Fig. 1
Omics’ approaches

Fig. 2
Metabolomics workflow
Human metabolome consisted of approximately 4229 endogenous metabolites in serum sample (Psychogios et al. 2011). Metabolites represent the intermediate and end products of metabolic reactions and cellular processes, and may have diverse functions in different organisms. Metabolites usually have low molecular weight of less than 1500 Dalton, and biological molecules including carbohydrates, fatty acids, and amino acids can be considered as metabolites. Metabolites also vary depending on their polarity, solubility and volatility. For instance, amino acids are hydrophilic polar metabolites, while lipids are hydrophobic non-polar metabolites.
3 Targeted and Untargeted Metabolomics
An appropriate experimental design is required to ensure that the metabolomic data is able to answer the questions of interest (Fig. 2). Two distinct metabolomic strategies, namely targeted and untargeted approaches have been applied to the analysis of metabolites. Targeted metabolomic approach is on the basis of hypothesis-driven manner and also used for biomarkers validation (Griffiths et al. 2010; Wei et al. 2010). Targeted metabolomics is the measurement of a select group of metabolites, typically focusing on one or more related pathways of interest (Dudley et al. 2010). It is normally performed using mass spectrometry (MS) based approach. By taking this approach, a novel association between metabolites and diseases may be revealed and enables a more comprehensive understanding on metabolic functions and pathways (Roberts et al. 2012).
In contrast to targeted metabolomics, untargeted approaches allow the unbiased detection of full set of metabolites in the biological samples. This approach offers the opportunity for the discovery of novel metabolites and metabolic pathways without prior knowledge of the identified metabolites (Patti et al. 2012). Therefore, untargeted metabolomics is classified as discovery-phase or hypothesis-generating research. Untargeted metabolomics can be performed by nuclear magnetic resonance (NMR) and MS approaches, in order to measure and quantify as many metabolites as possible (Alonso et al. 2015). However, the challenging part of this approach is build upon the complexity and pleiotropic nature of metabolomic data as well as the protocol and time required to analyze the massive data sets (Patti et al. 2012; Roberts et al. 2012).
4 Sample Preparation
The initial step in a metabolic profiling is the extraction of metabolites from biological samples. Metabolite extraction varies profoundly depending on study objectives, sample availability (cell, tissue or body fluid) and analytical platform to be used. During sample acquisition, several factors have been identified to interfere with metabolomic outcomes. For instance, gender, age, diurnal variations, diet, exercise and drug consumption may influence the metabolic profile of an individual (Yin et al. 2015). In addition, type of blood-collection tubes (such as lithium heparin, potassium EDTA and citrate), duration between sample collection and processing, transportation, storage and repeating freeze-thaw cycles of samples may also affect the analytical results (Yin and Xu 2014; Yin et al. 2015).
In the consideration of complex, heterogeneous and dynamic nature of metabolome, metabolite extraction can be performed in order to separate and maximize the total number of metabolites detected. The procedure of metabolite extraction is specific to samples types and metabolites of interest (Patti 2011). Liquid-liquid extraction and solid phase extraction are the most commonly used methods for metabolites extraction (Yin and Xu 2014). Metabolites should be quenched immediately to stop metabolism for example by perchloric acid treatment or freezing at −80 °C or liquid nitrogen prior to extraction. During extraction process, different solvents such as methanol, isopropanol, chloroform, acetonitrile or their combinations are used, thus allow the separation of polar and non-polar metabolites and also protein depletion (Bruce et al. 2008; Sellick et al. 2011; Sapcariu et al. 2014). Lipids are usually extracted with chloroform-methanol-aqueous mixtures (Jung et al. 2011). As a result, the upper aqueous soluble phase is consisted of polar metabolites, while non-polar metabolites are remained in the lower organic phase. However, it has been suggested that minimal sample preparation may lead to the production of accurate, reliable, and unbiased data (Dunn et al. 2011).
