Cancer type
Cellular target
Targeted agent
Class of agent
KRAS
Cetuximab
Monoclonal antibody against EGFR
HER2
Trastuzumab
Monoclonal antibody HER2/Neu (EGFR2)
BCR-ABL fusion protein
Imatinib
Receptor tyrosine kinase inhibitor
c-KIT
Imatinib
Receptor tyrosine kinase inhibitor
EGFR
Erlotinib and gefitinib
Receptor tyrosine kinase inhibitor
EML4-ALK fusion protein
Crizotinib
Receptor tyrosine kinase inhibitor
BRAF V600E
Vemurafenib
B-faf/MEK/ERK pathway inhibitor
BRCAI, BRCA2
Olaparib
Poly(ADP-ribose) polymerase (PARP) inhibitor)
Another example is HER2/neu gene amplification which leads to overexpression of its receptor on the cell membrane in approximately 30 % of human breast tumours. This has been shown to be is related to a worse prognosis in patients with node-positive breast cancer due to increased proliferation and angiogenesis and inhibition of apoptosis [27–30]. HER2/neu is also the target for the monoclonal antibody trastuzumab from which patients with HER2/neu overexpressing tumours benefit in a metastatic and adjuvant setting [45–47].
Non-cancer Paradigms
In cardiovascular disease things are not yet at the same stage of advancement as is seen in cancer therapy, but an important change has taken place all the same. In March 2014, the Joint British Societies (JBS) 3rd Report on CVD (JBS3) was launched [48]. The major change in JBS3 from JBS2 is the recommendation of an approach to risk estimation and management, based not only on traditional models of short-term (10-year) risk, but also on individual lifetime CVD risk. Short-term absolute risk estimates are heavily dependent on age and gender so that decisions based on this approach have resulted in drug prescription to a very large number of older individuals. Younger subjects and women have tended to be excluded even if they have substantially elevated modifiable risk factors and are consequently at high lifetime risk. Evidence has accumulated that long-term exposure to CVD risk factors actively drives atherogenesis and that early treatment can modify disease evolution and risk of future CVD events. This represents a real opportunity for ‘investment’ in future cardiovascular health rather than waiting for people to be unwell, or to get close to their “illness threshold”, before intervening. Of course, for both cardiovascular disease, and for cancer, the essential verities of a healthy lifestyle (avoiding excess weight and smoking, restricting sugar, salt, and fat intake, and taking regular exercise) all apply from the moment of birth onwards.
So the new JBS3 CVD risk calculator has been designed to identify the sizeable number of individuals in the population who are at low short-term risk but at high life-time risk [48]. Novel metrics such as ‘heart age’ and CVD event free survival are displayed together with 10-year risk. The calculator is designed to communicate the long-term consequences of an individual’s lifestyle and associated risk factors and the substantial lowering of CVD risk which can be obtained by early lifestyle changes and, where appropriate, by use of evidence based drug therapies. The JBS3 risk calculator should empower individuals to understand why they should start CVD risk reduction, when they should start, and what they should do. It also emphasises the need for long-term maintenance of risk factor lowering to have the greatest influence on CVD risk over lifetime. It will also help clinicians to engage in this important dialogue with their patients. It is thus an “appeal” to the individual, whereas previous health scores were more a means better to marshal scarce healthcare resources.
In terms of other chronic conditions – rheumatoid arthritis, psoriasis, solid organ transplant rejection, inflammatory bowel disease, dementia – in all of these cases there are (or soon will be) panels of biomarkers to predict future susceptibility, and, expensive new biologic agents which claim to be mechanistically-targeted interventions. Dementia in particular is a massive challenge for societies, and so recent advances in science-based predictive imaging [49], in biomarkers of susceptibility [50], and in potential novel therapeutic interventions [51], are very exciting, even at a very early stage. Segmenting the use of these new and inevitably expensive therapies to susceptible/responsive patient cohorts will be the key both to their wise application and affordability. In other words, sparing people who either will not develop the condition, or who will not respond adequately to the treatment, from the toxicity of an inappropriate therapy (the flip-side of choosing a responsive cohort) are just as serious potential gains which should accrue from the new ways of working.
Why Has Personalised, Predictive Medicine Now Become the “Flavour of the Month” in Countries with Already Super-Epensive Healthcare?
The wide-ranging impacts and myriad opportunities provided by personalised medicine can be summarized in reference to its four major attributes – which conveniently enough, all start with the letter “P” [9].
