Over nearly a decade, we have made substantial progress in predicting, understanding, and preventing hospital readmission following percutaneous coronary intervention (PCI). In 2007, the Medicare Payment Advisory Commission (MedPAC) noted that 30 day hospital readmission after PCI costs $359 million for Medicare . PCI readmission rates do seem to the setting in which PCI care is delivered, suggesting a relationship to quality of care. For example, the PCI readmission rate in New York is roughly triple the rate in northwestern Italy . Individual hospitals within the same region also feature wide variance in readmission rates even after adjustment for case mix . Furthermore, nearly half of PCI readmissions appear preventable .
In principle, therefore, reducing readmission after PCI might both improve quality and reduce unnecessary costs. To that end, the Centers for Medicare and Medicaid Services (CMS) and the National Cardiovascular Data Registry (NCDR) publically reported risk-adjusted 30-day readmission rates after percutaneous coronary intervention (PCI) as a pilot project. When the Hospital Readmission Reduction Program (HRRP) was enacted into law in 2010, penalties were imposed on hospitals for excess risk-standardized readmission rates for specific conditions . Although PCI has not been included among those penalizable conditions yet, the HRRP has been associated with decreasing readmission rates, even for non-penalty conditions . Furthermore, substantial overlap exists between PCI and acute myocardial infarction (AMI), which is included in the HRRP financial penalty.
1
Progress with risk prediction
The conceptual first step in preventing these readmissions is predicting them. Prediction can focus the attention of clinicians on PCI readmission, and target clinical resources to patients who are most in need of support. Models to predict hospital readmission after PCI are not new . Previous models have been based on clinical registry data alone, which offer more granular information than administrative data. However, potentially even more effective than clinical registry data alone would be adding clinical registry data to data about a patient’s resource utilization. In particular, variables such as subjective description of patient anxiety and previous emergency department utilization have been associated with readmission over and above models developed with clinical registry data . Instead of clinical registries, a more likely source of that information would be local electronic health records (EHRs).
A step toward integrating local EHR data with clinical registry data for PCI readmission prediction has now come from these models presented by Fanari et al. The Fanari et al. models were derived from a relatively small population in a single medical center, and as the authors acknowledge, may have weaker external validity than other models. However, this new model is notable conceptually because it integrates registry variables reported to the NCDR with local data from the Christiana Care health system. Access to these data about utilization may require studying a smaller study population, but makes substantial progress as a concept for risk prediction. In particular, the authors demonstrate a greater odds for 30 day readmission for patients who had previously been hospitalized during the previous 6 months. That type of information is more likely found in an EHR than a clinical registry. That integration is a key element of this new work.
2
Why risk prediction matters
Continuing to improve risk prediction for PCI readmission is critical, for two reasons. First, integrating novel variables into risk prediction for PCI readmission might generally inform how to extract data from the EHR to improve health care delivery for other procedures and disease processes as well. EHRs are now widely available across the United States. Part of the conceptual promise of EHRs is that data could be aggregated locally in ways that provide actionable information for improving the quality of care.
Second, risk prediction specifically for PCI is important because we have learned a lot over the past few years about PCI readmissions and how to prevent them. Therefore, better risk prediction for PCI readmission will help clinicians identify patients at highest risk, thus allowing better targeting of interventions to prevent readmission. For example, it is clear now that relatively few PCI readmissions are due to procedural complications such as stent thromboses and vascular access complications . Instead, the most common reason for readmission is chest discomfort without myocardial infarction .
The precise reason for so many patients readmitted for chest discomfort without myocardial infarction can be debated. On one hand, since these readmissions are associated with anxiety and previous health care utilization , better patient education, reassurance, and access to outpatient providers may help reduce readmission rates in ways that improve the patient experience for post-PCI patients. Alternatively, improvements in procedural techniques may reduce angina post-PCI. Given the high proportion of patients who receive repeat nuclear stress testing and repeat angiography without revascularization , the hypothesis that treatment of angina is inadequate is less likely. Rather, the essential element in reducing PCI readmissions is more likely better education, reassurance, and care coordination for these patients.
We are making meaningful progress. Local quality improvement efforts have reduced PCI readmissions . Perhaps in that context, PCI readmissions have declined nationally from 16.1% in 2000 to 15.4% in 2012 . Even though PCI was not explicitly included in the HRRP, the PCI readmission rate nationally fell about 10 times faster after the law was passed . Since the complexity of PCI patients has increased over that time period, the likelihood of 30 day readmission for a given patient declined by 33% between 2000 and 2012 .
These declines likely have benefitted many individual patients. Since PCI readmission is so common and so costly, these declines have likely also saved substantial health care costs.
2
Why risk prediction matters
Continuing to improve risk prediction for PCI readmission is critical, for two reasons. First, integrating novel variables into risk prediction for PCI readmission might generally inform how to extract data from the EHR to improve health care delivery for other procedures and disease processes as well. EHRs are now widely available across the United States. Part of the conceptual promise of EHRs is that data could be aggregated locally in ways that provide actionable information for improving the quality of care.
Second, risk prediction specifically for PCI is important because we have learned a lot over the past few years about PCI readmissions and how to prevent them. Therefore, better risk prediction for PCI readmission will help clinicians identify patients at highest risk, thus allowing better targeting of interventions to prevent readmission. For example, it is clear now that relatively few PCI readmissions are due to procedural complications such as stent thromboses and vascular access complications . Instead, the most common reason for readmission is chest discomfort without myocardial infarction .