5 Analytical Instrumentation
High-throughput analytical platforms allow the simultaneous separation and comprehensive detection of metabolites present in biological samples, mainly based on either NMR spectroscopy or MS technologies. These including, but are not limited to 1H-NMR spectroscopy, 13C-NMR spectroscopy, 15N-NMR spectroscopy, 31P-NMR spectroscopy, gas chromatography-MS (GC-MS), gas chromatography-flame ionization detection (GC-FID), capillary electrophoresis-MS (CE-MS), direct infusion-MS (DI-MS) and liquid chromatography-MS (LC-MS). High-resolution magic-angle spinning NMR spectroscopy can be used for metabolic profiling of intact tissues without any pretreatment of samples (Beckonert et al. 2010).
6 Nuclear Magnetic Resonance Based Method
NMR spectroscopy is a rapid and effective technique for untargeted metabolomics, as it can identify and quantify a wide range of metabolites in biological samples. This technique is a non-destructive method and requires only minimal sample preparation. 1H-NMR spectroscopy is the one of most common used methods for metabolomic analysis, and to a lesser extent 13C-, 15N- and 31P-NMR (Keun and Athersuch 2011). The principle of NMR involved the absorption of radiant energy by atomic nuclei (1H) in the presence of a magnetic field (Lane 2012). At the same field, different atomic nuclei within a molecule will generate different resonance frequencies. These signals will provide the chemical and structural information on the detected molecule.
However, NMR has relatively low analytical sensitivity that detects only high-abundance metabolites, thus leads to a requirement for greater initial sample volume (Bothwell and Griffin 2011). Moreover, complex datasets may result in peaks overlapping or similar coupling constants in one-dimensional NMR. This can be overcome by applying two- and three-dimensional NMR spectroscopy methods such as J-resolved spectroscopy, spin-echo correlated spectroscopy, diffusion ordered spectroscopy and ultrafast J-Resolved correlation spectroscopy (Ludwig and Viant 2010; Dunn et al. 2011; Larive et al. 2014). Furthermore, high-resolution magic angle spinning (HRMAS) NMR spectroscopy can be performed on intact tissues without sample preparation (Bothwell and Griffin 2011; Larive et al. 2014). During the process, sample is spun at magic angle (54°44’) to the magnetic field with high speed (2000–6000 Hz), in order to reduce or remove the chemical shift anisotropy and dipole-dipole couplings (Salek et al. 2011).
7 Mass Spectrometry Based Method
MS coupled with liquid chromatography (LC) or gas chromatography (GC), enables the ionization and subsequent separation of the ions and fragment ions according to their mass-to-charge (m/z) ratio (Pitt 2009). In LC-MS, several types of atmospheric pressure ionization methods are used for the ionization of different categories of metabolites. Of which, the most commonly used electrospray ionization is used for the initial screening of unknown metabolites (Xiao et al. 2012). Atmospheric-pressure chemical ionization and atmospheric-pressure photoionization are suitable for the detection of non-polar metabolites and have been widely applied in lipidomic studies (Xiao et al. 2012). There are different types of MS analyzers, including ion trap, time-of-flight, orbitrap, and quadrupole MS (Fuhrer and Zamboni 2015).
The high resolution of GC combined with the MS detection, and more recently GC × GC-MS, provides an excellent system for performing global metabolic profiling, with the help of metabolome databases in the identification of unknown compounds (Patti et al. 2012). However for GC-MS based method, sample derivatization is required prior to data acquisition. For aqueous metabolites, the common practice is to use a two-step derivatization processes (methoximation followed by silylation) prior to GC-MS analysis to reduce polarity and increase thermal stability and volatility (Cheng et al. 2014). The benefits of GC-MS based strategies may include very high resolution, good sensitivity and robustness; these are further enhanced by a two dimensional GC × GC-MS. The metabolites elute from the first column are separated based on volatility and followed by polarity in the second column (Lenz and Wilson 2007).
