Locus
Gene
Trait
Reference
1p32.3
PCSK9
LDL cholesterol
[59]
2p24.1
APOB
Total and LDL cholesterol
[60]
3p25
PPARG
HDL cholesterol
[61]
5q31
SEPP1
Insulin resistance
[62]
6q25.3
LPA
Lipoprotein(a)
[63]
8p21.3
LPL
HDL cholesterol
[61]
9q31.1
ABCA1
HDL cholesterol, Triglycerides
11q23
APOA1/C3/A5
HDL cholesterol, Triglycerides
[66]
11q23
APOA5
Triglycerides, Metabolic syndrome
[67]
12q24.31
SCARB1
CAD, ischemic stroke
[68]
16q21
CETP
Coronary artery stenosis severity, Postprandial lipemia
16q22.1
LCAT
HDL cholesterol
[72]
19q13.32
APOE
Total and LDL cholesterol, Age of CAD onset
20q13.12
PLTP
HDL cholesterol
[75]
Unfortunately, the results of many candidate gene studies have been inconsistent and were not replicated under further scrutiny. Some reported “positive” associations are the result of false findings and “negative” publication bias. A combination of small variant effect and a low sample size could lead to type 2 error which is the probability of a false negative result due to low statistical power. The probability of detecting association by chance (false positive) leads to type 1 error. Positive results are easier to publish, while negative studies, even if better-designed, may not reach criteria for publication and the significance of positive associations may be overestimated. The cardiovascular literature is enriched with positive genetic association studies, though few have true clinical value and have been helpful to clinical practice. Among the reasons for failing to replicate findings are incorrect SNP selection, variable definitions for cases and controls in different studies, important differences in enrolled cases due to variations in investigators’ clinical skills, failure to control confounding variables, lack of sufficient power (insufficient number of participants) and genetic and phenotypic heterogeneity. Phenotypic definition is of great importance when stratifying cases and controls. For example, in association studies for CAD, MI should be separated from other angiographic coronary disease phenotypes, since different genes may be involved in each process [58, 76].
7.5.3 Genome Maps
The effort of mapping and pinpointing susceptibility genes was facilitated by the construction and availability of genetic maps bearing known DNA markers [77]. In 1980, a map of RFLPs was initially proposed and this first map contained 403 polymorphic loci including 393 RFLPs [55]. It was estimated that the linkage map was detectably linked to at least 95 % of the DNA in the human genome [78]. In 1990s, microsatellite markers were also included in maps due to their high level of polymorphism [35]. A great collaborative effort to map more DNA markers, initiated in 1990 by the Centre d’Etude du Polymorphism Humain (CEPH) in which 63 research laboratories from United States, Canada, Europe, South Africa, Japan and Australia participated [79, 80]. The present version of the database (V10.0 – November 2004) contains genotypes for 32,356 genetic markers including 21,480 biallelic markers and >9,900 microsatellite markers. The mean observed heterozygote frequency of all the loci is 0.438 and for identifiable microsatellite loci 0.698, of which 56 % are highly polymorphic (observed heterozygote frequency ≥ 0.70). The CEPH database now manages 6,081,570 genotypes [81].
In October 2002, another great step was made towards a high-density SNP map (or haplotype map) construction covering the entire genome. The International Hapmap Project (http://www.hapmap.org/) was initiated for establishing a SNP database for populations with ancestry from parts of Africa, Asia and Europe. The aims of the International HapMap Project was to determine the common patterns of DNA sequence variation in the human genome and to make this information freely available in the public domain. Exploiting such information, The HapMap was intended to assist in the discovery of sequence variants that affect common disease, to facilitate development of diagnostic tools, and to enhance our ability to choose targets for therapeutic intervention [82]. In stage I (completed in 2005), more than one million SNPs were genotyped in 269 DNA samples from four populations: the Yoruba in Ibadan, Nigeria (YRI), Utah, USA, from the CEPH collection (CEU), Han Chinese in Beijing, China (CHB) and Tokyo, Japan (JPT). These data documented the generality of recombination hotspots, a block-like structure of linkage disequilibrium and low haplotype diversity, leading to substantial correlations of SNPs with many of their neighbors (tag SNPs) [83]. Tag SNPs are covering the whole genome and are able to represent neighboring SNPs with high accuracy, without the need for genotyping them directly. This is known as genotype imputation (i.e. genotype guessing) [2]. In stage II (completed in 2007), over 3.1 million human SNPs were genotyped in 270 individuals from the same population. The resulting HapMap database yielded a SNP density of approximately 1 SNP per 1 kilobase and is estimated to contain approximately 25–35 % of all the 9–10 million common SNPs (minor allele frequency ≥0.05) in the assembled human genome [84].
