Abstract:
The results of the treatment of congenital heart disease are influenced by numerous factors, including the structure of the treatment center, the processes in place to administer treatment, and the unique patient- and disease-specific variables that distinguish each congenital heart lesion as unique. Numerous metrics derived from a variety of data sources are used to define outcomes. This chapter discusses how to evaluate and understand this information.
Key Words
Outcomes, Databases, STS Congenital Heart Surgery Database, Results, Mortality rates, Morbidity, Public Reporting
No concern is more important to parents than whether their child will be OK. For the team members who collaborate on the care of these children, “OK” can encompass many concerns, not simply “will the child survive surgery?” but “will he or she have a good quality of life?” and “what, if any, limitations might be expected?” These results may be measured as mortality, morbidity, length of stay, neurologic and functional outcomes, or quality of life, all of which are called outcomes . Our goal with this chapter is to simplify the complex science of the analysis of outcomes so that health care professionals can have a common language and shared concept of what is meant by the broad term outcome . Such understanding among providers will facilitate clear communication of accurate, relevant, and understandable information with each other and with the patient’s family.
Relationship Between Structure, Process, and Outcome
In 1966 Avedis Donabedian described three domains of quality in medicine: structure, process, and outcomes. Structure is the context in which care takes place, including the health care facilities, equipment, and personnel. Process is the action of taking care of the patients and can include protocols, as well as the organization of the delivery of health care. Outcomes are the results. Structure and process combine to influence outcome. If the outcomes being achieved are undesirable, modifiable structure and process measures should be investigated, for, as Paul Batalden stated, “Every system is perfectly designed to get the results it gets.” The variation seen in outcomes across hospitals may be due to (1) differences in patient populations, (2) disparate structure and process, and (3) random variation or chance. If all hospitals had identical structure and processes, outcomes across these hospitals would be more similar.
Quality measures for congenital and pediatric heart surgery have been defined and sorted according to Donabedian’s triad. Intriguingly, the balanced scorecard principle propagated by Kaplan and Norton (described in Chapter 2 ) identifies outcomes as the result of specific process drivers. Many business models now employ the balanced scorecard approach to managing process drivers as the preferred method of influencing outcomes, or, stated in reverse, when the outcomes are not the ones that are desired—such as a high mortality, increased length of stay, or neurologic morbidity—then “what processes [or structures] need to be altered to improve the ultimate outcomes?” Structural measures include participation in a national database, multidisciplinary rounds involving multiple members of the health care team, availability of a pediatric extracorporeal life support program, and surgical volumes. Process measures include the conduct of a multidisciplinary preoperative planning conference and a regularly scheduled quality assurance and improvement conference (also known as a mortality and morbidity or M and M conference), the interpretation of intraoperative transesophageal and epicardial echocardiography, the selection and time of administration of prophylactic antibiotics, and the use of preprocedural and postprocedural time-outs as well as handoff protocols. Outcomes measures include operative mortality and the occurrence of a variety of morbidities, including renal failure, neurologic deficits, arrhythmias, diaphragmatic paralysis, the need for postoperative mechanical circulatory support, and the need for unplanned reoperation or interventional catheterization. Although several of these structural and process measures have not been linked to outcomes by definitive research, they were chosen by experts as likely to be associated. Others are more complex than they may initially seem. For instance, the availability of intraoperative echocardiography is a structural measure; however, how well the team engages in a discussion of and responds to the echocardiographic findings in an operating room is part of the process of care. Multidisciplinary rounds, conferences, time-outs, and hand-offs present the same dilemma. The existence of these interactions could be considered a structural measure while the conduct of the meeting and the way in which information is exchanged are process measures. Part of the difficulty in selecting measures lies in the ability to describe them in ways that can be quantified.
Structural Measures
Volume is the most frequently cited structural measure associated with outcomes. Volume has been defined in numerous ways, including all surgical cases performed, cases using or not using cardiopulmonary bypass, major or “index” cases, and cases sorted by level of surgical complexity or expected mortality risk. The definition of volume chosen should depend on the relationship being investigated. For example, if one wishes to know if the number of Norwood procedures performed by an institution is associated with mortality after that operation, the volume of Norwood operations performed should be used. If one is interested in whether or not the entire surgical experience of a program is associated with mortality after the Norwood operation, then the total surgical volume should be used. Volume is influenced by a number of factors. Alterations in program structure and process measures may attract referrals as might changes in outcomes.