Compared to NMR and GC-MS, LC-MS based method is able to detect a larger numbers (200–500) of metabolites, and therefore has been recommended for global metabolite profiling (Patti et al. 2012). In many ways, LC-MS analysis can be performed using reversed-phase gradient chromatography, electrospray ionization probe and Z-spray ion source in order to obtain the most comprehensive metabolic profile (Cheng et al. 2014). LC-MS may reduce the interference between analytes and background by improving gaps (ion suppression) or peaks (ion enhancement) signals (Lenz and Wilson 2007). However, it is suggested that identified metabolites have to be confirmed and validated with authentic metabolic standards.
The sensitivity of MS is determined by metabolite’s pK, hydrophobicity and ionization potential (Want et al. 2007). Therefore, quenching and metabolite extraction method as well as sample handling and storage may affect the amount of variability in the sample results. Another limitation of MS is the lower reproducibility as compared with NMR method. Despite these limitations, MS platform still outperforms NMR-based method for the detection of low-abundance and large quantity of metabolites (Theodoridis et al. 2011). MS possesses a greater sensitivity than NMR spectroscopy that capable to detect very low-abundance metabolites at picogram level (Dunn et al. 2011). By showing their respective pros and cons, the combination strategy of MS with NMR spectroscopy has proven to be effective and becomes increasingly popular in metabolomic studies.
In addition, advancement of new technology has been made in utilizing triple quadrupole (QqQ) MS for the quantitatively measurement of metabolites with higher sensitivity and specificity (Patti et al. 2012). QqQ MS consists of precursor ion scan, neutral loss scan, product ion scan, and selected reaction monitoring to filter and detect the metabolites with more sensitive and specific to their molecular weight and structure (Pitt 2009). QqQ MS is coupled with other separation techniques (LC, GC, and CE) and multiple reaction monitoring, in order to enhances its selectivity and sensitivity. Hence, QqQ MS technology provides excellent depth and breadth of metabolome coverage, and is well-suited for targeted metabolome analysis and quantification of metabolites.
8 Data Acquisition and Analysis
Data acquisition and analysis are conducted to identify the particular metabolites that could potentially serve as biomarkers for diseases. Putative identification of metabolites is initially carried out by matching the actual m/z values and theoretical m/z values in various databases such as Human Metabolome Database (http://www.hmdb.ca/), HumanCyc (http://humancyc.org/), LipidMaps (http://www.lipidmaps.org/), MassBank (http://www.massbank.jp/?lang=en) and Metlin (https://metlin.scripps.edu/index.php) (Johnson et al. 2014). Metabolomic data acquisition and processing can be performed by metabolomic softwares such as AMDIS, CODA, HighChem MassFrontier, LECO ChromaTOF, MathDAMP, MetAlign, MZMine, SpectralWorks AnalyzerPro, TargetSearch, WMSM and XCMS (Shulaev 2006; Kind and Fiehn 2010; Patti et al. 2012).
Metabolomics data has been analyzed with a wide range of statistical analyses, in order to reduce the number of variables and examine the difference between groups (Bartel et al. 2013). These analyses can be classified into either univariate analysis of t-test and ANOVA (analysis of variance) or multivariate analysis such as the widely used PCA (principal components analysis), PLS (Partial least squares) regression, and PLS-DA (PLS-discriminant analysis). In addition, metabolomics data have also been analysed using MANOVA (multivariate analysis of variance), ASCA (ANOVA-simultaneous component analysis), OPLS (orthogonal-PLS), OPLS-DA (OPLS-discriminant analysis), SIMCA (Soft independent modelling of class analogies), HCA (hierarchical cluster analysis), SOMs (self-organizing maps), SVM (Support vector machines) and Random Forest (Sugimoto et al. 2012; Bartel et al. 2013). Differential metabolic profiles between two groups can be compared using parametric Student t-tests and Wilcoxon rank sum nonparametric tests, while ANOVA test is applied for multiple groups (Bartel et al. 2013). In contrast, multivariate methods analyzed and compared between different metabolic features and interactions between them (Bartel et al. 2013; Alonso et al. 2015). These multivariate analyses can be classified into unsupervised (PCA, ASCA, HCA and SOMs) and supervised (PLS regression, OPLS, SIMCA and SVM) methods (Sugimoto et al. 2012; Bartel et al. 2013). Unsupervised methods analyze data irrespective to the type of study samples; whereas supervised methods categorize the study samples according to the phenotypes prior to analysis and more appropriate for constructing risk prediction models (Alonso et al. 2015).