The precise reason for so many patients readmitted for chest discomfort without myocardial infarction can be debated. On one hand, since these readmissions are associated with anxiety and previous health care utilization , better patient education, reassurance, and access to outpatient providers may help reduce readmission rates in ways that improve the patient experience for post-PCI patients. Alternatively, improvements in procedural techniques may reduce angina post-PCI. Given the high proportion of patients who receive repeat nuclear stress testing and repeat angiography without revascularization , the hypothesis that treatment of angina is inadequate is less likely. Rather, the essential element in reducing PCI readmissions is more likely better education, reassurance, and care coordination for these patients.
We are making meaningful progress. Local quality improvement efforts have reduced PCI readmissions . Perhaps in that context, PCI readmissions have declined nationally from 16.1% in 2000 to 15.4% in 2012 . Even though PCI was not explicitly included in the HRRP, the PCI readmission rate nationally fell about 10 times faster after the law was passed . Since the complexity of PCI patients has increased over that time period, the likelihood of 30 day readmission for a given patient declined by 33% between 2000 and 2012 .
These declines likely have benefitted many individual patients. Since PCI readmission is so common and so costly, these declines have likely also saved substantial health care costs.
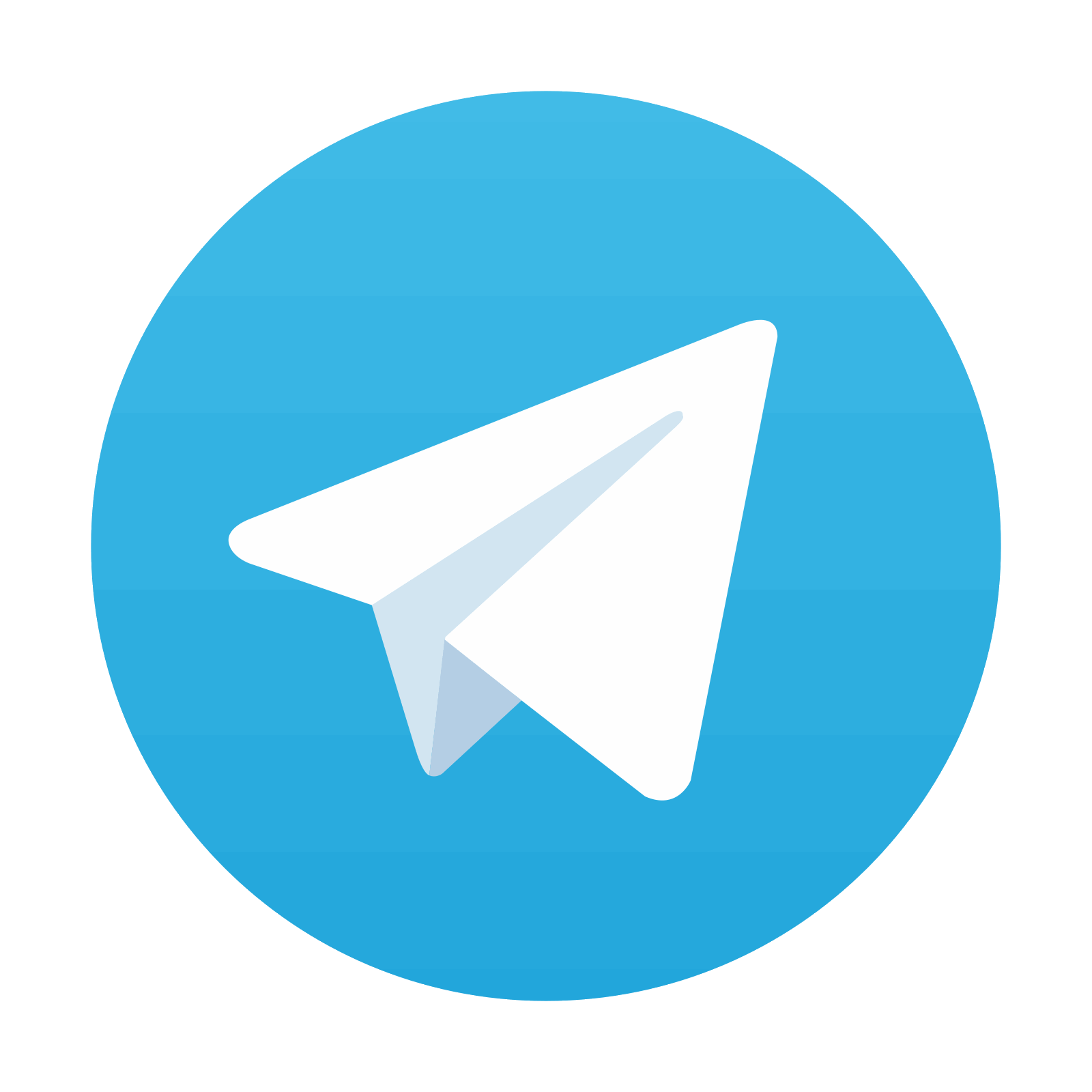
Stay updated, free articles. Join our Telegram channel

Full access? Get Clinical Tree
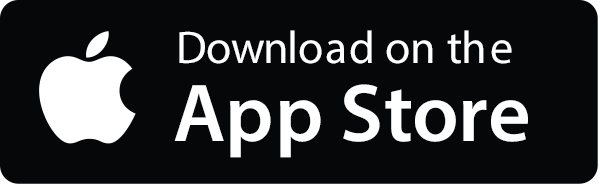