Other structural measures include the number of health care providers and their experience and the physical attributes of the hospital. Markers of professional standing such as membership in a professional organization like the Congenital Heart Surgeons’ Society may signal surgical expertise. More recently, the addition of specialty boards in congenital heart surgery (the Congenital Heart Surgery Certificate of the American Board of Thoracic Surgery) provides an additional structural element—either the surgeon is board certified as a congenital heart surgeon or not. The physical layout of centers varies. In some centers the operating room, intensive care unit, and cardiac catheterization laboratory are in relative proximity, which facilitates transfer of patients from one environment to another. In other institutions, movement of patients between these environments requires an elevator transport. Likewise, having a dedicated cardiac intensive care unit is a structural element. When studying the influence of such structural elements, extra attention should be paid to those structures that are modifiable. Some structures are fixed and inherent to an institution, and although they may influence care, they cannot be altered. Current work in business circles tries to identify “process drivers” to better control outcomes.
Process Measures
The process of care is critically important to the outcome. If a patient undergoes an operation in which technical errors are made, or if he or she is subjected to poor decision making related to the type or timing of surgical procedure or the conduct of perioperative care, they will be adversely affected. However, the associations between specific process measures related to congenital and pediatric cardiac surgery and subsequent outcomes have been poorly defined. This gap in knowledge may be for several reasons:
- •
First, it is difficult to distinguish between structural and process measures. For example, almost all programs now perform a preprocedural time-out and a handoff; however, the process of how that time-out or handoff is performed may vary between centers, including the actual safety for team members to “speak up” or the details of outlining the important specifics for (or of) the procedure to ensure that all equipment, supplies, personnel, and critical information are available. Furthermore, the relationship of this variation in the process to outcome may be impossible to determine because there are so many elements that factor into outcome. Likewise, most programs make multidisciplinary rounds, but the process for how these rounds are performed—how information is communicated or acted on—can be quite different between programs. It is very challenging to create a uniform process for some functions because there are so many important differences in people, structure, and even presentation of disease; nevertheless, variation in process may be critical in determining outcomes.
- •
Second, enthusiasm for process “mapping” has helped support the concept that process changes can result in improvement in outcomes. However, the uniqueness of our individual organizations and the wide variability in what processes need to be improved has led to considerable differences in process management across the spectrum of our varying structures. In other words, process management is far from “one size fits all.” It may suffice to say that the presence of some attentiveness to process improvement may be the distinguishing feature of how outcomes are influenced, rather than applying a specific process across the profession.
- •
Third, the associations between process measure and outcomes can be confounded by structural measures. High surgical volume, a structural measure, may be associated with both a process of care that yields excellent results and superior outcomes.
- •
Fourth, few process measures are included in large databases. This limitation of many multi-institutional databases is in part due to the challenge of defining such measures of process and the burden of capturing these data and is also likely due to the lack of evidence supporting the relationship of most process measures to outcome.
- •
Fifth, if the outcome of interest occurs infrequently, the number of patients or operations in the study cohort may not allow for the power to detect a statistically significant association.
- •
Sixth, each individual component of the process acts in concert with the others in the continuum of care.
Therefore the link between a single process measure and an outcome may be difficult to discern. Although process measures are critically important to outcomes, additional work needs to be done to link process measures to outcome in congenital and pediatric cardiac surgery.
Outcome Measures
The outcome most commonly considered is mortality. First, it is a critically important outcome. Second, it is binary and relatively easy to measure. Third, mortality rates have been high for certain congenital cardiac operations, making it a variable that is not rare in appearance. Fourth, mortality as a discrete point of data is relatively easy to collect. Yet, even this seemingly straightforward concept is complex. The definition of mortality has varied over time. Definitions have included any death after surgery before discharge, any death within 30 days of surgery, death that can be definitively linked to the operation, and all deaths regardless of cause. Operative mortality is defined in all Society of Thoracic Surgeons (STS) databases as (1) all deaths, regardless of cause, occurring during the hospitalization in which the operation was performed, even if after 30 days (including patients transferred to other acute care facilities); and (2) all deaths, regardless of cause, occurring after discharge from the hospital but before the end of the 30th postoperative day .
