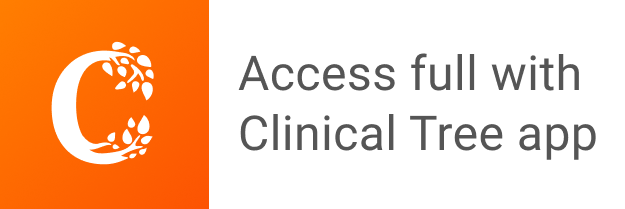