Fig. 9.1
Conceptual approach to overall study design for genomic assessment of genetic variants, transcriptome, and epigenetic marks in which internal (technical) and external (replication) validation are performed to ensure validity and generalizability of findings from the genomic analysis
The most important consideration in the study design for any genome-wide study is power [17] to detect significant associations given the large number of tests that are performed in the analysis phase. Power depends greatly on the number of individuals in the cohort but also on the effects size which is directly related to the strength and homogeneity of the trait/clinical phenotype of interest. Unfortunately, many of the complex diseases including ALI are heterogeneous in nature and thus an important consideration in the study design phase is whether there is a more homogeneous clinical subphenotype that should be analyzed; this should be balanced with the cohort size. In addition to the trait/clinical phenotype of interest, demographic characteristics such as age, gender, and race/ethnicity need to be considered when designing a genome-wide study as they can be confounders in the analysis if not appropriately taken into account. When using next generation sequencing methods, sequence depth and coverage are additional important considerations for all genomic studies [18].
Statistical approaches for controlling for multiple comparisons are also common to the three areas of discussion in this Chapter. The most conservative approach is the Bonferroni correction in which case the p value is multiplied by the number of comparisons that are performed. The assumption for this method is that each association test is independent of all other tests, an assumption that is generally untrue in the biological setting as there are correlations in genetic variants, epigenetic marks, and gene expression across the genome. An alternative that is often used is the false discovery rate (FDR) correction, developed by Benjamini and Hochberg, in which case FDR correction is made for the number of expected false discoveries, providing an estimate of the number of true results among those called significant [19]. The final approach that is sometimes used is permutation-based adjustment for multiple comparisons. This computationally intensive approach generates an empirical distribution of the test statistic based on a large number (1000 or more) random assignments of the phenotype to the genome-wide profile that is used to asses significance by comparing minimal p value from the real data to that distribution [17].
Genome
The human genome sequence provides the underlying code for human biology. Our genome is composed of 3 billion base pairs and about 20,687 protein-coding genes. Protein-coding regions comprise 1.2 % of the genome and are referred to as the exome (collection of exons). The remainder of the genome is involved in regulation of the protein-coding genes [20–22]. Variations in the DNA sequence are important susceptibility factors for complex common diseases and syndromes such as ALI.
Common Variants
The main focus of complex disease genetics for the past 10–15 years has been the identification of common genetic risk variants (allele frequencies >5 %) [23]. The basis for this search is the Common Disease Common Variant (CDCV) hypothesis, which assumes that a relatively small number of ancient common risk alleles exist that each confer small to moderate risks of disease. The CDCV hypothesis was testable in this timeframe in a number of complex diseases due to several practical considerations: [1] the high frequency of putative risk alleles, allowing for cost-effective analysis by genotyping as opposed to sequencing; [2] the extensive linkage disequilibrium (LD, correlation) [24] between common variants in the human genome, which allows for genotyping only a small number of markers (LD tags) and indirect assessment of other common variants by LD mapping; and [3] the development of high-throughput genotyping arrays, which have allowed Genome-Wide Association (GWA) studies. Consequently more than 2000 GWA studies exploring the role of common variants in several hundred complex diseases or phenotypes have been conducted to date [25].
Study Design
GWA study can be of a case-control design of unrelated individuals with the disease phenotype of interest (cases) and unaffected individuals (controls) or of a quantitative phenotype [26]. The key feature of these studies is that they are based on association analysis and therefore require unrelated individuals (as opposed to genetic linkage studies in which families are required).
Technologies
Dense genotyping arrays containing hundreds of thousands to millions of markers have been developed for GWA studies. Two major commercial sources of genotyping arrays are Illumina and Affymetrix and they offer a variety of specific array platforms that should be chosen based on study population and hypothesis of the project. Selection of markers that are included on the genotyping arrays has relied largely of HapMap [27] and more recently 1000 Genome [28] projects. Specific content has been developed to provide a variety of array platforms that range from basic coverage to make large-scale projects affordable (OmniExpress Bead Chip, for example) to providing the most comprehensive coverage across multiple populations (Omni2.5 Bead Chip for example that was designed using 1000 Genome data).
