Abstract
It has long been known that there is a heritable component to coronary artery disease (CAD). However, identifying the actual genetic loci influencing CAD risk was not possible prior to recent advances in molecular biology that have facilitated the identification of genomic loci with statistically very strong, i.e., genome-wide significant, associations with CAD. Several previously unrecognized pathophysiological mechanisms have been discovered with high-throughput genotyping and the emergent exome and whole genome sequencing. Most of the novel genetic variants that modulate CAD risk do so by completely new mechanisms. However, the identification of the underlying pathophysiology is just beginning. Using the genetic variants affecting risk as a starting point, further investigations can help to identify druggable targets and thus improve prevention and therapy of the disease. Our goal is to aid in the fast evolution of this field of research. Using recent examples, we want to point to the opportunities in prevention and therapy due to the novel knowledge of genetic risk.
Keywords
Coronary artery disease, myocardial infarction, genome-wide association studies, atherosclerosis, next-generation sequencing, risk genes, systems medicine, personalized medicine
Introduction
Ischemic heart disease is the leading cause of death in western countries. Bearing in mind that acute myocardial infarction and congestive heart failure range nearly behind, the importance of coronary atherosclerosis gets even more evident.
Modifiable risk factors of atherosclerosis can be distinguished from nonmodifiable risk factors, whereas risk factors as smoking, arterial hypertension, and diabetes can be favorably affected by lifestyle modification or pharmacological interventions , age and gender are not modifiable ( Fig. 8.1 ). Lipid metabolism disorders represent somehow idiosyncrasies as they are on one hand modifiable by pharmacological therapies, but on the other hand, often caused by an in-principle nonmodifiable genetic basis, e.g., familial hypercholesterolemia.

Positive family history, which is also expression of the genetic burden an individual carries, is another nonmodifiable risk factor. Individuals with a near relative suffering from myocardial infarction at young age have themselves an increased risk for coronary artery disease (CAD) which is depending on the degree of kinship ( Fig. 8.2 ). In the last years, the results from genome-wide association studies (GWAS) have shown that this genetic basis goes far beyond just a positive family history and cannot be reduced to just one affected gene. In fact, each individual, independent of a positive family history, carries a large number of genetic risk alleles. The sum (or interactive effects) of these risk alleles rather constitutes the genetic risk of a person.

The search for genetic variants causing CAD began with a limited number of candidate genes and then moved on to a search throughout the genome as technology improved. Initially, genes encoding for proteins known to modulate traditional risk factors were the main targets of the search for genetic risk factors which was due to the limited technical possibilities. Causal involvement in the disease process could indeed be shown for the genes encoding the low-density-lipoprotein (LDL) cholesterol receptor and lipoprotein (a), LDLR and LPA , respectively. In many cases, however, no relationship was found between genetic polymorphisms and the manifestation of CAD. A hypothesis-free approach that queried variants across the entire genome proved to be more effective. With deciphering of the human genome and the annotation of single-nucleotide polymorphisms (SNPs), the search for variants associated with coronary disease risk throughout the whole genome became possible by GWAS.
Genome-Wide Association Studies
GWAS effectively represent all common [minor allele frequency (MAF)>1%–5%] variants throughout the genome. They have strict statistical procedures for analysis and interpretation, which reduces the propensity for false genetic findings (for further information on GWAS, please see , http://www.nchpeg.org , or http://www.genome.gov ). While large studies of complex traits have identified hundreds of genetic variants that are associated with genome-wide significance, the effect sizes of these variants tend to be very small. Additionally, GWAS hit tag a genomic region as opposed to identifying a specific causal variant, and identifying the causal variant once a genomic region has been identified has proved to be a daunting task for most studied traits.
To date, more than 60 genomic loci have been identified to be genome-wide significantly associated with CAD ( Table 8.1 ). The association is only proven for SNPs. Hence, the genes that are annotated to each of these loci are usually those that are situated most closely to the SNP. As most of the SNPs are not located in coding regions, it is not sure whether these genes are actually underlying the association signal or whether the SNP might influence another gene. However, only a few of these genes had been previously implicated to play a pathophysiological role in CAD, e.g., the gene encoding the LDLR . As a matter of fact, a just little larger number of the identified genes in this list of genome-wide significantly associated genes is associated with traditional risk factors as lipid levels, e.g., the already mentioned LDLR and LPA loci, or arterial hypertension.