Given that the current mortality rate for all patients undergoing congenital cardiac surgery operations in the United States is under 4%, outcomes other than mortality are important to over 96% of patients. Perioperative morbidity is a group of outcome measures frequently collected in addition to mortality. As with mortality, consistency of definition is critical. In addition, the data source may influence the apparent outcome by how complete or accurate the desired information is. For example, administrative data often does not differentiate between preexisting conditions and postoperative occurrences. As a result preexisting conditions may be linked to an operation as morbidities when in fact they were not.
Long-term functional and neurologic outcomes are increasingly important as more and more children who undergo cardiac surgery survive to adulthood. Yet these outcomes are infrequently collected due to cost and the logistical challenges. Several national quality collaboratives, including the National Pediatric Cardiology Quality Improvement Collaborative (NPC-QIC) and the Cardiac Neurodevelopmental Outcome Collaborative (CNOC), are now dedicated to the multi-institutional investigation of functional and neurodevelopmental outcomes among neonates and infants with congenital heart disease. These groups have also implemented cooperative practices among centers and practitioners, designed to improve care based on best practices.
Case-Mix Adjustment
Variation in medical and surgical outcomes may result from differences in severity of disease, differences in the effectiveness of treatment, or random chance. Risk adjustment is necessary to account for differences in severity of disease, and data should be presented with confidence intervals to account for random chance. Adjusting for case mix in congenital and pediatric cardiac surgery is challenging because of the large numbers of diagnoses and procedures, as well as the large variety of potential risk factors, including chromosomal abnormalities, syndromes, noncardiac congenital anatomic abnormalities, and preoperative factors such as preoperative mechanical circulatory support, shock, preoperative mechanical ventilation, preoperative renal dysfunction, and preoperative neurologic deficit.
Risk Stratification
In pediatric and congenital cardiac surgery, risk stratification is a commonly used methodology to adjust for case mix. Risk stratification is a method of analysis in which the data are divided into relatively homogeneous groups (called strata). Methodologies of risk stratification evolved in pediatric and congenital cardiac surgery because, unlike other specialties such as adult cardiac surgery (in which a large numbers of individual operations, such as coronary artery bypass grafting and aortic valve replacement, are performed), congenital cardiac surgery consists of a wide variety of operations, each of which is performed in relatively small numbers. As a result, any one operation is not performed in large enough numbers to allow for meaningful comparison across hospitals. Therefore strategies were developed to group operations for analysis. In pediatric and congenital cardiac surgery, three methods of risk stratification have been used on a large multi-institutional basis:
- 1.
R isk A djustment for C ongenital H eart S urgery-1 categories (RACHS-1 categories)
- 2.
A ristotle B asic C omplexity levels (ABC levels) and the Aristotle Comprehensive Complexity Score
- 3.
The S ociety of T horacic Surgeons (STS)–European A ssociation for Cardio- T horacic Surgery (EACTS) Congenital Heart Surgery Mortality Categories (STAT Mortality Categories)
RACHS-1 categories were reported in 2002 and introduced into the STS Congenital Heart Surgery Database (STS CHSD) Feedback Report in 2006. The RACHS-1 methodology groups operations into six categories based on discharge mortality. With few exceptions, additional patient and disease specific factors are not considered. Operations with similar mortality rates were placed together so that operations with the lowest expected mortality are in category one and operations with the highest expected mortality are in category six. RACHS-1 was created using a combination of judgment-based and empirical methodology. RACHS-1 categories are used today in the analyses of administrative data because, unlike ABC levels and STAT Mortality Categories, the RACHS-1 methodology includes the linking of the International Classification of Diseases, Ninth Revision, Clinical Modification (ICD-9-CM) codes for the various operations to the mortality risk categories. A list of the RACHS-1 categories is provided in Online Appendix 1 .