Analysis
Analysis of common genetic variants is performed in three steps: [1] testing for departures from Hardy–Weinberg Equilibrium (HWE) proportions; [2] estimate of variant–variant LD to assess the genetic structure of the cohort [24]; and [3] association analysis under a specific genetic model (additive, recessive, or dominant) via logistic regression. Logistic regression models usually include demographic covariates such as age and gender. Instead of using basic race/ethnicity categories from self-reports, principal components (PCs) from the genome-wide genetic variant data are also included in the regression model. This is done because even among a sample of a single racial/ethnic group such as nonhispanic white individuals, consideration of the effects of population stratification is very important. Significance level in GWA studies is most commonly assessed using the concept of genome-wide significance, which uses an estimated number of independent genomic regions based on the distribution of LD in the genome for a specific population; for European populations, this is approximately p < 5 × 10−8 [17].
One of the fundamental concepts in the analysis of GWA studies is that of meta-analysis. In this approach, findings from multiple studies can be combined to increase the power of identification of significant genetic variants. An essential principle in meta-analysis is that all studies included examined the same hypothesis and that the original analysis was performed in an almost identical fashion [29]; when this is not possible, statistical approaches to assess heterogeneity of analysis methods are used [26]. Software package METAL is often used for meta-analysis of GWA studies [30] and detailed protocols for this type of study have been published [31].
Imputation analysis of genotype data is often performed in GWA studies for two reasons: [1] to identify additional genetic risk loci, and [2] to provide information on the same set of variants and thus facilitate meta-analysis. In this approach, a reference panel such as Hapmap or 1000 Genome data are used in conjunction with genotype data to infer genotypes that were not directly measured on the genotyping array using haplotypes (groups of variants that are inherited together, or are in high LD, in a specific population). The most important consideration in imputation is to use the reference population that is best matched to the study cohort. In practice, study sample haplotypes may match multiple reference haplotype and imputation assigns probability of the presence of specific allele(s). This analysis is performed using software such as IMPUTE [32] or MaCH [33].
While tools for functional annotation of genetic variants can be applied to most significant hits from the GWAS analysis, additional fine mapping by denser genotyping or sequencing is generally required to identify variants that are likely to have functional consequences. Functional annotation strategies are discussed in the next section.
Rare Genetic Variants
While GWA studies have provided a wealth of information on the genetic basis of common complex diseases, they have generally explained a small portion of disease heritability even after judiciously designed GWA studies screening >1 million markers in large groups of cases and controls have been carried out. The “missing heritability” problem has elicited interest in the potential role of rare variants in complex disease. Under the Common Disease Rare Variant (CDRV) hypothesis, any given risk gene or locus is characterized by high allelic heterogeneity and these risk loci contain multiple rare independent risk alleles across the population each with moderate to high penetrance. As a consequence of expected allelic heterogeneity, sequencing rather than genotyping is required for exploration of the CDRV hypothesis in complex diseases. Furthermore, screening of these rare alleles is not amenable to LD tagging approaches as they are poorly tagged by common variants and individual rare variants are expected to occur on different haplotypes (a group of variants that are inherited together). The emergence of massively parallel sequencing technologies has dramatically reduced the time and cost of study population sequencing, setting the stage for exploration of the CDRV hypothesis in complex diseases. Different models of the genetic basis of complex traits are discussed in detail elsewhere [34].
Study Design
Whole genome, whole exome, and targeted sequencing projects are mostly designed to identify rare genetic variants but they will also capture information on common variation. Whole genome sequencing (WGS) studies capture variation in both coding and noncoding variants while whole exome sequencing (WES) studies are focused on coding variants (although newer target enrichment strategies capture UTRs and some promoter and intronic sequence). WES is ~10 fold less expensive than WGS thus allowing for a larger number of samples to be profiled, and the data produced are less complex to analyze. Targeted resequencing studies are well suited for following up on GWAS or linkage loci and are generally designed to capture the entire locus, including both coding and noncoding regions. Loci with previously associated common variants through GWAS can be fine mapped and are more likely to contain functional rare variants and therefore may be the most fruitful application of next generation sequencing to complex disease. It was initially proposed that two affordable strategies for identification of disease-causing variants were [1] sequencing of affected individuals in a pedigree followed by genotyping of candidate variants to demonstrate co-segregation with disease in the family and [2] extreme-trait sequencing of a small number of individuals at the tails of the trait distribution followed by targeted sequencing or genotyping in a larger cohort [35, 36]. As the cost of sequencing has decreased, other study designs have been adopted. Statistical considerations for the design of rare variant association studies are discussed in detail elsewhere [37].