7.5.4 Genome-Wide Association Studies
The information available from the completion of The Hapmap Project along with the development of large-scale genotyping SNP chips (microarrays) shifted research for common diseases, like CVD, from single/few gene associations to genome-wide association studies (GWAS). GWAS, also known as whole-genome association studies, involve the examination of genetic variation across a given genome using hundreds of thousands of SNPs (500,000–1 million) on DNA arrays. The SNPs throughout the genome are printed in large series of disease cases and disease-free controls [57]. There are two main companies offering high-throughput genome-wide scanning, Affymetrix and Illumina and the cost is decreasing with time. In contrast to candidate approach, in GWAS no a priori biological hypothesis is needed and previously unsuspected genes can be identified. GWAS are based on the concept of “common disease – common variant hypothesis” where common polymorphisms (minor allele frequency > 5 %) are believed to influence, in part (low effect size), genetic susceptibility to common diseases. As a consequence, very large sample sizes (>100,000 subjects) are needed to achieve statistical power to detect more variants associated with the disease under investigation. However, in GWAS the likelihood of false positive associations is high and thus stringent criteria are applied in order to declare true positive associations. Bonferroni correction adjusts the threshold of significance by dividing P = 0.05 with the number of tests and thus the P-value is often P < 10−8 which is known as the “genome-wide significance” [14, 39]. Even though applying such stringent statistical methods, positive results should always be replicated by independent cohorts. Regarding reproduction, increased sample size and meta-analysis could result in the identification of associations missed by individual GWAS. In GWAS, odds ratios (ORs) provide an estimate of the risk conferred by an allele in a given SNP. An allele with OR > 1.0 is associated with increased probability of disease in carriers of this allele and thus it is considered as a risk allele [39]. It is important to note that GWAS do not test directly SNPs that alter structure or function and induce pathogenicity. Instead, they test tag SNPs, in other words SNPs that are linked to functional variants through linkage disequilibrium. The gene closest to an association signal that makes biological sense is nominated as the candidate one.
The expected outcomes of GWAS concerning CVD are the following: (1) the identified SNPs could lead to genomic regions carrying genes that influence new molecular mechanisms and pathways and, (2) SNP results could help to diagnose and treat specific patients which may lead to personalize treatment strategies [39]. GWAS have successfully confirmed already established loci and identified novel ones for CAD and its risk factors (diabetes mellitus, blood pressure, dyslipidemias) and are listed in Table 7.2. Although the responsible gene is clear in some studies, most genomic regions possess multiple candidate genes and therefore, further research is needed in order to filter out irrelevant variants. Additionally, GWAS have resulted in some novel loci bearing genes with either an unknown function or without a clear connection with CAD-related mechanisms. An example of this is the chr9p21.3 locus which appears to be GWAS hotspot and for many years it remains the strongest and most significant determinant of the risk for CAD and other CVD phenotypes (type 2 diabetes, abdominal aortic aneurysm and intracranial aneurysm) [14]. However, the exact mechanism whereby the chr9p21.3 variation increases CVD risk remains unidentified. The nearest protein coding genes, in relation to tag SNPs, are CDKN2A (150 kilobases) and CDKN2B (118 kilobases) and are known to control cellular proliferation and apoptosis [14]. Functional studies concerning the chr9p21.3 locus have shown that this region seems to have regulatory role on the activity of primary aortic smooth muscle cells. Deletion of the region in mouse models leads to increased expression of CDKN2A and CDKN2B genes and high proliferation of aortic smooth muscle cells [14, 101, 102].