The ABC score and the ABC level were introduced into the STS CHSD Feedback Report in 2002. The ABC score and the ABC level are measures of procedural complexity based on the potential of mortality, the potential of morbidity, and technical difficulty of the operation. A listing of the ABC score and the ABC level values are provided in Online Appendix 2 .
The Aristotle Comprehensive Complexity Score addressed a perceived limitation of RACHS-1 by adding patient- and disease-specific variables that were felt to increase the complexity of the operation and influence the outcome (particularly mortality) of a surgical repair. Thus a 2.8-kg infant receiving complete repair of tetralogy of Fallot would have the additional patient-specific risk factor of young age and small weight compared to an elective tetralogy of Fallot repair on a 5-month-old. If a child received an aortopulmonary shunt in infancy because the child had an anomalous coronary artery, the anomalous coronary artery would be counted as a disease-specific risk factor in the Aristotle Comprehensive Complexity Score. Many of the patient-specific factors and disease-specific factors described in the Aristotle Comprehensive Complexity Score were ultimately incorporated into the STS CHSD Mortality Risk Model.
The STAT Mortality Score and STAT Mortality Categories are an empirically derived methodology of complexity stratification based on statistical estimation of the risk of mortality from an analysis of objective data from the STS CHSD and the EACTS Congenital Heart Surgery Database. The STAT Mortality Score and STAT Mortality Categories were based on analysis of 77,294 operations entered in the STS CHSD and the EACTS Congenital Heart Surgery Database (EACTS, 33,360 operations; STS, 43,934 operations) and introduced into the STS CHSD Feedback Report in 2010. Procedure-specific mortality rate estimates were calculated using a Bayesian model that adjusted for small denominators. Operations were sorted by increasing risk and grouped into five categories that were designed to minimize within-category variation and maximize between-category variation. A listing of the STAT Mortality Categories is provided in Online Appendix 3 .
Like RACHS-1 categories and ABC levels, the STAT Mortality Score and STAT Mortality Categories group operations based on the risk of in-hospital mortality. Unlike RACHS-1 and Aristotle, which use expert opinion (subjective probability) to group operations, operations were placed in the five STAT Mortality Categories based on actual objective mortality rates derived from the STS CHSD and the EACTS Congenital Heart Surgery Database.
STAT Mortality Categories include more operations and have better discrimination than either RACHS-1 categories or the ABC score. Regarding case inclusion, previously published data reveal that of the eligible cardiac index operations, 85.8% were eligible for analysis by the RACHS-1 method and 94.0% were eligible for analysis by the ABC approach. Meanwhile, in the spring 2017 STS CHSD Feedback Report, 98.5% of all eligible cardiac index operations were eligible for analysis with STAT Mortality Categories. Regarding discrimination, the initial publication of STAT Mortality categories documented that the STAT Mortality Categories have better discrimination than RACH-1 or Aristotle. In the subset of procedures for which RACHS-1 categories and ABC scores are defined, discrimination was highest for the STAT Mortality Score (C-index = 0.787), followed by STAT Mortality Categories (C-index = 0.778), RACHS-1 categories (C-index = 0.745), and ABC scores (C-index = 0.687). When patient covariates were added to each model, the C-index improved: STS-EACTS score (C-index = 0.816), STS-EACTS categories (C-index = 0.812), RACHS-1 categories (C-index = 0.802), and ABC scores (C-index = 0.795).
Importance of Disease- and Patient-Specific Factors
All four methods of risk stratification (RACHS-1, ABC score, STAT Mortality Score, and STAT Mortality Categories) classify patients by the operation performed. However, when analyzing outcomes, one must also account for unique features of a given patient: patient-specific factors and procedure-specific factors. Although RACHS-1, ABC score, STAT Mortality Score, and STAT Mortality Categories were all designed to be maximally homogeneous with respect to estimated mortality risk, residual variation still exists in risk across procedures within the same category.