Chromosome | Lead SNP | RAF | OR | Gene(s) at Chromosomal Locus | Refs. |
---|---|---|---|---|---|
1 | rs11206510 | T (0.82) | 1.08 | PCSK9 | |
rs17114036 | A (0.91) | 1.17 | PPAP2B | ||
rs17465637 | C (0.74) | 1.14 | MIA3 | ||
rs599839 | A (0.78) | 1.11 | SORT1 | ||
rs4845625 | T (0.47) | 1.06 | IL6R | ||
2 | rs6544713 | T (0.30) | 1.06 | ABCG5/ABCG8 | |
rs6725887 | C (0.15) | 1.14 | WDR12 | ||
rs515135 | G (0.83) | 1.07 | APOB | ||
rs2252641 | G (0.46) | 1.06 | ZEB2 | ||
rs1561198 | A (0.45) | 1.06 | VAMP5-VAMP8-GGCX | ||
rs1801251 | A (0.35) | 1.05 | KCNJ13-GIGYF2 | ||
3 | rs2306374 | C (0.18) | 1.12 | MRAS | |
4 | rs7692387 | G (0.81) | 1.08 | GUCY1A3 | |
rs1878406 | T (0.15) | 1.10 | EDNRA | ||
rs17087335 | T (0.21) | 1.06 | REST-NOA1 | ||
5 | rs2706399 | G (0.51) | 1.07 | IL5 | |
rs273909 | C (0.14) | 1.07 | SLC22A4-A5 | ||
6 | rs12526453 | C (0.67) | 1.10 | PHACTR1 | |
rs17609940 | G (0.75) | 1.07 | ANKS1A | ||
rs12190287 | C (0.62) | 1.08 | TCF21 | ||
rs3798220 | C (0.02) | 1.51 | LPA, SLC22A3, LPAL2 | ||
rs10947789 | T (0.76) | 1.07 | KCNK5 | ||
rs4252120 | T (0.73) | 1.07 | PLG | ||
rs3130683 | T (0.86) | 1.09 | C2 | ||
7 | rs10953541 | C (0.80) | 1.08 | BCAP29 | |
rs11556924 | C (0.62) | 1.09 | ZC3HC1 | ||
rs2023938 | G (0.10) | 1.08 | HDAC9 | ||
rs3918226 | T (0.06) | 1.14 | NOS3 | ||
8 | rs2954029 | A (0.55) | 1.06 | TRIB1 | |
rs264 | G (0.86) | 1.11 | LPL | ||
9 | rs4977574 | G (0.46) | 1.29 | 9p21.3 | |
rs579459 | C (0.21) | 1.10 | ABO | ||
rs111245230 | C (0.04) | 1.14 | SVEP1 | ||
10 | rs2505083 | C (0.38) | 1.07 | KIAA1462 | |