Bioinformatic tools have contributed to metabolomics field by transforming the metabolomic raw data obtained into biologically meaningful information (Johnson et al. 2014). A number of bioinformatic databases are currently publicly available for metabolomic data analysis and visualization tools, including KEGG (http://www.genome.ad.jp/kegg/), BioCyc (http://biocyc.org/), DOME (http://medicago.vbi.vt.edu), MapMan (http://gabi.rzpd.de/projects/MapMan/), MetaCyc (http://metacyc.org/), MetNet (http://metnet.vrac.iastate.edu/) and KaPPA-View (http://kpv.kazusa.or.jp/kappa-view/) (Shulaev 2006; Johnson et al. 2014; Sas et al. 2015). Other bioinformatics tools for pathway mapping and network visualization include Paintomics, VANTED (Visualization and Analysis of Networks containing Experimental Data), and MetaboAnalyst. In order to build a network of genes-metabolites pathways interaction, several network tool such as MetScape, MBRole (Metabolites Biological Role), MSEA (Metabolite Set Enrichment Analysis), MetaMapp, 3Omics, ProMeTra and mummichog attempted to extend this approach to metabolite biomarkers discovery (Shulaev 2006; Johnson et al. 2014; Sas et al. 2015).
9 Metabolomic Biomarkers
As indicated in Table 1, a number of metabolites have been discovered in metabolomics studies associated with incident hypertension (Holmes et al. 2008; Liu et al. 2011; Zheng et al. 2013a, b; Zhong et al. 2014; Nikolic et al. 2015; van Deventer et al. 2013; Wang et al. 2015). These metabolites may serve as biomarkers for early diagnosis, prevention and treatment of this disease.
Table 1
Association of metabolic changes with incident hypertension
Metabolomic markers | Techniques employed | Statistical analysis | References | |
---|---|---|---|---|
Increased concentrations | Decreased concentrations | |||
Alanine | Formate, hippurate and N-methylnicotinate | 1H-NMR | OPLS-DA | Holmes et al. (2008) |
Choline | Urea, α-1 Acid glycoprotein | 1H-NMR | OPLS and PLS-DA | De Meyer et al. (2008) |
D-glucose, D-galactose, glucosamine, L-sorbose, sucrose, D-sorbitol, inosose, myo-inositol, heptanoic acid, 1-stearoylglycerol, oleic acid, 1-palmitoylglycerol, nonanoic acid, eicosanoic acid, hexanoic acid, pipecolic acid, L-ornithine, L-lysine, pyroglutamic acid, L-histidine, L-alanine, glutamine, L-isoleucine, α-aminoadipic acid, N-acetylglycine, L-tyrosine, homocysteine, L-aspartic acid, glutamic acid, L-tryptophan, allantoin, 3-amino-2-piperidone, urea and 2-ketoglutaric acid | D-Fructose, D-cellobiose, lactobionic acid, glycerol 3-phosphate | GC-TOF-MS | PCA, PLS and OPLS | Liu et al. (2011) |
4-Hydroxyhippurate, 5α-androstan-3β,17β-diol disulfate, androsterone sulfate and epiandrosterone sulfate | – | GC-MS and LC-MS | PCA | |
Very low density lipoprotein, low density lipoprotein, lactic acid, acetone and acetylformic acid | Valine, alanine, glucose, inose, p-hydroxyphenylalanine and methylhistidine | 1H-NMR | PLS-DA, OPLS-DA and t-test | Zhong et al. (2014) |
Oxalic acid, fumaric acid, glycerol, adenine, pyrophosphate and uric acid | L-Valine, L-isoleucine, glycine, L-threonine, L-methionine, ornithine, L-asparagine, L-glutamine, citrulline, L-lysine, L-tyrosine, L-tryptophan, L-cystine and capric acid | GC-MS | PCA, Mann-Whitney-Wilcoxon | Wang et al. (2015) |
3-OH-Sebacic acid, 2-OH-isovalerate, 4-OH-phenyl-lactate, tricarballylic acid and lactic acid | Hesperetin, hexenoylcarnitine, fumaric acid, methylguanosine, N-acetylarylamine, kynurenic acid, phenylglyoxylate, indole carboxylate glucuronide, methyluric acid, dimethyluracil and trimethyl-L-lysine | GC-MS and LC-MS | PCA | van Deventer et al. (2013) |