P Is for Personalised
Personalised medicine integrates personal genetic or protein profiles to strengthen healthcare at a more personalised level, particularly with the aid of recently emerging “-omic” technologies such as nutritional genomics, pharmacogenomics, proteomics, and metabolomics [52]. Personalised medicine targets what has a positive effect on a patient’s disease and then develops safe and effective treatments for that specific disease [9]. In fact, genetic biomarkers that may be specifically associated with a disease state are the foundation and cornerstone of personalised medicine. Knowledge of a patient’s genetic profile leads to the proper medication or therapy so that physicians can manage a patient’s disease or predisposition towards it using the proper dose or treatment regimen [10].
P Is for Predictive
Personalised medicine enables physicians to select optimal therapies and avoid adverse drug reactions. Molecular diagnostic devices using predictive biomarkers provide valuable information regarding genetically defined subgroups of patients who would benefit from a specific therapy. These complex diagnostic tests can be used to classify patients into subgroups to inform physicians whether patients would be treated successfully with hormone therapy alone or may require more aggressive chemotherapy treatment.
P Is for Preventative
Personalised medicine pursues not reaction but reaction. With the ability to forecast disease risk or presence before clinical symptoms appear, personalized medicine offers the opportunity to act on the disease through early intervention. In lieu of reacting to advanced stages of a disease, preventive intervention can be life-saving in many cases. For example, females with genetic mutations in the BRCA1 or BRCA2 genes have a higher chance of developing breast cancer compared to those in the general female population [53–55].
P Is for Participatory
Personalised medicine might and indeed should lead to an increase in patient adherence to treatment. When personalised healthcare assures its effectiveness and can minimize adverse treatment effects sparing the expenses, it can be expected that patients will be more likely and willing to comply with their treatments. Of course, to “qualify” for entry into the new treatment club, one first has to agree freely to share all relevant information from personal genetic and other analyses.
And P Is Also for Perhaps?
Perhaps we can find reliable predictive biomarker and genetic panels to tell us susceptibility and response? Perhaps the information from genome-wide screening, and individual genomic analysis, will provide us with novel mechanistic targets for interventions? But perhaps also this is an over-hyped paradigm, derived from enthusiastic extrapolation from a small sub-set of oncological conditions to the whole of medicine? Will society accept this “new deal”? We shall all find out ‘ere long’.
Statistical and Logistical Challenges Which Still Need to be Overcome
The critical component to success in personalised medicine is the discovery of gene signatures that drive individual variability in clinical outcomes or drug responses. Without these, there is unmanageable uncertainty. A number of systematic approaches have been proposed to identify “molecular fingerprints” that are predictive of patient prognosis and response to cancer treatments. In the data-driven approach, biomarkers associated with tumour characteristics are objectively searched in genome-wide analysis using data-mining tools. Unbiased biomarker discovery is the merit of this approach. A downside is that gene signatures identified by the data-driven approach are often difficult to interpret due to limited knowledge about their biological functions. In contrast, the knowledge-driven approach attempts to select candidate genes using prior knowledge or surveying the literature for evidence of linkage to either cancer pathological processes or pathways important in drug responses. As such, genes that are unknown to be involved in a process cannot be included.
Biomarker discovery begins by collecting molecular data in a drug response experiment. A large amount of genomic or genetic characteristics on cell-lines are experimentally determined using high-throughput technologies. The drug’s patterns of activity in cells are measured on a continuous (percent of cell survival or death) or discrete scale (responsive or resistant).
After narrowing down candidate genes to a few hundred, a statistical classification modelling technique is then used to construct a multivariate prediction model. Single biomarkers are less likely to furnish sufficient sensitivity and specificity for most applications. Several classification methods have been utilised, including a variant of linear discriminant analysis [56], support vector machines [57–59], Bayesian regression [60], partial least squares [61], principal component regression [62], and between-group analysis [63].
The ultimate evidence of the usefulness of a prediction model in a clinical setting remains randomised, prospective validation in clinical trials [64]. This is absolutely pivotal as it would underpin with robust evidence the assertion that this approach was of true benefit both to individuals and to wider society.
Several key challenges must be overcome before this tsunami of personal profile data can be successfully translated into clinically relevant opportunities for patients. Improved knowledge obtained using advanced profile technologies will not be sufficient for this purpose, but all stakeholders involved in personalised medicine will need to work together to take responsibility. Regulatory authorities will need to provide clear guidelines for evaluating and approving newly developed “personalised” drugs and should independently and rigorously validate the capabilities of the diagnostic devices that predict patient prognoses or drug responses. Medical educational institutions should prepare the next generation of physicians to use and interpret personal genetic information appropriately and responsibly. Public and private insurers need to evaluate the clinical and economic utility of “personalised” drugs and devices to facilitate reimbursement.
