, Xiaoyi Jiang1, Mohammad Dawood2 and Klaus P. Schäfers2
(1)
Department of Mathematics and Computer Science, University of Münster, Münster, Germany
(2)
European Institute for Molecular Imaging, University of Münster, Münster, Germany
We have discussed methods for PET motion estimation and motion correction in the previous chapters. In particular, a priori knowledge about the image generation process, in terms of mass-preservation, was utilized to improve the motion estimates needed for the advanced correction pipelines. This chapter gives an outlook of potential improvements and modifications of the presented methods and future advances of PET motion correction in general. The proposed improvements of the process of motion estimation and motion correction are given by, e.g., extracting motion from accompanying MRI scans instead of the PET data itself. Further, we briefly discuss future developments of PET motion correction in clinical applications.
4.1 Future Work of Vampire and MPOF
The methods described in Chaps. 2 and 3 describe the general proceeding for mass-preserving motion estimation and motion correction in PET. In the following, we discuss some potential improvements of the described proceeding. These modifications are:
Using an improved gating technique,
Extension to a spatial-temporal approach,
Application to dynamic PET data.
Further improvements by parameter optimization is not discussed in great detail. We just provide the following list of starting points. It is desirable to determine the
4.1.1 Gating
The gating schemes for Vampire used in [51] and MPOF in [37] use an equidistant time-based gating for the cardiac cycle. A more sophisticated cardiac gating with time varying cardiac gates [127] or with grouping of cardiac phases [74] could better resolve the cardiac phases (in particular regarding the systole) and should be considered in future work.
For respiratory motion, the proposed amplitude-based gating has the advantage of (almost) equal statistics in each gate, which manifests itself in a comparable noise level in the reconstructed images. This allows us to choose a constant set of regularization parameters for all motion estimation tasks. The downside of this gating scheme is the varying motion blur in different gates. Infrequent motion states feature an increased blurring induced by motion. A phase-based gating scheme would allow to create gates with equal motion blur in each gate, however, with the effect of different SNRs in the gates. The optimal trade-off between remaining motion and statistics per gate should thus be explored. This is directly related to the analysis of the optimal number of dual gates, which is not addressed in this work but interesting to analyze, cf. [36].
4.1.2 Spatial-Temporal Approach
Respiratory and cardiac motion is cyclic and smooth over time. It should thus be taken advantage of this information. The approaches described in Chap. 2 estimate the motion between each motion phase and the reference phase independently. Modifications could be an estimation of motion between each phase and its subsequent phase to keep the expected motion vectors small [73]. This is particularly interesting for optical flow methods due to the Taylor linearization. Another extension is to explore the cyclic nature of motion [83]. As hyperelastic regularization allows for large motion, this is not essential in the case of Vampire. Still, a global smoothness constraint which accounts for smooth transitions and cyclicality should be investigated to further improve the robustness of motion estimation towards noise.
Klein et al. [73] also propose a tissue-dependent regularization in their work by modeling the incompressibility of myocardial tissue. If different tissue compartment are given, e.g., by applying a prior segmentation, non-uniform regularization could be applied to incorporate further a priori knowledge about tissue-dependent motion behavior.
4.1.3 Dynamic PET
The applications of motion estimation and correction discussed in this book assume that the tracer is already accumulated in the tissue. We expect no considerable tracer dynamics between different motion phases. However, dynamic PET scans are also acquired in daily clinical routine and should thus also be corrected for motion. Consequently, simultaneous estimation of tracer kinetics and motion in dynamic PET scans is an important and interesting field of application.
Motion estimation in dynamic PET brings up some challenges, which are presumably the reason why motion correction in dynamic PET is more or less neglected so far. We have already seen that the main assumption of most motion estimation techniques is that corresponding points in different images have approximately the same intensity. In the case of dynamic PET we cannot expect similar intensities at corresponding points as the uptake of radioactivity varies for each individual organ over time according to the tracer dynamics. As in the case of mass-preservation, this deviation from the assumption of similar intensities needs to be modeled explicitly. We thus have to model the tracer dynamics, e.g., using kinetic modeling [26, 120], in addition to the motion estimation. A possible sequential correction scheme could be:
1.
Respiratory gating using the whole data set (tracer dynamics are averaged out).
2.
Estimation of respiratory motion (with mass-preservation).
3.
Kinetic modeling using the whole data set (respiratory motion corrected).
4.