Congenital heart disease is complicated. Moreover, every child is different. However, providers tend to group children into categories of heart defects and report outcomes according to these categories. Although this approach facilitates investigation of questions related to “outcomes science” and education, it may not necessarily direct the best treatment for each child. The concept of precision medicine, which is rapidly becoming the preferred approach, is based upon the concept of individualized care that integrates existing information but also accounts for the unique characteristics of any given patient.
A child with a specific heart defect may have disease-specific risk factors, and he or she may also have patient-specific risk factors. If these factors are associated with an outcome, it is important to know what these factors are so that children with and without such factors can be considered differently. It is also important to understand that many of these patient- and disease-specific factors can influence one another (interact) and therefore may behave differently in certain combinations or in specific patients. Examples of disease-specific risk factors are anatomic or physiologic variances that might be associated with a heart defect and that change the risk for that disease entity. For example, two babies with tetralogy of Fallot may be quite different if one has a left anterior descending artery from the right coronary artery crossing the right ventricular outflow tract or a very small or atretic pulmonary valve whereas the other does not. These anatomic subtypes may increase the risk or change the nature of surgery (thus leading to a different subset of potential complications or outcomes) compared to a child with a normal coronary pattern or a reasonably normal-sized pulmonary valve. Therefore simply compiling results for the defect tetralogy of Fallot will not account for the added incremental risk or differences conferred from these disease-specific features. Similarly, patients with hypoplastic left heart syndrome (HLHS) can be divided into four typical anatomic variants (aortic atresia with mitral atresia, aortic stenosis with mitral atresia, aortic atresia and mitral stenosis, and aortic stenosis with mitral stenosis). However, one of these (aortic atresia and mitral stenosis) has a higher prevalence of coronary abnormalities, which increases the risk of a poor outcome. If one investigates outcomes for all patients with HLHS without accounting for this specific characteristic, the results will not represent an accurate portrayal of the risk of an individual patient, and outcomes at institutions that include a high number of these “higher-risk” patients may be substantially different than institutions without patients with this anatomic subtype. Most outcome reports lump together all patients with HLHS; however, failure to distinguish important disease-specific risk factors may contribute to differences between institutional outcomes. Luckily, for most congenital heart defects, many of the unfavorable morphologic variants are known from past study, allowing the compilation of a fairly comprehensive list of common disease-specific factors that can influence the outcome and even the treatment strategy.
The ability to interpret outcome data is further complicated by patient-specific factors, such as patient age, weight, other noncardiac congenital anatomic abnormalities, chromosomal abnormalities, genetic syndromes, or concurrent illness. The STS CHSD defines and captures data about both procedure-specific factors and patient-specific factors, and these variables are included in the current STS CHSD data specifications and STS CHSD data collection forms, as well as the current STS CHSD Mortality Risk Model. These factors can have variable influence on both a preferred treatment strategy (including timing of intervention) and expected outcomes for the prescribed treatment strategy.
Risk Modeling
RACHS-1, ABC score, STAT Mortality Score, and STAT Mortality Categories all classify patients by the operation performed. In all three cases, mean mortality rates were used to determine to which category an operation was assigned. One patient who undergoes a certain operation may have a low expected mortality risk while another undergoing the same operation may have a high mortality risk; however, they are both in the same category because it is the mean mortality rate of all patients undergoing that operation that was considered for classification rather than individual situations. To account for patient-specific factors and procedure-specific factors, STS developed the STS CHSD Mortality Risk Model. Variables included in the STS CHSD Mortality Risk Model are listed in Table 4.1 .
|
a Modeled as a piecewise linear function with separate intercepts and slopes for each STS-defined age group (neonate, infant, child, adult).
b The model adjusts for each combination of primary procedure and age-group. Coefficients obtained via shrinkage estimation with the Society of Thoracic Surgeons–European Association for Cardio-Thoracic Surgery (STS-EACTS [STAT]) Mortality Category as an auxiliary variable.
c Any other preoperative factor is defined as any of the other specified preoperative factors contained in the list of preoperative factors in the data collection form of the STS Congenital Heart Surgery Database, exclusive of 777 = “Other preoperative factors.”