Talose, lyxose, glucose-1-phosphate, methylmalonic acid, malonic acid, shikimic acid | Threonine, nicotinoyl glycine, phenylalanine, aspartic acid, Gly-Pro, galactose, thymol, noradrenaline, methyl-beta-D-galactopyranoside, 2-methoxyestrone, alpha-tocopherol | GC-MS | t-test/Wilcoxon rank sum test | Hao et al. (2016) |
Carbohydrate metabolism alterations were found to be associated with hypertension, and patients with essential hypertension demonstrated impaired glucose tolerance, insulin resistance and abnormal glucose metabolism (García-Puig et al. 2006). In conjunction with the elevated level of glucose, altered levels of other monosaccharides, disaccharides and polysaccharides were observed in hypertensive patients. Of which, the levels of lactate, galactose, glucosamine, glycerol, 4-hydroxyphenyllactate, 2-hydroxyvaleric acid, 2-ketoglutaric acid, oxalic acid, sorbose, sucrose, sorbitol, inosose and myo-inositol were increased, whereas fructose, cellobiose, methyluric acid, lactobionic acid, indole carboxylic acid, tricarballylic acid formate and glucuronide were decreased (Liu et al. 2011; Zhong et al. 2014; van Deventer et al. 2013; Wang et al. 2015). These results suggest that carbohydrate metabolism dysregulation may play an important role in hypertension, through sodium retention, renal tubular sodium reabsorption, sympathetic nervous system and adverse effects of anti-hypertensive drugs (Gambardella et al. 1993; Sechi et al. 1997; Savica et al. 2010).
Several lines of evidence also indicated that free fatty acids were significantly associated with the development of hypertension (Fagot-Campagna et al. 1998; Wang et al. 2008), possibly by regulating vascular tone and microvascular function, inhibiting endothelium-dependent vasodilatation and increasing blood pressure (de Jongh et al. 2004; Spijkers et al. 2011). Furthermore, increased levels of free fatty acids may contributed to hypertension through the alteration of membrane microviscosity and lipid metabolism, by modulating ion transport, pH regulation, intracellular Ca2+ handling and signaling pathway (Zicha et al. 1999). Interestingly, findings from metabolomic studies indicated that the levels of very low-density lipoprotein, low-density lipoprotein, acetone, 1-stearoylglycerol, 1-palmitoylglycerol, and free fatty acids such as oleic acid, nonanoic acid, ecosanoic acid, hexanoic acid, and heptanoic acid were significantly higher among hypertensive patients compared to normotensive controls (Liu et al. 2011; Zhong et al. 2014).
Another metabolic pathway that is repeatedly found to be perturbed in hypertensive patients is amino acid metabolism. Amino acids, including alanine, histidine, isoleucine, lysine, homocysteine, ornithine, tyrosine, p-hydroxyphenylalanine, methylhistidine, aspartic acid, glutamine, glutamic acid and pyroglutamic acid were observed at higher concentrations in blood samples of patients with hypertension (Liu et al. 2011; Zhong et al. 2014). However, isoleucine, glycine, threonine, phynylalanine, methionine, ornithine, asparagine, glutamine, citrulline, lysine, tyrosine, tryptophan, cystin, hexenoylcarnitine and trimethyl-L-lysine were found to have lower concentrations in other metabolomic studies (van Deventer et al. 2013; Wang et al. 2015). Alanine is found in dietary sources of proteins, but is particularly concentrated in meats. Alanine was positively associated with high blood pressure, which in agreement with several metabolomic studies (Holmes et al. 2008; Liu et al. 2011; Zhong et al. 2014). In addition, other amino acids, branched chain amino acids and sex steroid metabolites such as 5α-androstan-3β,17β-diol sulfate, androsterone sulfate and epiandrosterone sulfate, were independently associated with increased risk of hypertension (Zheng et al. 2013a, b).