Table 7.2
Results of GWAS analysis of CVD and its risk factors
Disease or trait | Gene | Reference |
---|---|---|
HDL cholesterol | SLC39A8 | [85] |
HDL cholesterol and Triglycerides | LPL | [86] |
LDL cholesterol | MYLIP/GMPR | [85] |
LDL cholesterol | PPP1R3B | [85] |
Triglycerides | AFF1 | [85] |
Triglycerides | APOA5 | [87] |
Ischemic stroke | AGTRL1 | [88] |
Ischemic stroke | HDAC9 | [89] |
Ischemic stroke | PRKCH | [90] |
Ischemic stroke | ARHGEF10 | [91] |
Ischemic stroke | ABO | [92] |
Myocardial infarction | LTA | [93] |
Myocardial infarction | LGALS2 | [94] |
Myocardial infarction | PSMA6 | [95] |
Myocardial infarction | MIAT | [96] |
Myocardial infarction | ITIH3 | [97] |
Coronary artery disease | LIPA | [98] |
Type 2 diabetes | TPMRSS6 | [99] |
Type 2 diabetes | BCL2 | [100] |
Based on the polygenic model of CVD, most variants when considered alone have a very low impact on phenotype. In order to obtain meaningful information for clinical practice, the estimation of “genetic scores” has been recently introduced by combining data from as many as possible variants related with a given phenotype. Twenty SNP variations with additive effect on LDL cholesterol explain 14 % of this lipoprotein’s variance in healthy men and women [103]. Furthermore, 116 independent blood pressure-related SNPs explain approximately 2.2 % of the variance observed in systolic and diastolic blood pressure measurements [104].
Unfortunately GWAS, based on common variation, have explained only a small portion of the expected heritability of CVD risk as previously reported by twin studies. This “missing heritability”, is currently believed, might be explained by rare variants based on the alternative concept of “common disease – rare variant” hypothesis. Next-generation applications such as exome sequencing or whole genome sequencing will enable the identification of rare variants which may provide considerable clues in missing heritability [39].
GWAS are also expected to elucidate our knowledge in the fields of pharmacogenetics and pharmacogenomics. The two terms are often confused and used as one but actually they are quite different. Pharmacogenetics refers to the study of inherited differences (variation) in drug metabolism and response, while pharmacogenomics refers to the general study of the many different genes that determine drug behavior [105]. The information produced by these studies will help predicting the effectiveness, the risk of adverse reactions and the appropriate dose of drugs for each patient leading to personalized medicine [37]. Genetic tests for variations in CYP2C9 and VKORC1 genes are already established, prior to warfarin usage predicting slow metabolizers [106, 107]. Interestingly, two single-blind, randomized trials comparing a genotype-guided (based on CYP2C9 and VKORC1 variations) dosing of acenocoumarol or phenprocoumon did not reveal any improvement on the percentage of time in the therapeutic INR [108]. Concerning lipids, SLCO1B1 is a future biomarker candidate for simvastatin use influencing statin-induced myopathy [109]. Moreover, GATM is considered to be associated with cholesterol homeostatis and it has been recently identified to be associated with differences is susceptibility to statin-induced myopathy [110].
7.6 Conclusions
Technological advances have considerably contributed to our better understanding of the human genome structure. Genetic variability seems to account for most common diseases, such as CVD, as well as for drug response and adverse drug reactions. Genetic variability exists in many forms including SNPs, indels, STRs, VNTRs and CNVs. Most common diseases are complex, influenced by multiple genetic and non-genetic factors, and constitute a major concern of public health. GWAS are currently flourishing producing a massive amount of genetic data and have pointed out previously unsuspected candidate genes. Although GWAS have helped to illuminate previously unknown biological and metabolic pathways, clinical significance in predicting future risk of disease is still low. The study of genetics and its involvement in various disease entities offers an excellent paradigm of Translational Research, since basic research findings eventually find their way to the treatment of the individual patient but also to application in populations. An important issue raised by unraveling the genetic basis of complex traits in humans and yielding so much genetic information at individual level, is the management of this information. Genetic information should be handled with an ethical and confidential way avoiding genetic discrimination. And as Nakamura [37] has said “although we are all different, we should have equal rights and should respect each other’s differences”.
References
1.
Watson JD, Crick FH. Molecular structure of nucleic acids; a structure for deoxyribose nucleic acid. Nature. 1953;171:737–8.PubMed
2.
Bochud M. Genetics for clinicians: from candidate genes to whole genome scans (technological advances). Best Pract Res Clin Endocrinol Metab. 2012;26:119–732.PubMed
3.
Genetics home reference, mitochondrial DNA. 2013. http://ghr.nlm.nih.gov/mitochondrial-dna. Accessed 2 Dec 2013.
4.
Mendel GJ. Versuche uber Pflanzen-Hybriden. Verh Naturforsch Ver Brunn. 1866;4:215–22.
7.
