A wide variety of multivariable risk models have been developed to predict mortality in the setting of cardiac surgery; however, the relative utility of these models is unknown. This study investigated the literature related to comparisons made between established risk prediction models for perioperative mortality used in the setting of cardiac surgery. A systematic review was conducted to capture studies in cardiac surgery comparing the relative performance of at least 2 prediction models cited in recent guidelines (European System for Cardiac Operative Risk Evaluation [EuroSCORE II], Society for Thoracic Surgeons 2008 Cardiac Surgery Risk Models [STS] score, and Age, Creatinine, Ejection Fraction [ACEF] score) for the outcomes of 1-month or inhospital mortality. For articles that met inclusion criteria, we extracted information on study design, predictive performance of risk models, and potential for bias. Meta-analyses were conducted to calculate a summary estimate of the difference in AUCs between models. We identified 22 eligible studies that contained 33 comparisons among the above models. Meta-analysis of differences in AUCs revealed that the EuroSCORE II and STS score performed similarly (with a summary difference in AUC = 0.00), while outperforming the ACEF score (with summary differences in AUC of 0.10 and 0.08, respectively, p <0.05). Other metrics of discrimination and calibration were presented less consistently, and no study presented any metric of reclassification. Small sample size and absent descriptions of missing data were common in these studies. In conclusion, the EuroSCORE II and STS score outperform the ACEF score on discrimination.
Risk prediction models have been used in cardiac surgery for >30 years. Predictive models used in this setting allow for risk stratification, which may facilitate informed consent and affect considerations for the timing and choice of surgical intervention. For example, in patients with aortic stenosis, high surgical risk as determined by the Society for Thoracic Surgeons 2008 Cardiac Surgery Risk Models (STS) score may be used to justify a transcatheter rather than surgical valve replacement. Because all-cause mortality in the perioperative period is a leading cause of death in many countries (e.g., third most common cause in the United States), it is particularly desirable to have predictive tools that can discriminate between high-risk and low-risk patients. However, there is a broad range of predictive models that are available in this field, leaving clinicians with an assortment of models to choose from. Moreover, there are concerns from other fields of prognostic research that the predictive literature often suffers from poor methodologic quality, lack of external validation, and worsening predictive performance on external validation. To the best of our knowledge, the evidence on the relative utility of multivariable models used to predict mortality in cardiac surgery and the reporting practices in this literature have never been systematically studied. Here, we evaluated the evidence on comparisons that have been made among the European System for Cardiac Operative Risk Evaluation (EuroSCORE) II, the STS score, and the Age, Creatinine, Ejection Fraction (ACEF) score. These are 3 established multivariable models used to predict perioperative mortality in the setting of cardiac surgery that have been adopted by recent guidelines. We systematically reviewed the published reports related to the relative performance of these models, performed meta-analyses related to the discrimination performance of these models, and evaluated the presence of reported bias.
Methods
We used the PRISMA checklist of preferred reporting items for systematic reviews and meta-analyses in the planning of this study and elaboration of this article. The methods and protocol used in this study were adapted from a similar study that considered comparisons of risk prediction models for cardiovascular disease in asymptomatic patients.
We used recent guidelines published in the United States and Europe to select 3 multivariable prediction tools that have been explicitly recommended for use in preoperative risk stratification during myocardial revascularization or cardiac valvular surgery. These models (hereafter referred to as “core” models) include the EuroSCORE II, STS score, and ACEF score. Although some additional prediction tools were mentioned in these guidelines, they were excluded from the list of core models because their use was not recommended, their use was only recommended for percutaneous coronary intervention (rather than surgery), and/or their use was recommended for assessing medium- to long-term outcomes (rather than perioperative outcomes).
We searched Medline (last update October 12, 2015) for articles that made a comparison between at least 2 of the 3 core risk models (see Appendix for search terms). Two independent reviewers (PGS and JDW) initially screened articles at the title and abstract level without year or language restrictions. Potentially eligible articles were downloaded and examined in full text. Article references, review articles, and a meta-analysis identified by our search were scrutinized for additional articles that met study eligibility. Furthermore, Web of Knowledge (v 5.19) and Scopus (last update January 6, 2016) were used to review all the citing reports of the original articles describing the EuroSCORE II, STS score, and ACEF score to identify whether there were any additional eligible studies.
Two investigators (PGS and JDW) independently assessed the articles for eligibility, and persisting discrepancies were resolved by consensus and arbitration by a third investigator (JPAI). Articles were eligible for inclusion if they compared at least 2 of the 3 core models for the prediction of 1-month and/or inhospital mortality in cardiac surgery. We focused on 1-month and/or inhospital mortality to be inclusive of the common definitions used for perioperative mortality, including the end points used in the development of the EuroSCORE II (inhospital mortality), STS score (inhospital or 30-day mortality), and ACEF score (inhospital or 30-day mortality). Studies were eligible regardless of whether any additional prediction models (“noncore” models) were also assessed.