Cardiac gating of the whole data set (respiratory motion corrected and compensated for tracer dynamics).
5.
Estimation of cardiac motion (with mass-preservation).
6.
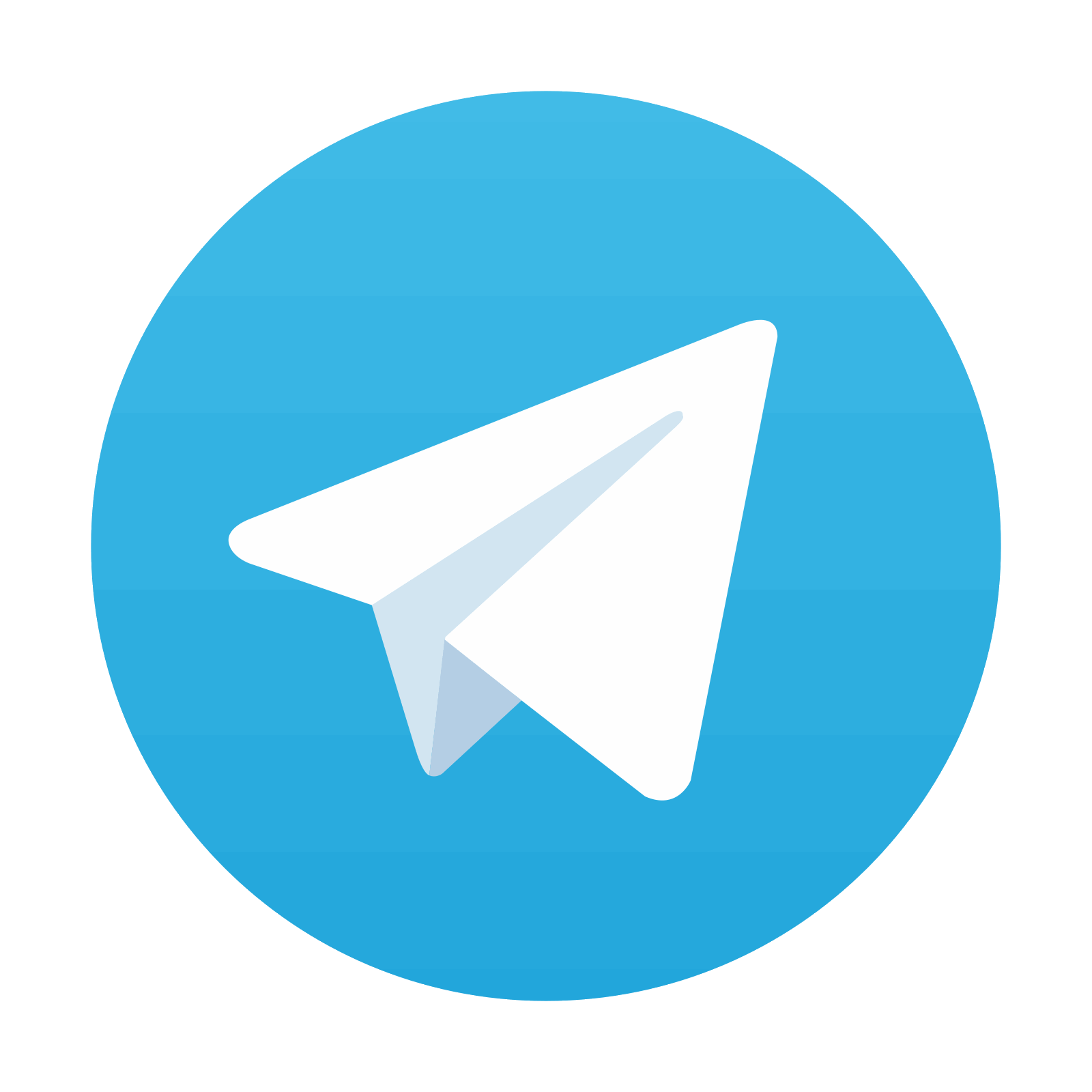
Reconstruction of the final image which is corrected for respiratory and cardiac motion and tracer dynamics.