As an example of the new challenges facing this field, take the colossal task of storage, retrieval and expeditious analysis of the massive amount of data we shall need to acquire to achieve these goals. A whole new way of archiving and working using massive super-computer arrays has been developed [65]. In the era of “big data”, biomedical databases are brimming with protein structures, image collections and genomic sequences. As the data mount, new ‘cave automatic virtual environments’, or CAVEs, are being built to help researchers pick through the files. One such is the largest currently in the world, CAVE2 at Australia’s Monash University in Melbourne, Australia. What appears to be an enormous electronic billboard encircles the space, forming a cylindrical room with a 24-ft diameter. The images displayed on the 80, high-definition, liquid-crystal display panels beam out at a staggering 84-megapixel resolution. And with a pair of stereo glasses, they emerge out of the eight-foot-high display wall in three dimensions. Science fiction? No, science fact.
CAVE2 is the state of the art in electronic engineering and computer visualization technologies—and Monash’s CAVE2, which opened its doors only late last year (2013), is one of just two such facilities in the world. The first, slightly smaller CAVE2, with 72 panels instead of 80, started accepting scientists at the University of Illinois at Chicago (UIC) in October 2012. The circular CAVE2 design of both facilities is the latest immersive virtual environment to emerge from UIC’s Electronic Visualization Laboratory (EVL) – see [65].
Conclusions: “Back to the Future” – Is Personalised Medicine Coming Soon to a Hospital Near You?
Personalised medicine has received and continues to attract a growing amount of attention for its tremendous potential opening up a myriad new opportunities. The ultimate promise of personalised medicine depends on the discovery of the personal genetic causes of many common diseases. The remarkable advent of current high-throughput technologies in combination with improved knowledge of the molecular basis of malignancy, provides a solid base for identifying novel molecular targets driving drug-discovery programmes. The use of high-throughput technologies is expected to increase massively in the next few years driven largely by the fact that the cost of technologies will continue to drop sharply. Genomic sequencing and its interpretation will have to be further developed and standardised for routine clinical practice to develop efficient and effective methods for discovering and verifying new biomarkers and enabling personalized medicine technologies. In particular, efforts to standardise existing technologies will need to lead to more reproducible and robust identification of biomarkers. This revolutionised paradigm in healthcare is already beginning significantly to affect both research and clinical practice. And what has already started to happen in oncology will surely soon start to happen in other disease areas?
So, personalised healthcare — or what Professor Donna Dickenson calls “me” Medicine [66] — coupled to increasing patient “choice” through information emancipation – is now starting radically to challenge and then to transform our longstanding “one-size-fits-all” system model. Technologies such as direct-to-consumer genetic testing, pharmaco-genetically developed therapies in cancer care, private umbilical cord blood banking, and neurocognitive enhancement, all claim to cater to an individual’s specific biological character, and, in some cases, these technologies have shown significant potential. Yet in other areas they have produced negligible, or even negative, results to date [66]. Whatever is behind the rise of “me” Medicine, it doesn’t seem to be the quality of the science which is driving it alone – there is also a healthy dose of “economics” as well. So if it is indeed the case that “me” Medicine is now about rapidly to edge out “we” Medicine, will our commitment to our collective health have suffered as a result? Increasingly knowledgeable patients, prepared to use and share more of their own private data, are directly investing in their own health – this is a challenge in particular to systems which can be characterised as publically-funded, centralised, socialised healthcare.
Well before this amazing “Brave New World” healthcare paradigm has been shown to be both effective, and cost-effective, it is now being vigorously adopted. This is because it is a “Big New Idea”. The new concepts are taking physical form – in several places in the United Kingdom there have sprung up new “ivory-tower institutes”, often with titles as grandiose and otiose as their porticos and atria, emphasising (amongst other things) their scale, ambition and reach. As an example, it was announced by the United Kingdom’s Business Secretary Dr Vince Cable in August 2013 that a “Diagnostics for Stratified Medicines Catapult” would be established, joining several other Catapults, or “technological accelerators” [67]. A number of partners (including the Technology Strategy Board and the Medical Research Council) have committed to spend over £200 m over a 5 year period to promote the development of stratified medicine, providing the tools, processes and systems for identifying the right therapy for the right patient, at the right time and at the right dose. Presumably also from the right doctors in the right hospitals?
