Background
Previous single-center studies have shown that telemedicine improves care in newborns with suspected heart disease. The aim of this study was to test the hypothesis that telemedicine would shorten time to diagnosis, prevent unnecessary transports, reduce length of stay, and decrease exposure to invasive treatments.
Methods
Nine pediatric cardiology centers entered data prospectively on patients aged <6 weeks, matched by gestational age, weight, and diagnosis. Subjects born at hospitals with and without access to telemedicine constituted the study group and control groups, respectively. Data from patients with mild or no heart disease were analyzed.
Results
Data were obtained for 337 matched pairs with mild or no heart disease. Transport to a tertiary care center (4% [ n = 15] vs 10% [ n = 32], P = .01), mean time to diagnosis (100 vs 147 min, P < .001), mean length of stay (1.0 vs 26 days, P = .005) and length of intensive care unit stay (0.96 vs 2.5 days, P = .024) were significantly less in the telemedicine group. Telemedicine patients were significantly farther from tertiary care hospitals than control subjects. The use of inotropic support and indomethacin was significantly less in the telemedicine group. By multivariate analysis, telemedicine patients were less likely to be transported (odds ratio, 0.44; 95% confidence interval, 0.23–0.83) and less likely to be placed on inotropic support (odds ratio, 0.16; 95% confidence interval, 0.10–0.28).
Conclusions
Telemedicine shortened the time to diagnosis and significantly decreased the need for transport of infants with mild or no heart disease. The length of hospitalization and intensive care stay and use of indomethacin and inotropic support were less in telemedicine patients.
One percent of all children are born with congenital heart disease. Echocardiography is used for definitive diagnosis or exclusion of congenital heart disease in newborns, and the management of neonates with sepsis, pulmonary hypertension, and prematurity is also greatly enhanced by echocardiography. However, many neonates in rural areas or smaller cities do not have immediate access to local pediatric sonographers or echocardiographic interpretation by pediatric cardiologists. This can result in suboptimal echocardiogram quality, delay in the initiation of medical intervention, unnecessary patient transport, and increased medical expenditures.
Telemedicine has been used with increased frequency to improve the efficiency of pediatric cardiology care in hospitals not served by pediatric cardiologists. Several reports from single institutions suggest that telecardiology is accurate, improves patient care, is cost-effective, and enhances echocardiographic quality in locations not served by pediatric cardiologists. Telemedicine has also been shown to prevent unnecessary transport of neonates with suspect heart disease by ruling out congenital heart defects or diagnosing lesions without immediate hemodynamic consequences that require only basic medical intervention or outpatient follow-up.
We report the first multicenter (nine institutions) study assessing the impact of telemedicine on the delivery of care to infants undergoing echocardiography. Time to diagnosis, rates of transport, hospital length of stay (LOS), and exposure to invasive treatments were compared between telemedicine and control patients (matched by gestational age, weight, and diagnosis) without significant heart disease. We hypothesized that telemedicine would shorten time to diagnosis, prevent unnecessary transports, reduce LOS, and decrease exposure to invasive treatments.
Methods
Patients and Institutions
In this 36-month, prospective, multicenter cohort study (July 1, 1999, to December 31, 2001), all infants aged ≤ 6 weeks who were referred to one of the participating tertiary care institutions with the diagnosis of “heart murmur” or “rule out congenital heart disease” were enrolled by a pediatrician or neonatologist, who evaluated the patients and made all management decisions. Sonographers employed by community hospitals who scanned adult and pediatric patients performed all echocardiographic studies. Each participating tertiary care center interpreted echocardiograms obtained at delivery hospitals that had access to telemedicine and those that did not have access to telemedicine. Subjects born at hospitals with access to telemedicine constituted the study group, and those without telemedicine constituted the population from which the control group was drawn. Institutional review board approval was obtained at each participating institution (tertiary care and referral delivery hospitals).
A brief description of telemedicine co-investigators, facilities, technologies used, and number of transmissions per month per site is provided in Appendix A . In this study, no attempt was made to compare results by technology used. Each site co-investigator was responsible for study and control group patient identification and for data entry at that individual site.