Riordan JR, Rommens JM, Kerem B, Alon N, Rozmahel R, Grzelczak Z, et al. Identification of the cystic fibrosis gene: cloning and characterization of complementary DNA. Science. 1989;245:1066–73.PubMed
< div class='tao-gold-member'>
Only gold members can continue reading. Log In or Register a > to continue
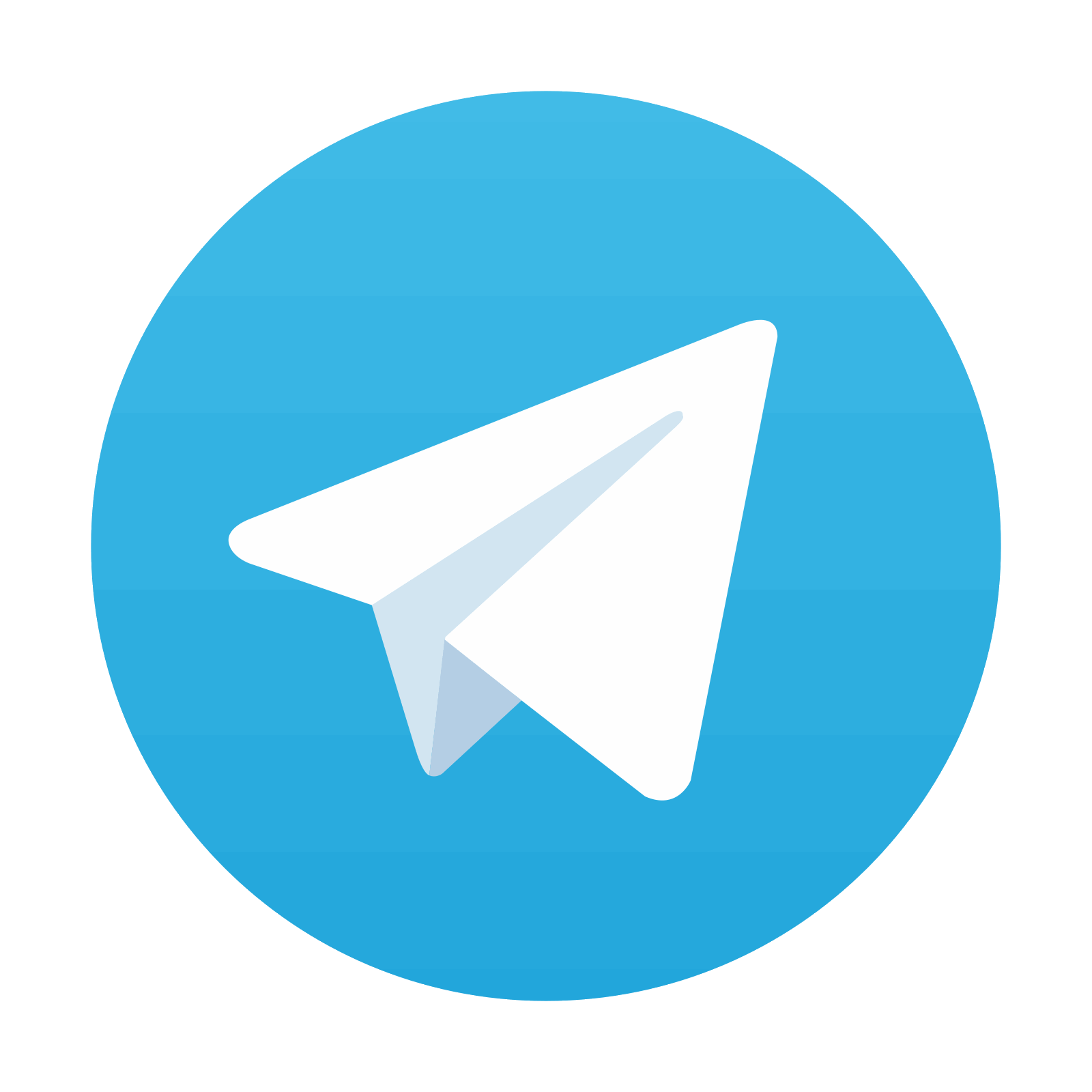
Stay updated, free articles. Join our Telegram channel

Full access? Get Clinical Tree
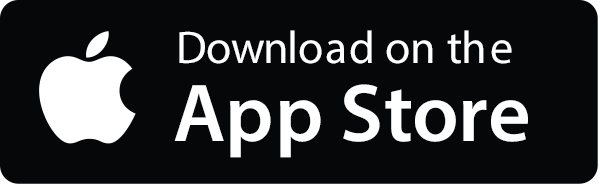