Technologies
The first sequences of the human genome published a decade ago [38, 39] were accomplished using automated Sanger sequencing with dideoxy chain termination [40] at a cost of approximately $2.7 billion to produce a draft sequence of the human genome (http://www.genome.gov/11006943). In contrast, today a human genome can be sequenced at 20× coverage for roughly $2500 using next generation sequencing (NGS) technology. NGS refers to a group of strategies that rely on a combination of template preparation, sequencing and imaging, and genome alignment and assembly methods, producing gigabases (GB) of sequence data per run in the form of short reads [41]. The Illumina sequencing platform is the most commonly used at the present time and its read length is currently capped at 2 × 125 bp. Single molecule technologies such as from Pacific Biosciences do not require the clonal amplification of molecules to be sequenced, but rather a single DNA molecule is sequenced by synthesis using a DNA polymerase. Single molecule sequencing technologies promise a much simpler sample preparation and offer longer read lengths but generally have lower accuracy than short-read sequencers, which makes them less desirable for rare variant studies. However, their long read lengths are useful in the regions of the genome that are difficult to assemble using short reads, with highly repetitive genomic regions being the best example. A combination of short and long read length technologies provides the best solution in some cases. Single molecule technology is reviewed elsewhere [42].
Another important aspect of sequencing exomes or genomic regions of interest, such as those identified by GWA studies, is target capture. Large genomic regions are most efficiently and cost-effectively captured using hybridization-based approaches such as Agilent SureSelect and Nimblegen SeqCap while PCR-based approaches offered by Life Technologies AmpliSeq and Agilent Haloplex are more suited for capture of smaller regions [43].
Analysis
Four main steps in the analysis of sequencing data are [1] base calling and sequence assembly [2], variant detection [3], statistical analysis to identify significant associations, and [4] functional analysis of the identified variants. Several recent reviews have detailed discussion of these steps [36, 44, 45] with the most important points briefly summarized here. Data analysis workflow begins with the base calling and alignment of sequence data to the reference genome [46]. Base-calling procedures generate per-base quality values (QVs) that are typically converted to Phred-like quality score [47]. Most alignment software provides run metrics that allow the user to assess quality of sequence data; these include number of raw reads, number of mapped reads, number of unique reads, sequence coverage, and coverage for and percent of “on target” reads for targeted approached [44]. The quality controls metrics allow the researchers to determine potential experimental and alignment biases and remove low quality and poorly mapping reads from further analysis.
The high quality, aligned reads are then analyzed to identify DNA sequence variants, most commonly single nucleotide variants (SNVs); information on structural variants (SVs) and copy number variants (CNVs) can also be obtained. Multiple software options exist for variant calling with both those that perform variant calls in individual samples and those that use multiple samples to call variants [45, 47]; commonly used pipelines include GATK [48] and SAMTools [49], among others. Single marker statistical analysis of common variants (>5 %MAF) from sequence data is identical to that used for GWA studies. On the other hand, most study populations will be underpowered to conduct single marker tests of association for rare variants (0.1–1 % range of MAF) despite the expectation of high effect sizes for rare risk alleles (relative risks ~2.5–5.0). The general approach taken is therefore to test for association between disease status and the accumulation of rare variants across the risk locus or gene units rather than with any single variant. Three main classes of collapsing tests are [1] tests that use group summary statistics on variant frequencies in cases and controls; [2] those that test for similarity in unique DNA sequences in different individuals; and [3] regression models that test collapsed sets of variants (and other variables) as predictors of the phenotype [36]. Many of the features of the different approaches are combined in a single software SKAT-O, where O refers to optimal [50] that is commonly used in these analyses.