Urea, the main metabolite of protein metabolism, has become an independent predictor of hypertension (De Meyer et al. 2008; Liu et al. 2011). Likewise, uric acid and allantoin were observed at higher levels in hypertensive patients, in contrast to dimethyluracil and methyluric acid (Liu et al. 2011; van Deventer et al. 2013; Wang et al. 2015). The underlying mechanism could be explained by the regulation of urinary sodium (Na) homeostasis via an effect of diuresis and natriuresis (Cirillo et al. 2002). In hypertensive patients, urinary urea was inversely associated with blood pressure, as the higher urea level may reduce glomerular filtration rate and impaired renal function (Cirillo et al. 2002; Martin et al. 2005).
Researchers also highlighted changes in metabolites originated from gut microorganism, indicating a possible link between gut microflora activities with hypertension. Notably, microbial metabolites (such as formate, hippurate, 4-hydroxyhippurate and lyxose) detectable blood and urine in humans were found significantly changed and can be associated with to the development of hypertension (Hao et al. 2016; Holmes et al. 2008; Zheng et al. 2013a, b). Together, these finding shows a promising future research direction for hypertension.
10 Lipidomic Biomarkers
The number of lipidomic studies related to hypertension is limited (Graessler et al. 2009; Hu et al. 2011; Kulkarni et al. 2013). As shown in Table 2, the identified lipid metabolites were ether phosphatidylcholine, ether phosphatidylethanolamine, lysophosphatidylcholine, phosphatidylcholine, sphingomyelin, cholesteryl ester, triacylglycerol, monohexosylceramide, phosphatidylinositol and diacylglycerol, which mainly involved in lipid signaling pathway. Dysregulation of this signaling pathway may contribute to the pathogenesis of human diseases such as inflammation, cancer and metabolic disease (Wymann and Schneiter 2008). For instance, sphingomyelins consisted of phosphocholine or phosphoethanolamine as their phospholipid polar head group and served as substrates for the hydrolysis of ceramides through action of sphingomyelinases (Hannun and Obeid 2008). Sphingomyelinases enzymes further break down sphingomyelin to generate ceramide and phosphocholine, which may, in turn, accelerate the formation of diacylglycerol from phosphocholine (Hannun and Obeid 2008). Therefore, diacylglycerol (final product of lipid signaling pathway), together with the second messenger inositol-1,4,5-triphosphate, play important roles in regulating protein kinase C activity and calcium release (Hannun and Obeid 2008). Moreover, it has been reported that the accumulation of triacylglycerol lipid species may induce lipotoxicity in hypertension (Hu et al. 2010). Therefore, there is a strong biological plausibility for the role of lipid signaling molecules in pathophysiology of hypertension (Kulkarni et al. 2013).