Over the past 20 years the STS CHSD has expanded to include data from over 435,000 operations from 127 congenital heart surgery hospitals in North America—124 in the United States and 3 in Canada. Therefore the STS CHSD contains data from approximately 96% of pediatric heart surgery programs in the United States and approximately 98% of pediatric heart surgery operations performed in the United States. Because of the large amount of data submitted to the STS CHSD and the nearly universal participation among congenital cardiac surgery centers in the United States, robust risk adjustment that accounts for a large number of influential patient- and disease-specific factors is possible.
The STS CHSD Mortality Risk Model was developed from an analysis of 52,224 index cardiac operations from 86 centers during the 4-year analytic window of January 1, 2010, to December 31, 2013. The 2014 STS-CHSD Risk Model has the highest c-index of any pediatric and congenital cardiac surgical risk model to date (c-index in developmental sample = 0.875, c-index in validation sample = 0.858) and is the state of the art in pediatric and congenital cardiac surgical risk adjustment. A c-index represents the likelihood that the results are more than random. For example, in a random model, such as a coin flip, the c-index is 0.5 because the likelihood of heads versus tails is 50/50. The model undergoes recalibration with updating of the coefficients in the model on a twice-yearly basis to coincide with the production of each STS CHSD Participant Feedback Report.
The following information is available in Table 16 of the STS CHSD Participant Feedback Report and is based on the STS CHSD Mortality Risk Model :
- •
Observed mortality rate is a percentage calculated by dividing the number of observed deaths by the number of eligible patients included in the calculation. This percentage is often referred to as the “raw mortality rate” or the “unadjusted mortality rate.”
- •
Expected mortality rate is a percentage that reports the number of expected deaths for the given case mix, using the STS CHSD Mortality Risk Model. The STS CHSD Mortality Risk Model is used to estimate the number of expected patient deaths when considering the unique case mix of an STS CHSD participant or the mix of patients treated as defined by all of the variables listed in Table 4.1 .
- •
Observed-to-expected (O/E) ratio is the number of observed deaths divided by the number of expected deaths. An O/E ratio greater than 1 means that the STS CHSD participant had more deaths than expected based on the actual case mix of that STS CHSD participant. An O/E ratio of less than 1 means that the STS CHSD participant had fewer deaths than expected based on the actual case mix. Small differences in the O/E ratio are usually not statistically significant, which is why the O/E ratio is reported along with 95% confidence intervals.
- •
Adjusted mortality rate (AMR) is an estimate (based on the STS CHSD Mortality Risk Model) of what the hospital’s mortality rate would be if its observed performance was extrapolated to the overall STS case mix (specifically, the mix of age, weight, procedure types, and other model-specific variables, including prior cardiothoracic operations, noncardiac congenital anatomic abnormalities, chromosomal abnormalities, syndromes, and preoperative risk factors). It is calculated by the following formula: AMR of hospital = O/E ratio of hospital × overall observed STS mortality rate. Small differences in the AMR are usually not statistically significant, which is why AMR is reported along with 95% confidence intervals.
Importance of Risk Adjustment
The importance of risk adjustment can be seen in the following example. The volume-mortality relationship in pediatric cardiac surgery was investigated using clinical data from the STS CHSD. Average annual program case volumes were categorized into four groups: small (<150 cases per year), medium (150 to 249 cases per year), large (250 to 349 cases per year), and very large (>350 cases per year). When raw mortality rates were compared, there were no statistical differences in mortality between the four volume groups. However, this homogeneity was misleading. Small hospitals tended to operate on fewer neonatal patients and as a result fewer high-complexity patients. After adjustment for patient-level risk factors and surgical case mix, there was an inverse relationship between overall surgical volume as a continuous variable and mortality. Examined categorically, the mortality rate at small programs was significantly worse than that at very large programs. In addition, the volume-mortality relationship varied based on case difficulty. For low-difficulty operations, all four volume groups performed similarly. In contrast, for high-difficulty operations, small programs had substantially higher discharge mortality relative to very high-volume programs. For the Norwood procedure, very high-volume programs outperformed all other volume groups. In congenital cardiac surgery, volume alone is an imperfect discriminator of mortality. Patient and surgical case mix–adjusted data are essential for identifying better-performing, higher-quality hospitals. Furthermore, there are undoubtedly other factors that are not considered in databases and that exist besides volume, such as the experience or unique skills of individual members of the team or the ability of some groups to work as a team with higher performance than groups that are unable to work as an effective team regardless of volume. This concept helps to explain how some small programs may perform consistently well and better than volume alone might predict.