Following association testing, identified variants are analyzed for functional consequences. Algorithms that predict deleteriousness of protein-coding variants such as SIFT or PolyPhen are used to prioritize nonsense and frameshift mutations because they result in loss of protein function [51, 52]. SIFT has been incorporated into ANNOVAR pipeline [53] while PolyPhen is a part of the SeattleSeq pipeline (http://snp.gs.washington.edu/SeattleSeqAnnotation) as well as the PLINK/SEQ suite (http://atgu.mgh.harvard.edu/plinkseq/) for comprehensive functional annotation of variants. Methods for prioritizing noncoding variants based on nucleotide sequence conservation are also being utilized in large-scale sequencing studies; one commonly used of the many available algorithms (listed in Ref. [54]) is the Genomic Evolutionary Rate Profiling (GERP) algorithm [55]. These algorithms use comparative genomics, generally limited to mammalian species as nucleotide sequence is less conserved than protein sequence, to estimate nucleotide-level evolutionary constraints in genomic sequence alignments and assign conservation scores. Higher conservation scores are indicative of a more likely regulatory function and can be used to prioritize noncoding variants for further studies. A recently developed Combined Annotation–Dependent Depletion (CADD) method integrates many diverse measures of functional relevance such as deleteriousness, conservation, and other scored into a single measure (C score) for each variant that can be used to objectively prioritize variants [56]. An alternative to post hoc analysis of variants in associated genes/loci is to incorporate functional information into the test and stratify or weigh rare alleles by functional significance. A number of tests allow for inclusion of prediction scores in test statistics; PLINK/SEQ, for example, includes previously computed PolyPhen scores [57].
Targeted Methods for Validation
The choice of the platform for internal or technical validation of variants identified by genome-wide technologies is guided by the frequency of the variant and number of samples. The most cost-effective way to validate variants with low frequencies is usually to directly sequence the region that contains multiple rare variants in different individuals. This is achieved by Sanger sequencing or PCR-based next generation strategies such as the IonTorrent Ampliseq platform. On the other hand, variants with higher frequencies can be genotyped at a lower cost than sequencing using targeted genotyping panels; Illumina, Sequenom, and Fluidigm, to name a few, have solutions for custom genotyping panels.
Progress to Date in ALI
Because of the nature of ALI, specifically the fact that it does not occur spontaneously but as an outcome of severe illness, no family pedigrees exist and therefore early linkage studies that identified some regions of the genome of interest in other lung diseases such as asthma could not be performed in ALI. Despite this, it is thought that genetic factors influence individual’s predisposition to development of severe illness, ALI/ARDS, and response to treatment in a multistage genetic risk model that has been proposed [4]. This is further supported by the established role of genetic variation in the control of host response to stimulation with PAMPs and other innate immune stimuli [58–60].
Early studies of association of SNPs in candidate genes in inflammatory and other relevant pathways with ALI phenotypes are summarized in [61]. More recent candidate gene studies have identified association of SNPs in the elafin or peptidase inhibitor 3 (PI3) gene with increased risk of ARDS [62], ANGPT2 genetic variant with trauma-associated ALI [63], IL1RN coding variant with lower risk of ARDS [64] as well as SNPs in an adiponectin-like gene ADIPOQ [65] and colony stimulating factor 2 (CSF2) [66] with higher mortality, among others. Because of the prominent role of platelet levels in the pathophysiology of ALI/ARDS, another targeted study examined association of genetic variants in five loci previously associated with platelet levels through a meta-analysis with ARDS outcomes. This work confirmed the importance of LRRC16A in platelet formation and suggested a role for it in ARDS pathophysiology [67]. Importantly, distinct genetic risk factors have been identified as associated with ARDS caused by direct versus indirect injury to the lung [68], demonstrating that study of more homogeneous clinical phenotypes is critical in genetic studies.
Genomic assessments of the effect of genetic variants of ALI phenotypes are just emerging. The first GWAS of 600 ALI cases and 2266 controls followed by replication in 212 cases and 283 controls identified 159 significant SNPs (p < 0.05 for replication) associated with risk of ALI, providing support for further evaluation of genetic variation in this syndrome. Similarly, an exome sequencing study of 96 ARDS cases compared to 1000 Genome control data identified 89 SNPs associated with susceptibility to ARDS, with a few of these variants also associated with severity as assessed by the APACHE II score as well as mortality [62].