Table 2
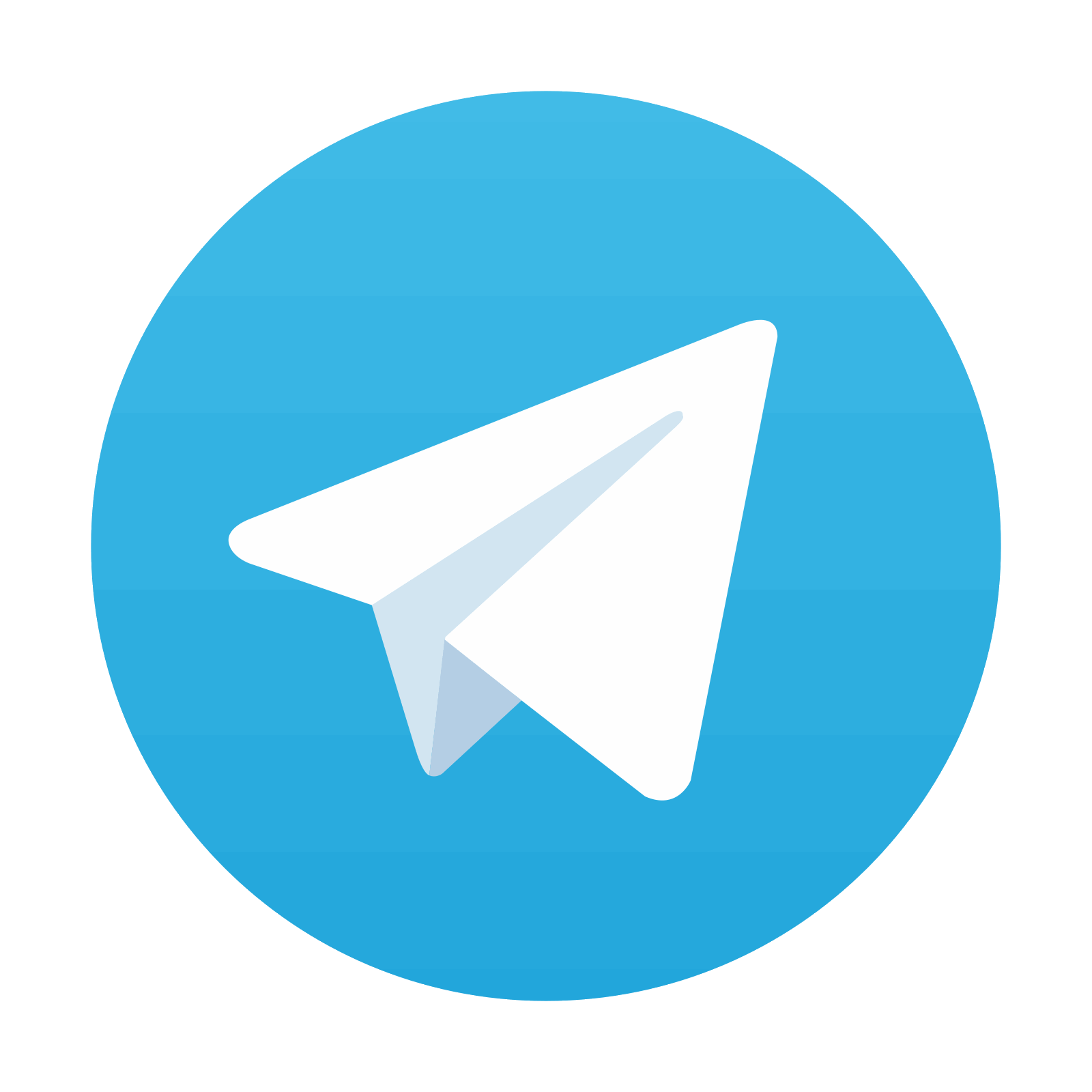
Association of lipid metabolites with incident hypertension
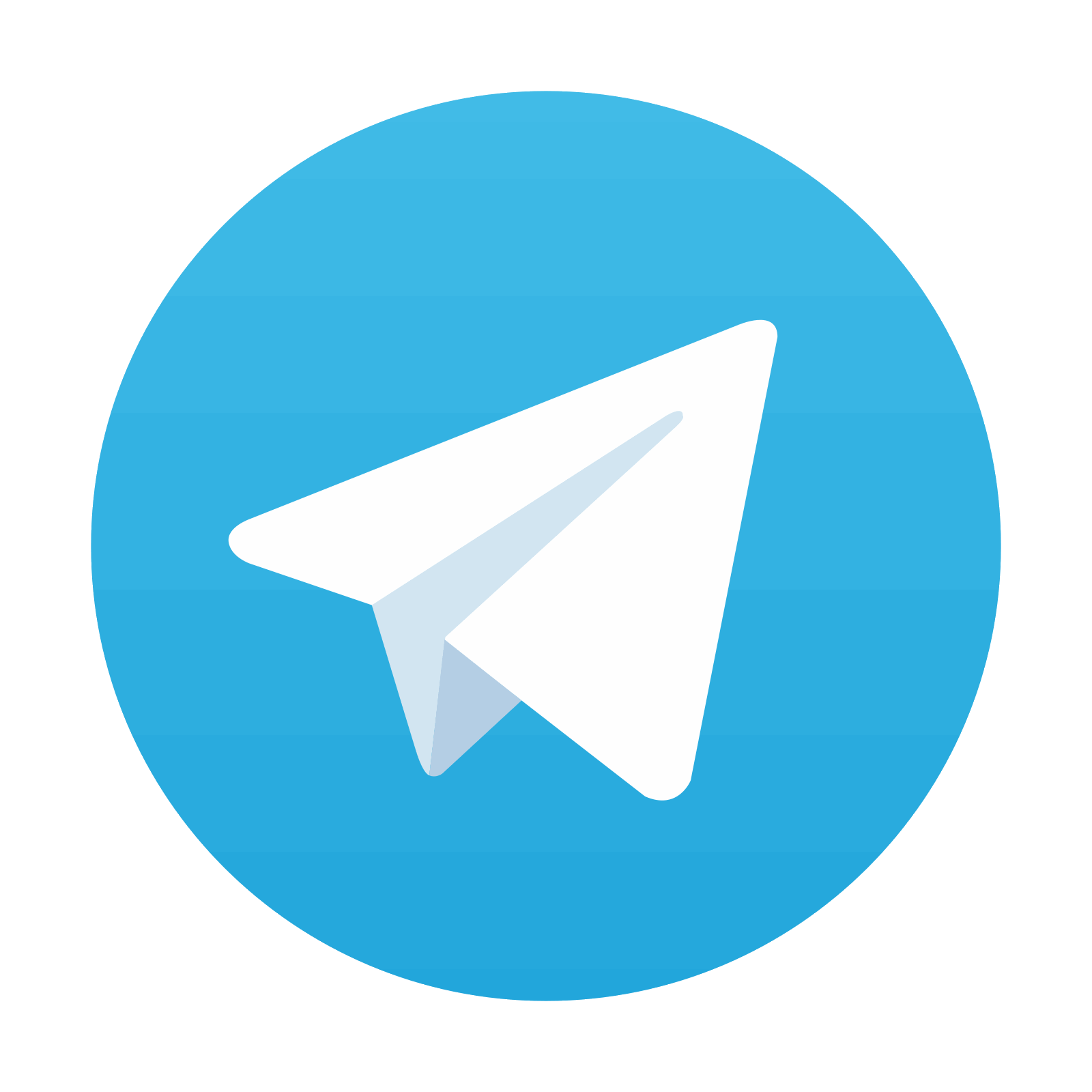
Stay updated, free articles. Join our Telegram channel

Full access? Get Clinical Tree
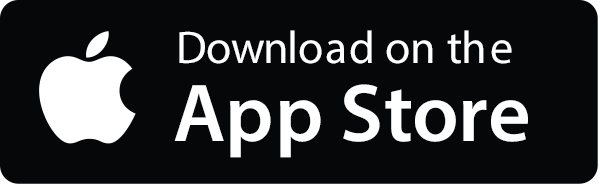

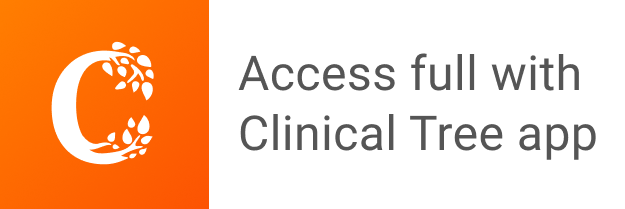