Alternative Approaches
Most surgical registries, including the STS CHSD, are procedurally based. Patients are entered only if they receive surgery at a participating institution. Similarly, the STAT Mortality Categories are procedurally based. An alternative approach of analysis is the use of a diagnosis-based database, but the challenge for attaining these data from a variety of sources is quite daunting because it would require entering ALL patients born with congenital heart disease, regardless of what management strategy is employed (e.g., observation, medical management, catheter-based intervention, or surgery). On a less daunting scale, however, one could analyze outcomes of all patients treated with surgery stratified into diagnostic groups rather than procedural groups, and some of these diagnoses-based analyses are already present in the STS CHSD Participant Feedback Report.
No clinical database currently exists that includes all congenital cardiac patients regardless of whether or not they undergo surgery or transcatheter intervention. Such a diagnosis-based database would certainly be valuable. However, our current procedurally based database does provide a significant body of information upon which to try and understand the critically ill patients with congenital heart disease that have undergone surgery (who represent most of the patients we care for in our intensive care units). Together, the patient- and disease-specific factors, along with the risk-stratification algorithm, allow benchmarking of center-level performance compared to other national aggregate data.
Numerous factors impact a patient’s risk of mortality. Although the focus of most assessments of mortality is on the performance of the congenital cardiac surgeon and the care provided by the congenital cardiac team, these elements are only part of the picture. The majority of a patient’s mortality risk is determined by factors related to the patient themselves rather than the care they receive. Karamlou and colleagues used the Congenital Heart Surgeons’ Society data sets to analyze 2421 complex neonatal operations. They found that institutional performance varied among groups and that institutional excellence in managing one particular group of patients did not translate into excellence in other groups. Moreover, surgeon and center volume (or other experience domains) influenced outcomes in only one of the defects studied (transposition of the great arteries [TGA]). Patient or disease factors, such as low birth weight or anatomy, were the most influential in all other complex groups. Risk stratification systems and mortality risk models are designed therefore to account for a portion of this risk. Most of the current models, as we have seen, are dominated by a variety of patient-specific and disease-specific factors such as anatomy or weight; some, however, might be improved by including management strategies or processes (including the date of surgery in relationship to admission or birth, or intubation or feeding status). Some of these factors are modifiable, and some are not. For example, a patient with an active respiratory illness who needs an operation requiring cardiopulmonary bypass will have a lower risk of mortality if the patient can wait until the illness has resolved. In other words, the patient’s risk of death may be lowered by delaying the operation. A 3-year-old who undergoes elective repair of a secundum atrial septal defect has a lower mortality risk than a neonate undergoing a Norwood procedure regardless of the center where they undergo surgery. In this case the patient’s risk cannot be modified because the patient’s diagnosis cannot be changed. Patient outcomes may be improved by addressing modifiable risk factors so that patients arrive in the operating room in optimal condition for surgery. In addition, systemic factors or infrastructure that transcend the congenital cardiac surgical team may have an important impact on patient outcomes. This interdependence and shared resource necessity is highlighted in the paper by Caddell and colleagues, which investigated the relationship between outcomes within complex pediatric surgical specialties. These authors concluded that “Hospitals with low mortality rates for 1 high-risk pediatric surgical specialty tended to have low rates for other specialties. This observation suggests that diverse surgical fields share institutional resources and processes that affect their mutual performance. Implementation of these common pillars may lead to broader improvements in quality than efforts focused on individual disciplines.” The finding that rates of mortality for seemingly disparate pediatric surgical specialties (cardiac surgery, neurosurgery, general surgery) were correlated suggests that shared institutional resources and processes affect their mutual performance.