Transcriptome
The transcriptome is the collection of all the RNA molecules, or transcripts, present in a cell. DNA is transcribed by RNA polymerase to create complementary RNA strands, which in turn are spliced to remove introns, producing mature transcripts that contain only exons, which are translated into protein. While only a small percentage of the human transcriptome is translated into proteins, a number of proteins have different isoforms that stem from alternatively spliced transcripts. The remaining transcriptome is largely comprised of a number of noncoding RNAs that are involved in regulation of gene expression; these include thousands of pseudogenes [69], circular RNAs [70], long noncoding RNAs [71], and small noncoding RNAs [71]. The most well studied group of noncoding RNAs are microRNAs (miRNAs). They control gene expression by binding to the 3′ untranslated regions (UTRs) of messenger RNA (mRNA), which leads to either mRNA degradation or inhibition of protein translation. Regulation of gene expression by miRNAs is complex in that many miRNAs can regulate expression of a single gene and, similarly, each miRNA can regulate a large number of genes. Other noncoding RNAs are reviewed in detail elsewhere [71].
Study Design
Study design for genome-wide assessment of coding and noncoding RNA is in principle the same with two important considerations: power to detect association with the outcome variable of interest, and potential confounders including covariates and batch effects. Power and covariates were discussed in the general design section. Batch effects are broadly classified as known (amount of labeled RNA, date of library preparation or hybridization, position on the array, lane on the sequencing run, etc.) and unknown batch effects. While statistical approaches for correcting for known and unknown batch effects are available and will be discussed in the analysis section, care should be taken during study design to minimize the effect of known batch effects. This is done by assigning samples from each experimental group to each set or batch of labeling reactions, library preparation, hybridization, sequencing lane, etc., for small sample sizes or randomizing across batches for larger sample sizes [72].
Technologies
Genomic profiling of gene expression levels in lung disease began as early as in other diseases and used both homemade cDNA arrays and some of the first commercially available arrays that both suffered from many technical issues [73–75]. However, substantial improvements in both laboratory and analytical aspects of microarray-based analysis of gene expression have occurred [76, 77] and genomic analysis of gene expression on microarrays has reached maturity to become a fairly standard approach. Major providers of gene expression arrays are Affymetrix, Agilent, and Illumina and they all use one-color assays at this time (some of the older platforms used two colors).
RNA sequencing-based approaches were introduced much more recently and are not as mature as microarrays [78]. While protocols for library preparation and sequencing are fairly standard at this time, best practices for data analysis of RNA-seq data are still under development (and will be discussed in the next section). Sequencing of polyA-enriched libraries at relatively low coverage is the most affordable form of RNA-seq and gives information on coding transcripts that is comparable to microarrays. Sequencing of the same library at higher coverage results in an expanded detection limit for more rare transcripts and data can also be analyzed for alternative splicing. On the other hand, sequencing of ribosomal RNA-depleted libraries provides information on coding and noncoding RNAs. One extension of this protocol is dual RNA-seq for capturing transcriptome information on both the host and pathogens [79]. Small RNA-seq library preparation protocols are required to capture mature small RNA species such as miRNAs. An important note is that standard RNA extraction protocols do not always capture small RNA and therefore care must be taken in this step to capture them if they are of interest to the study.
The most exciting advance in RNA-seq technology in the past few years has been the extension to single cell analysis of the transcriptome. In this approach, technologies such as that offered by Fluidigm are used to isolate single cells, often following flow cytometry or other methods for selection of pure cell populations, and create cDNA in 96-well plate format that can be used for either standard quantitative PCR or RNA-seq library preparation. While the cost of this type of experiment is high due to the need to sequence large numbers of single cells, it is somewhat offset by the fact that lower sequencing coverage is needed for single cells. This technology has recently been used to provide a greater molecular understanding of lung development and cell lineages in the lung [80] and is a promising approach to understand molecular underpinnings of lung diseases such as ALI.
Analysis
The main steps in the analysis of transcriptome data are: [1] alignment of sequence data in the case of RNA-seq [2] normalization and scaling [3], assessment of batch effects, and [4] identification of differentially expressed genes or noncoding RNAs. The output of a microarray experiment is background-subtracted set of intensities for all probes on the array. The output of RNA-seq data are short read sequences that first need to be aligned to the genome with gapped sequence aligners such as TopHat [81] and quantified relative to known genes in the genome using software such as BEDTools [82]. More detailed consideration of sequence alignment and read quantification for transcriptome analysis is presented in [83, 84]. Both array and RNA-seq data need to be normalized and scaled so that across-sample comparisons can be made. The most commonly used normalization approach is quantile normalization such as robust multi-array average (RMA) [85] or similar approaches tailored for RNA-seq data [86].