Although articles that exclusively studied percutaneous interventions (such as percutaneous coronary intervention and transcutaneous aortic valve replacement/implantation) were excluded, we did not exclude comparisons made in studies that included mixed cohorts of surgical patients and those who underwent percutaneous interventions. Whenever mixed cohorts were used, we used only the subset of data on surgical patients, as the core models were developed for prediction in cardiac surgery.
For studies that met inclusion criteria, 2 investigators extracted the first author, PubMed ID, year of publication, journal, study design, study interval, geographic region of the study, study population, predictive tools that were assessed, type of surgery, sample size, and number of events. For each nonoverlapping cohort of patients, we identified the perioperative mortality endpoint that allowed for comparisons among the maximum number of core models. We counted as a comparison any attempt to explicitly compare the performance of 2 risk models on any metric of discrimination, calibration, or reclassification.
As was done previously, if a single article allowed for comparisons among all 3 core models, information for each eligible pairwise comparison was extracted. We focused on outcomes pertaining to the entire population, unless only subgroup data were reported. In those cases, each eligible subgroup was considered a unique population and data were extracted accordingly. When we suspected that a patient cohort overlapped across multiple articles that met our eligibility criteria, we contacted the authors to determine the nature of the possible overlap to prevent redundant model comparisons within the same cohort. If additional comparisons could be made to noncore predictive models within eligible reports, details about each of those pairwise comparisons were also extracted in the same manner as mentioned earlier. In cases where the precise predictive model used was ambiguous because of overlapping nomenclature (e.g., in differentiating between the EuroSCORE models), we relied on the citations used to the index articles and our best judgment to classify the model.
For each comparison, we recorded the available metrics of discrimination (AUC with associated confidence intervals and p values, p values for comparisons of AUC, Brier score, D-statistic, R 2 statistic, and Somers D xy ) that were present. Similarly, we recorded all metrics of calibration (including observed-to-expected [O:E] event ratios and the p value for the Hosmer-Lemeshow goodness-of-fit statistic) that were included for each outcome of each prediction model of each included study. In cases where the O:E ratio was not explicitly available, we calculated it with information from the article. Finally, we searched for the presence of any metric of reclassification, such as the number of patients who were reclassified to a different risk group using an alternative predictive model.
Articles were scrutinized for any discussion related to the presence of missing data on examined outcomes and on variables included in the prediction models. For eligible articles, we recorded how the investigators managed the missing data. We also assessed whether the authors made any statement about the sample size of the study.
For every study that met inclusion criteria, we considered all possible pairwise comparisons between the assessed risk models. For discrimination, we recorded all the AUC values and SDs thereof, the model with the highest AUC, whether a statistical test had been performed making a comparison between AUCs, and whether the relative difference in the AUC was >5%. The choice of a 5% threshold was selected for descriptive purposes, as was performed in a similar study. For each of the comparisons between the core risk models, we performed a meta-analysis to calculate a summary difference in AUCs between the 2 models with the information that we had extracted from the original reports. To calculate the standard error of the difference between AUCs, we used the Hanley and McNeil p value from one of the articles that met our inclusion criteria and information from that paper to impute r, the correlation introduced between the 2 AUCs. We used this single estimate for r (0.010) for all calculations of the standard error of the difference between the 2 areas. We also performed a sensitivity analysis setting r to 0.30 and 0.50. Heterogeneity for the studies was quantified by calculating the Q statistic and I 2 statistic for each meta-analysis.
We also performed meta-analyses of the AUC estimates, separately for each of the 3 core models. This approach does not account for the paired comparisons but offers insights about the absolute magnitude of the AUC and its heterogeneity across studies for each of the 3 core models. Data on AUC differences and absolute AUCs were combined with random effects (DerSimonian and Laird method) modeling. Fixed-effect estimates were also obtained, but they should be interpreted with caution in the setting of significant heterogeneity.
Regarding calibration, we recorded which model had the O:E ratio that was closest to 1. Finally, we searched for any description by the investigators to capture reclassification, such as whether 1 model was able to reclassify participants to different risk groups using a separate, non-nested model.
Analyses were done in SAS Enterprise Guide 6.1 (SAS Institute, Cary, North Carolina). The meta-analyses were performed in R (version 3.2.3) using the metafor package.
Results
We identified 265 articles in our Medline search and selected 49 articles for a review in full text. Of these 49 articles, 28 were excluded for various reasons ( Figure 1 ). After reviewing the references of all articles that met our inclusion criteria, the references in the meta-analysis found in our original search, and all articles citing the index papers for the core risk models using both Web of Knowledge and Scopus, we identified 1 additional article for inclusion. This left 22 articles with at least 1 comparison between the core risk models.
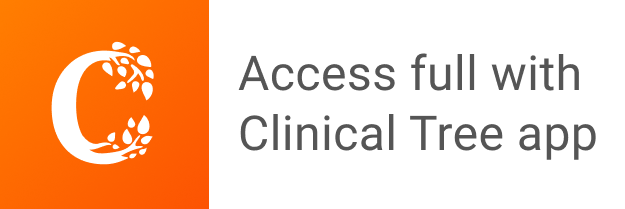