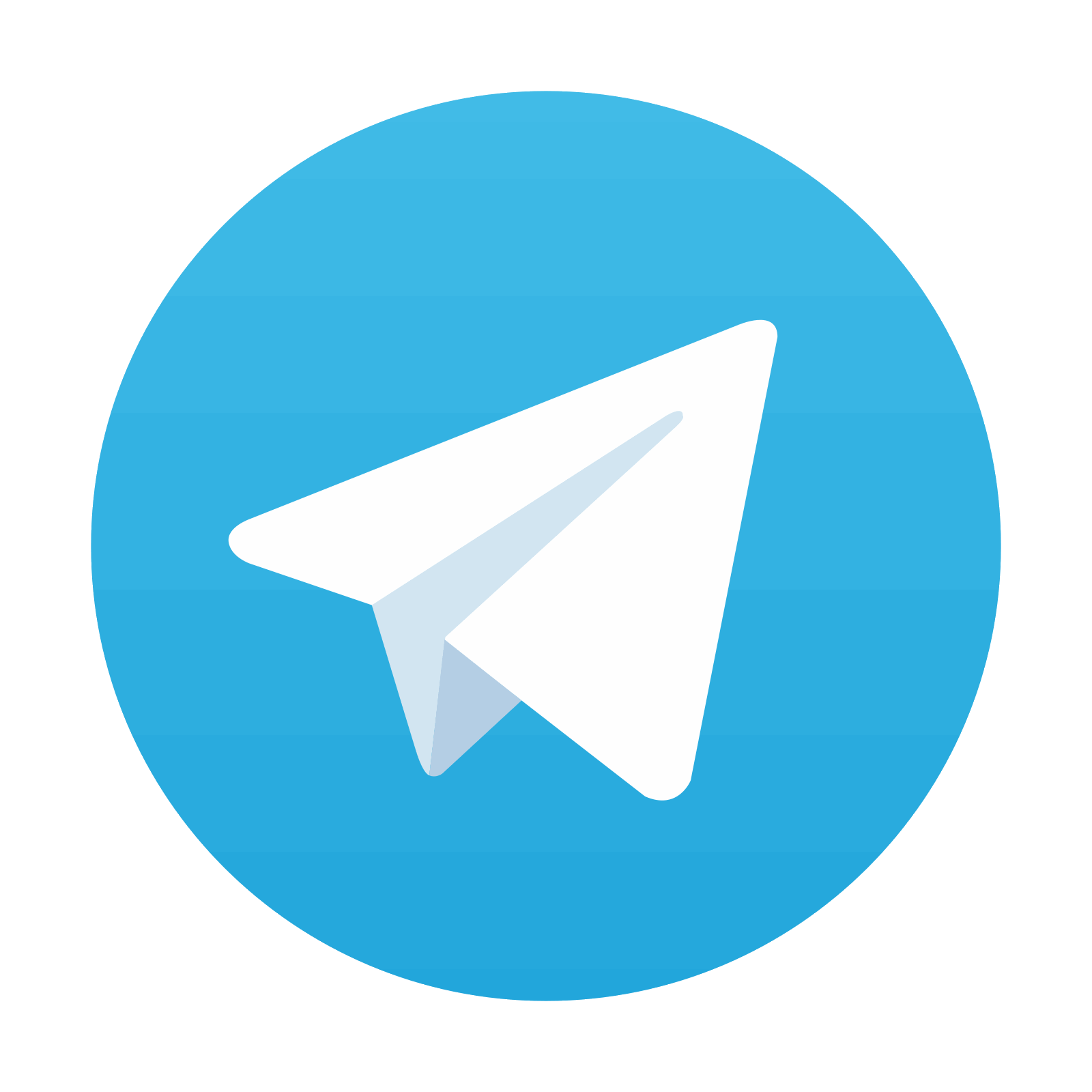
Stay updated, free articles. Join our Telegram channel

Full access? Get Clinical Tree
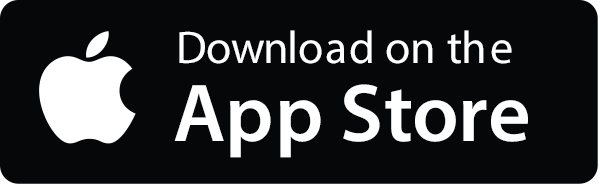