Each participating tertiary care center provided both urgent and elective consultative services to both the telemedicine outreach sites and the control outreach sites. Participants fell into three general groups: (1) those needing immediate stabilization and referral for significant cardiac disease, (2) those who could be referred to an outpatient clinic at a later date for stable or asymptomatic cardiac disease (e.g., small ventricular septal defect, atrial septal defect, mild valvular stenosis), and (3) those with no heart disease. One control patient was matched to each study group patient within the referral population of each participating center by weight, gestational age, and diagnosis. For a large percentage of cases, it was not feasible to match on distance, and hence this aspect of matching was not successful in eliminating differences between groups on this variable. Every effort was made to ensure control group recruitment prospectively. However, in some cases, when the incidence of a specific diagnosis was very rare, a control patient was recruited retrospectively from infants referred in the 1-year time period before the start of the study. In addition to this stratified matching, we controlled for any residual influence of these variables by including weight and gestational age as covariates in all tests of between-subjects and within-subject effects.
Obtaining data from and matching control infants with mild or no heart disease, and matching them with infants in the telemedicine arm of the study, was more difficult compared with the infants with serious heart disease, who were always referred to the tertiary care institution for inpatient admission. We dealt with this problem by ensuring that co-investigators at each participating tertiary care center identified a collaborator at each of their referring institutions who worked with the co-investigator to facilitate data collection.
Participating physicians from nine institutions entered 1,057 patients into the database between September 1999 and December 2001. Of these, 581 (55.0%) received telemedicine services, and 476 (45.0%) were control patients. Control and experimental data were satisfactory from six of the nine institutions (see Appendix A ); the other three institutions did not enter control data, and hence their patients were not included in this analysis. For the remaining six sites, all telemedicine patients were matched with control subjects on diagnosis and disease severity. This yielded 37 matched telemedicine-control pairs with significant heart disease and 337 matched pairs with mild or no heart disease ( Table 1 ) from the six institutions with complete data. The length of time of telemedicine practice ranged from 3 to 8 years at these six hospitals. Because the nature of the questions we could address varied by severity of disease (e.g., the effects of telemedicine on avoidance of more intense and invasive treatment than necessary), we did not include the children with significant disease. Hence, the 337 matched pairs of infants with mild or no heart disease comprise the study sample for this report.
Diagnosis | n | % |
---|---|---|
Patent ductus arteriosus | 222 | 33 |
Ventricular septal defect (all types) | 132 | 20 |
Muscular | 96 | |
Membranous | 34 | |
Other | 2 | |
Atrial septal defect: secundum/patent foramen ovale | 120 | 18 |
No cardiac disease identified | 120 | 18 |
Pulmonary artery branch stenosis | 32 | 4.7 |
Pulmonary hypertension | 22 | 3.3 |
Atrioventricular septal defect | 16 | 2.4 |
Pulmonary valve stenosis | 6 | 0.9 |
Tricuspid valve regurgitation | 2 | 0.3 |
Right ventricular hypertrophy | 2 | 0.3 |
Data Collection
Data were collected at three separate time points for each study and control group patient. A single data entry Web site provided easy access for all participating centers to centralize electronic data entry. Data were entered for the study and control groups using questionnaires provided in the electronic database ( Appendices B–E ). Input from participating centers regarding refinement of these questionnaires was solicited at the beginning the study.
A master database received input from the participating centers, and aggregate data were provided to all principal and co-investigators every 3 months. In addition, each participating center received data specific to its own institution as requested. Aggregate data were presented at meetings of the investigators, which were held three times per year at national pediatric cardiology meetings (American Society of Echocardiography, American Heart Association, and American College of Cardiology). The data were masked to maintain confidentiality. A study code and key were maintained securely.
At the beginning of the study, each tertiary care center completed a detailed questionnaire ( Appendix B ) to ascertain the type of telemedicine technology at each site, the type of traditional cardiac care delivery, other demographic referral characteristics such as population served, and the volume of cardiac referrals at each participating tertiary care center. Data collected included patient identification number at both referring and tertiary care institutions, birth weight, gender, gestational age, and date of study entry.
Outcomes
The following outcomes were compared between telemedicine and control subjects: (1) fetal echocardiography diagnosis; (2) noncardiac diagnoses; (3) time from initial phone call requesting consultation to definitive diagnosis (interpretation of echocardiogram via telemedicine or videotape review from control sites); (4) transport to tertiary care center; (5) distance from tertiary care center; (6) total (combined for referral and tertiary care sties when applicable) LOS and intensive care unit (ICU) LOS (outliers were categorized as >60 days); and (7) adverse medical effects, including death, cardiac arrest, need for extracorporeal membrane oxygenation, intraventricular hemorrhage, mechanical ventilation, use of inotropic support, use of indomethacin, and use of prostaglandin E 1 .