rs1746048 | C (0.87) | 1.09 | CXCL12 | ||
rs1412444 | T (0.42) | 1.09 | LIPA | ||
rs12413409 | G (0.89) | 1.12 | CYP17A1, NT5C2 | ||
11 | rs974819 | T (0.32) | 1.07 | PDGFD | |
rs964184 | G (0.13) | 1.13 | APOA1-C3-A4-A5 | ||
rs11042937 | T (0.49) | 1.04 | MRVI1-CTR9 | ||
12 | rs10840293 | A (0.55) | 1.06 | SWAP70 | |
rs3184504 | T (0.44) | 1.07 | SH2B3, HNF1A | ||
rs11830157 | G (0.36) | 1.12 | KSR2 | ||
rs11172113 | C (0.41) | 1.06 | LRP1 | ||
rs11057830 | A (0.15) | 1.08 | SCARB1 | ||
13 | rs4773144 | G (0.44) | 1.07 | COL4A1, COL4A2 | |
rs9319428 | A (0.32) | 1.06 | FLT1 | ||
14 | rs2895811 | C (0.43) | 1.07 | HHIPL1 | |
15 | rs3825807 | A (0.57) | 1.08 | ADAMTS7 | |
rs17514846 | A (0.44) | 1.07 | FURIN-FES | ||
rs56062135 | C (0.79) | 1.07 | SMAD3 | ||
rs8042271 | G (0.9) | 1.10 | MFGE8-ABHD2 | ||
16 | rs1800775 | C (0.51) | 1.04 | CETP | |
17 | rs216172 | C (0.37) | 1.07 | SMG6-SRR | |
rs12936587 | G (0.56) | 1.07 | PEMT, RASD1, SMCR3 | ||
rs46522 | T (0.53) | 1.06 | UBE2Z, GIP, ATP5G1 | ||
rs7212798 | C (0.15) | 1.08 | BCAS3 | ||
18 | rs663129 | A (0.26) | 1.06 | PMAIP1-MC4R | |
19 | rs116843064 | G (0.98) | 1.14 | ANGTPL4 | |
rs1122608 | G (0.77) | 1.14 | LDLR | ||
rs2075650 | G (0.14) | 1.14 | APOE | ||
rs12976411 | A (0.91) | 1.33 | ZNF507-LOC400684 | ||
21 | rs9982601 | T (0.15) | 1.18 | MRPS6, SLC5A3, KCNE2 | |
22 | rs180803 | G (0.97) | 1.20 | POM121L9P-ADORA2A |
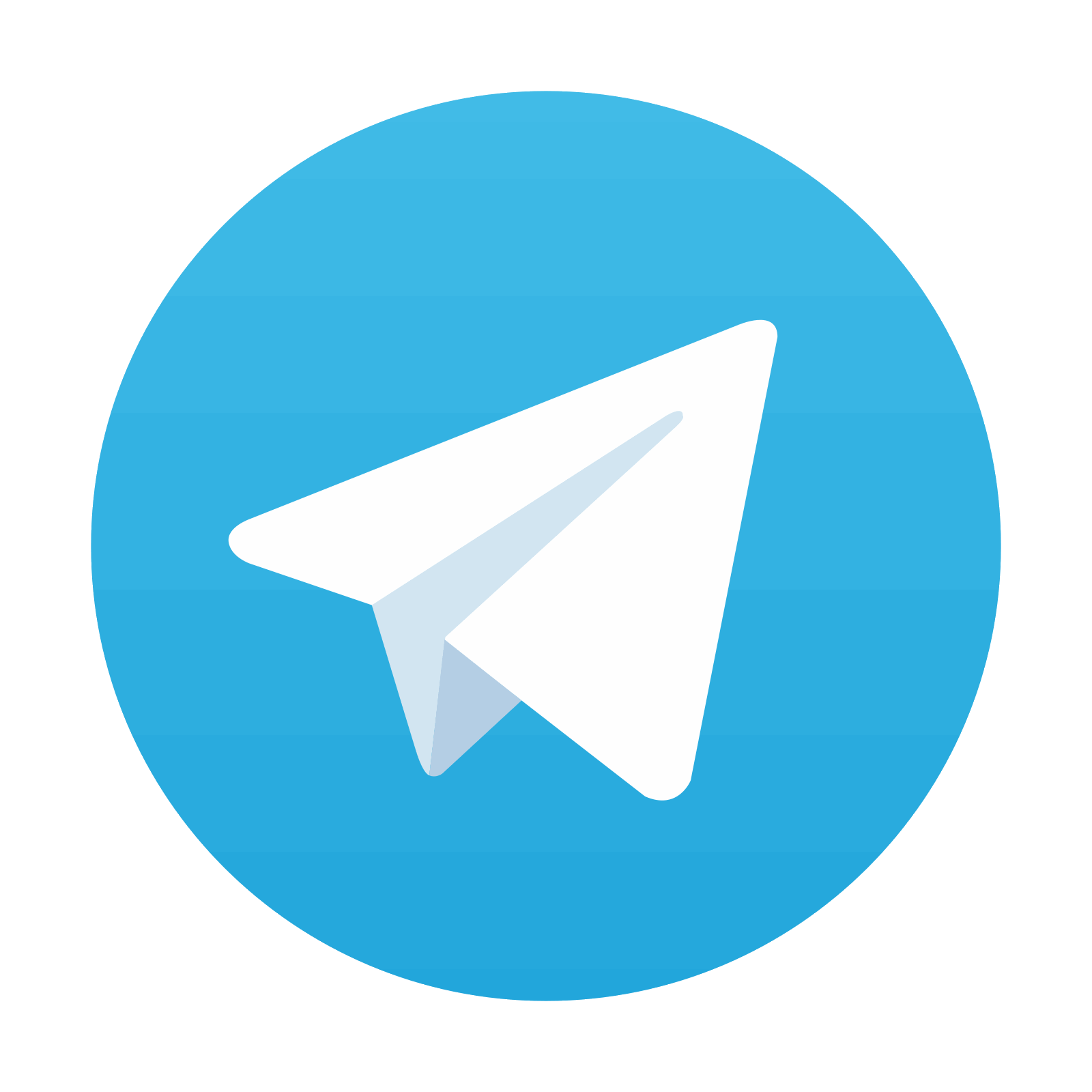
Stay updated, free articles. Join our Telegram channel

Full access? Get Clinical Tree
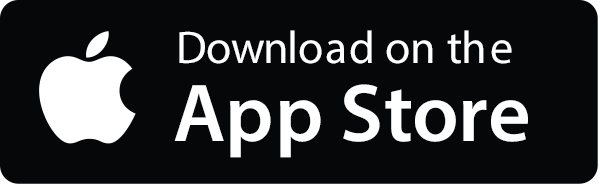

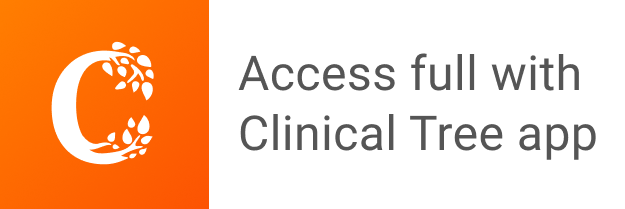