< div class='tao-gold-member'>
Only gold members can continue reading. Log In or Register a > to continue
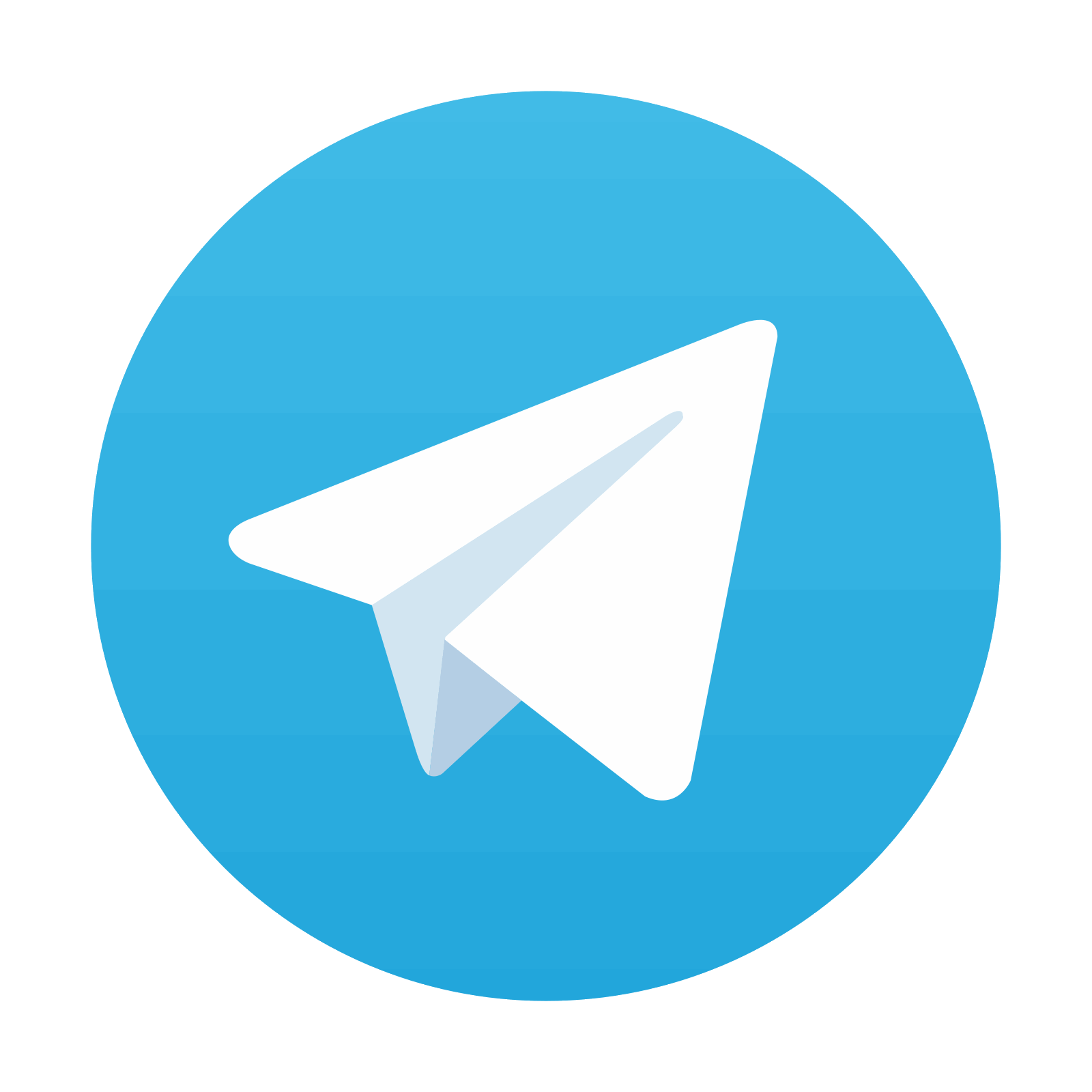
Stay updated, free articles. Join our Telegram channel

Full access? Get Clinical Tree
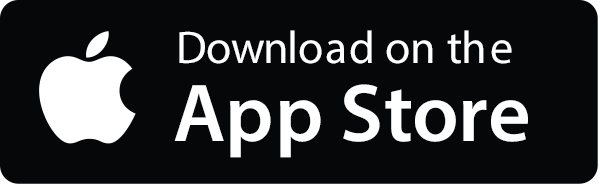