Statistical Analysis
The database was developed and maintained to ensure completeness and accuracy, with range limits and item verification for each variable. It was backed up securely on a regular basis. Access to the database Web site was password controlled, and patient-identifying information was not shared among institutions.
Diagnostic accuracy was approached through the use of sensitivity-specificity analysis and measures of concordance (Cohen’s κ statistic) in the telemedicine subgroup. The original echocardiographic videotape was the standard for accuracy. Only a subset of patients underwent follow-up echocardiography on the basis of clinical indications. Two-by-two tables were constructed (presence or absence of telemedicine diagnosis by presence or absence of interpretation of echocardiogram) for a number of diagnostic questions, calculating sensitivity, specificity, and positive predictive value for this population. Kappa statistics were used to assess the degree of agreement.
Descriptive statistics were computed as a further check on data integrity and to characterize the sample and outcomes. Because the telemedicine and control samples were matched, we expected no differences between groups with regard to patient risk factors, and analysis of variance (ANOVA) comparing groups confirmed this. Some research questions addressed differences between groups (telemedicine vs nontelemedicine), whereas others involved predictive models. For example, we were interested in between-subjects differences in time to definitive diagnosis, as well as in the factors influencing time to diagnosis for each group. We used general linear regression analysis, including ANOVA and analysis of covariance. The former type of question (i.e., the mean differences between groups) was tested using the F statistic (i.e., ANOVA or analysis of covariance), whereas the latter type of question (i.e., percentage of variance explained by individual variables) was evaluated using ordinary least squares regression. We corrected for the effects of inflated experiment-wise error using the Holm method. Holm’s approach addressed the potential problem posed by multiple comparisons, while applying less stringent criteria to the establishment of α levels than is the case for the Bonferroni method.
To estimate sample size, a priori statistical power calculations were carried out under several different assumptions regarding the distribution of variables to be used in the analysis. Some analyses were conducted on the entire sample using ordinary least squares regression. Testing the statistical power of multiple regression for sample sizes of 150 through 250, we determined that, controlling for four variables, if a given independent variable accounts for 3% of the variance in the dependent variable out of a total explained variance of 25%, with statistical significance (α) of 0.05, then statistical power (1 − β) is approximately 0.80 for a sample of 195 subjects. To test between-groups hypotheses, statistical power was estimated for ANOVA, assuming a small to medium effect size (0.3) and α = 0.05. The calculations suggested that power of 0.80 could be anticipated using a sample of 45 subjects; a medium effect size (0.5) could be detected with the same α level and power using a sample of 25. Hence, a priori power calculations supported the adequacy of the sample size in this study.
Results
In unadjusted analyses, there were no significant between-groups differences in birth weight, gestational age, gender, and history of fetal echocardiography ( Table 2 ). Telemedicine patients were more likely to have respiratory disease ( P = .015) or heart disease associated with genetic syndromes ( P < .001). The prevalence of other noncardiac diagnoses was similar across groups. Four percent of telemedicine patients and 10% of control patients ( P < .01) required transport to other facilities ( Table 3 ). Time required for a definitive diagnosis ( Table 3 ) among telemedicine patients was significantly shorter ( P < .001) than time to diagnosis for control patients. Telemedicine patients were located significantly farther ( P < .01) from tertiary care hospitals than control patients ( Table 3 ). There were no statistically significant differences in the number of level 2 and level 3 nurseries between telemedicine and control hospitals. No diagnostic errors were reported in the telemedicine group (compared with subsequent videotape review).
Variable | Telemedicine | Control | P |
---|---|---|---|
Weight (kg) | 2.5 ± 1.2 (0.44–5.1) | 2.6 ± 1.2 (0.45–5.2) | NS |
Gestational age (wk) | 35 ± 5.5 (23–42) | 35 ± 5.6 (24–43) | NS |
Female/male | 180/157 | 160/177 | NS |
Fetal echocardiography | 5% (18) | 7% (24) | NS |
Noncardiac diagnoses | |||
Respiratory | 24% (64) | 15% (41) | .015 |
Genetic syndrome | 11% (29) | 2% (6) | <.001 |
Neurologic | 8% (21) | 8% (22) | NS |
Gastrointestinal | 3% (7) | 4% (11) | NS |
Genitourinary | 2% (5) | 3% (7) | NS |
Musculoskeletal | <1% (1) | 2% (6) | NS |
Variable | Telemedicine | Control | P |
---|---|---|---|
Transported to tertiary care hospital | 4.% (15) | 10 % (32) | .01 |
Distance from tertiary care hospital (mi) | 19 ± 15 (0–92) | 10 ± 15 (0–113) | <.001 |
Time to diagnosis (min) | 100 ± 67 (10–311) | 147 ± 60 (25–474) | <.001 |
Total LOS and ICU LOS were significantly shorter among the telemedicine patients than the control patients ( P = .005 and P = .024, respectively; Table 4 ). LOS in the pediatric ward and well nursery did not differ between groups. Differences in LOS and ICU LOS remained significant even after outliers (hospital stay >60 days) were excluded from the analysis ( P = .027 and P = .026, respectively). Cardiac issues were not responsible for prolonged LOS in any patient.