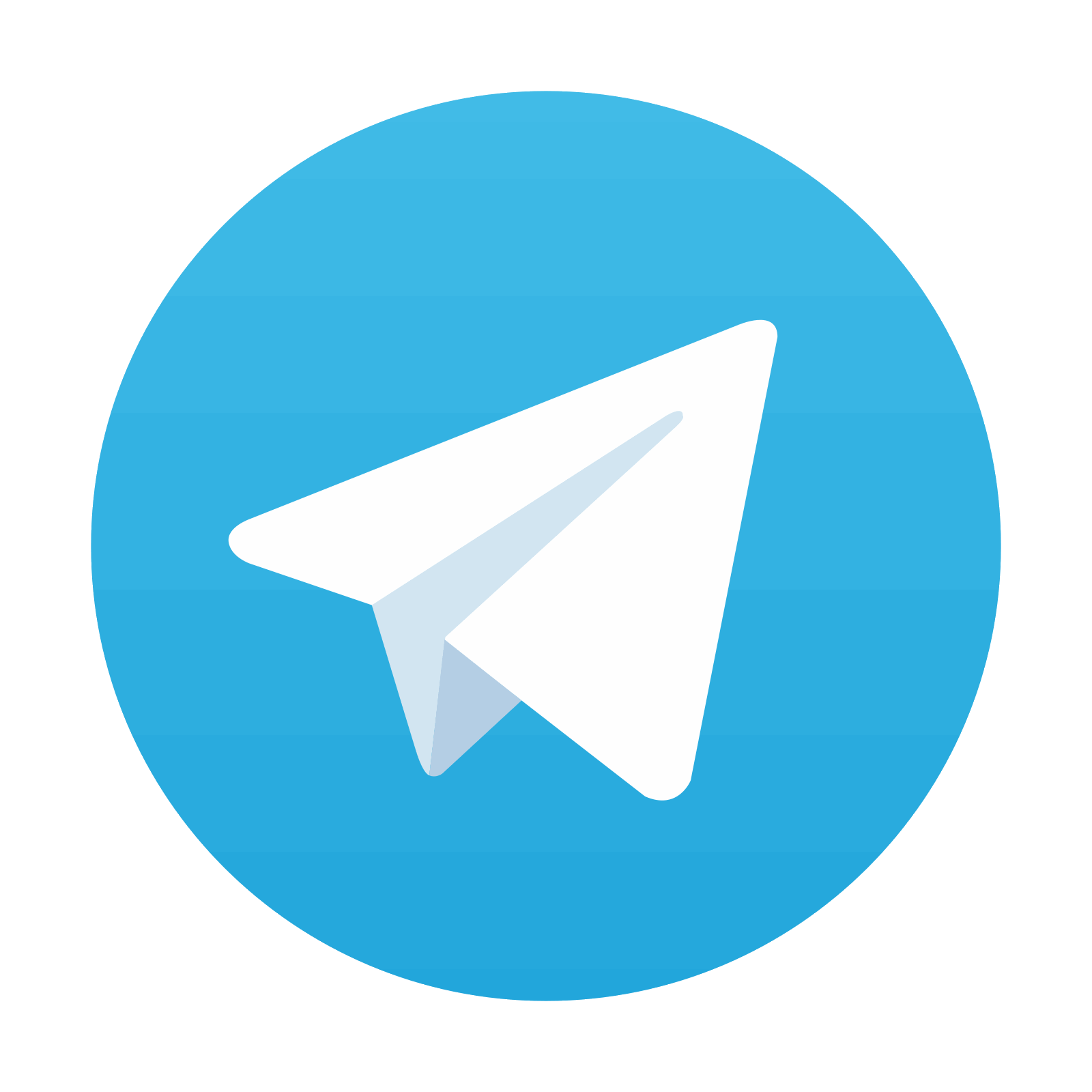
Stay updated, free articles. Join our Telegram channel

Full access? Get Clinical Tree
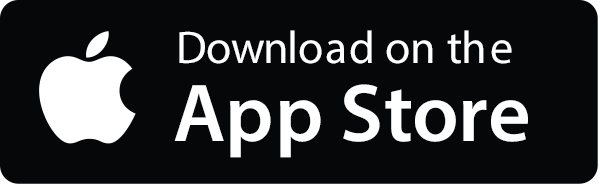