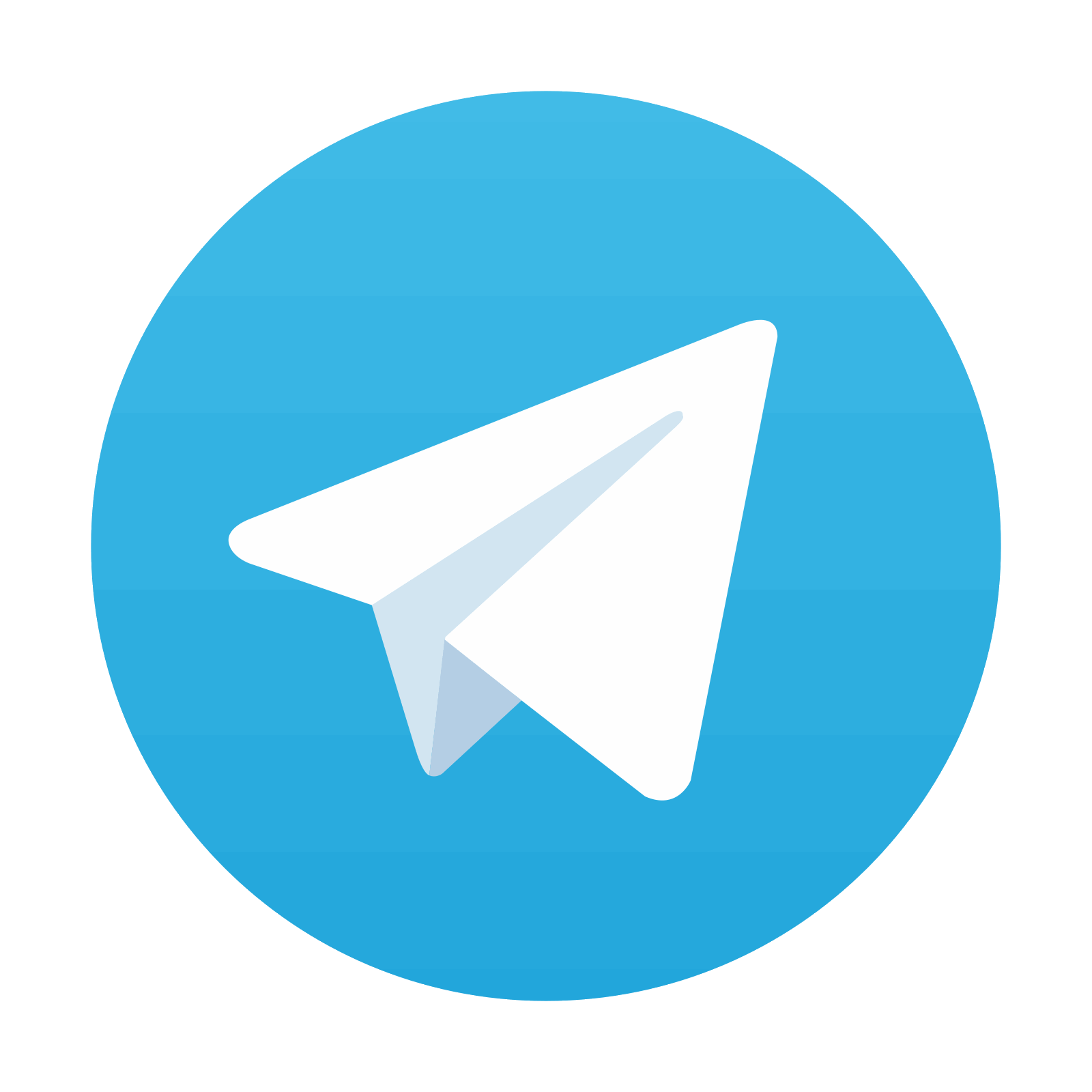
Stay updated, free articles. Join our Telegram channel

Full access? Get Clinical Tree
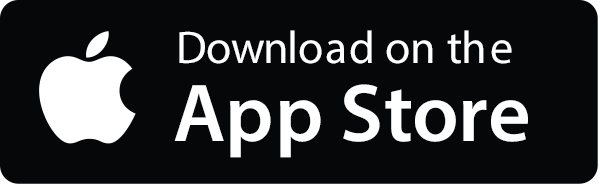

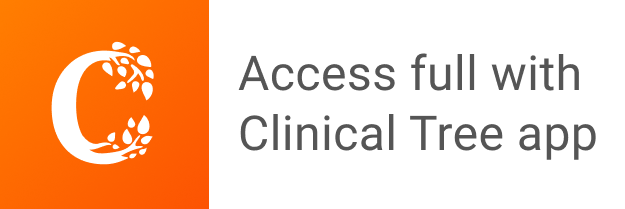