Telemedicine | Control | P | |
---|---|---|---|
Including outliers ∗ | |||
Total LOS (d) | 1.0 ± 6.8 (0–102) | 2.6 ± 11 (0–96) | .005 |
Length of ICU stay (d) | 0.96 ± 6.8 (0–102) | 2.5 ± 11 (0–96) | .024 |
Excluding outliers ∗ | |||
Total LOS (d) | 0.72 ± 4.1 (0–44) | 1.6 ± 6.4 (0–58) | .027 |
Length of ICU stay (d) | 0.65 ± 4.0 (0–44) | 1.6 ± 6.2 (0–58) | .026 |
The results pertaining to medical complications are shown in Table 5 . Controlling for birth weight and gestational age, control patients were significantly more likely to receive inotropic support ( P = .001) and indomethacin ( P = .026). There were no significant differences in the incidence of death, cardiac arrest, and intraventricular hemorrhage or in the use of prostaglandin infusion, mechanical ventilation, or extracorporeal membrane oxygenation.
Complication | Telemedicine | Control | P |
---|---|---|---|
Inotropic support | 8% (27) | 26% (88) | <.001 |
Indomethacin ∗ | 11% (29) | 18% (47) | .026 |
Prostaglandin E 1 | 0% (0) | <1% (1) | NS |
Mechanical ventilation | 28% (94) | 30% (101) | NS |
Extracorporeal membrane oxygenation | 1% (2) | <1% (1) | NS |
Intraventricular hemorrhage | 7% (24) | 5% (15) | NS |
Cardiac arrest | 2% (6) | 3% (11) | NS |
Death | 4% (13) | 4% (12) | NS |
∗ Indomethacin data were available for only 268 matched pairs.
Using multivariate logistic regression models ( Table 6 ) to predict the effect of telemedicine versus control group membership on outcomes, with birth weight and gestational age as covariates, telemedicine patients were less likely to be transported (odds ratio, 0.44; 95% confidence interval, 0.23–0.83) and less likely to be placed on inotropic support (odds ratio, 0.16; 95% confidence interval, 0.10–0.28). Logistic regression revealed no significant difference in the occurrence of death, cardiac arrest, placement on mechanical ventilation, cerebral hemorrhage, use of prostaglandin E 1 , placement on extracorporeal membrane oxygenation, and prior fetal echocardiography. Total and ICU LOS were significantly shorter in the telemedicine group ( P = .028 for total LOS and P = .026 for ICU LOS).
Dependent Variable | Odds ratio (telemedicine) | 95% confidence interval | P |
---|---|---|---|
Transported | 0.435 ∗ | 0.229–0.827 | .011 |
Inotropic support | 0.164 ∗ | 0.096–0.280 | .001 |
Cardiac arrest | 0.527 ∗ | 0.184–1.505 | NS |
Death | 0.922 ∗ | 0.398–2.136 | NS |
Intraventricular hemorrhage | 1.849 | 0.900–3.799 | NS |
Mechanical ventilation | 0.841 ∗ | 0.555–1.274 | NS |
Fetal echocardiography | 0.711 ∗ | 0.373–1.355 | NS |
Extracorporeal membrane oxygenation | 2.069 | 0.186–23.043 | NS |
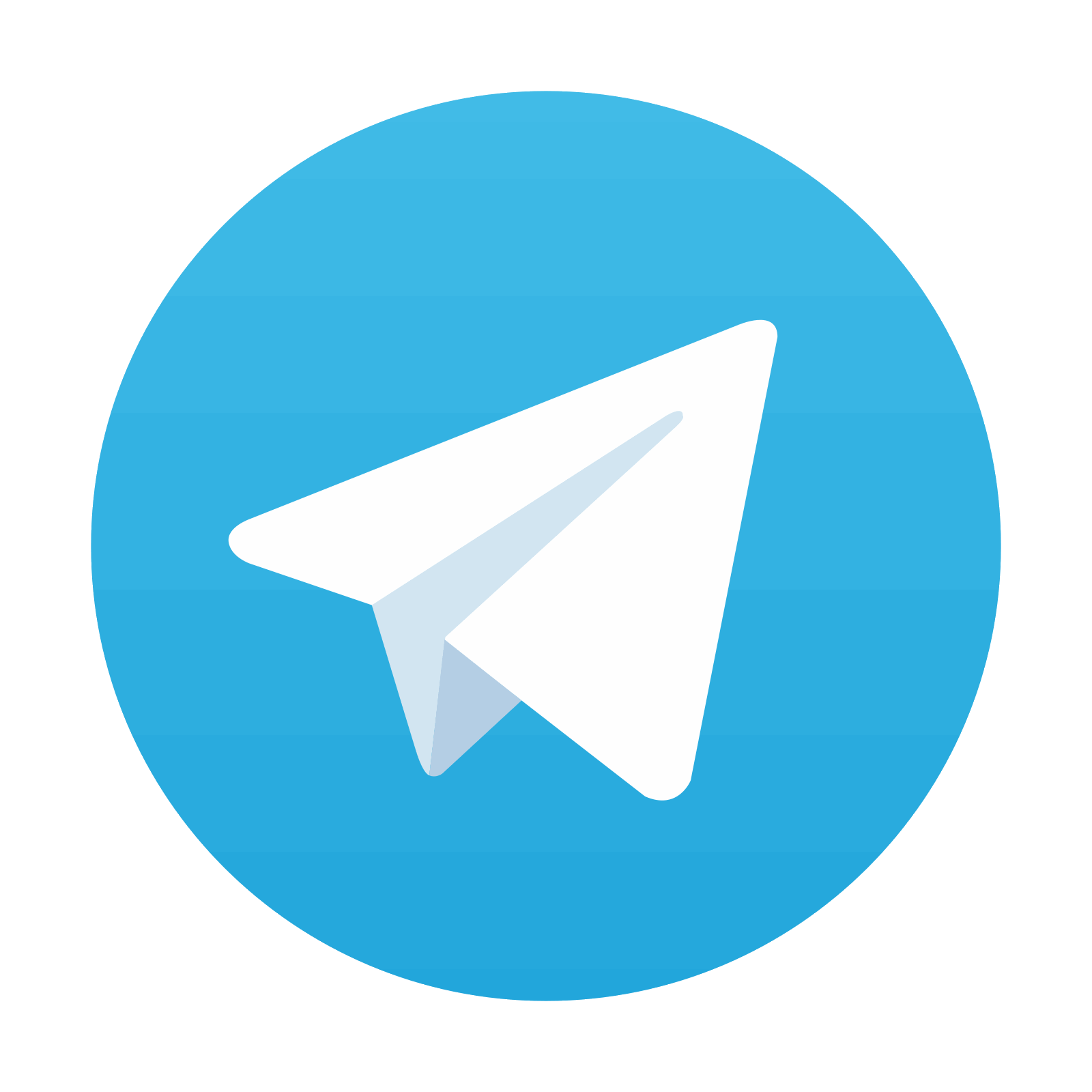
Stay updated, free articles. Join our Telegram channel

Full access? Get Clinical Tree
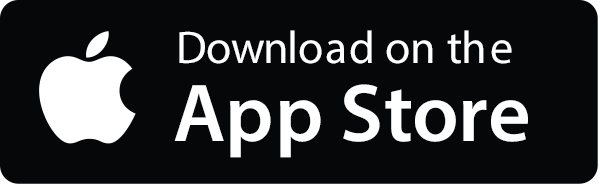

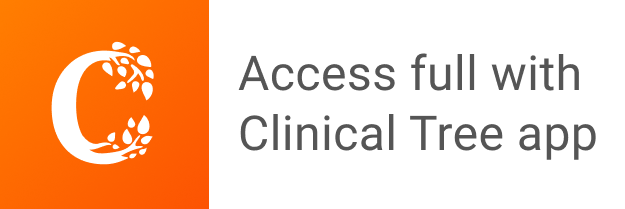