Given the role of comorbid conditions in the pathophysiology of HFpEF, we aimed to identify and rank the importance of comorbid conditions associated with post-hospitalization outcomes of older adults hospitalized for HFpEF. We examined data from 4,605 Medicare beneficiaries hospitalized in 2007–2014 for HFpEF based on ICD-9-CM codes for acute diastolic heart failure (428.31 or 428.33). To identify characteristics with high importance for prediction of mortality, all-cause rehospitalization, rehospitalization for heart failure, and composite outcome of mortality or all-cause rehospitalization up to 1 year, we developed boosted decision tree ensembles for each outcome, separately. For interpretability, we estimated hazard ratios (HRs) and 95% confidence intervals (CI) using Cox proportional hazards models. Age and frailty were the most important characteristics for prediction of mortality. Frailty was the most important characteristic for prediction of rehospitalization, rehospitalization for heart failure, and the composite outcome of mortality or all-cause rehospitalization. In Cox proportional hazards models, a 1-SD higher frailty score (0.1 on theoretical range of 0 to 1) was associated with a HR of 1.27 (1.06 to 1.52) for mortality, 1.16 (1.07 to 1.25) for all-cause rehospitalization, 1.24 (1.14 to 1.35) for HF rehospitalization, and 1.15 (1.07 to 1.25) for the composite outcome of mortality or all-cause rehospitalization. In conclusion, frailty is an important predictor of mortality and rehospitalization in adults aged ≥66 years with HFpEF.
Heart failure with preserved ejection fraction (HFpEF) comprises 50% of heart failure across the United States and is a condition that can lead to dyspnea, peripheral edema, and fatigue despite a preserved, or normal, left ventricular ejection fraction. Although many clinical trials have been conducted over the past decade, none have identified pharmacologic agents that improve outcomes in patients with HFpEF. The leading hypothesis for these failed clinical trials is that HFpEF is a highly heterogeneous condition, with a diverse set of abnormalities that span across cardiovascular and noncardiovascular systems. In response to this heterogeneity, the field has embraced a “phenotype-matching” approach to developing and studying therapeutics for HFpEF, whereby treatment can be personalized based on the presence (or absence) of specific clinical characteristics. By identifying and describing unique phenotypes within HFpEF, pharmacologic therapeutics that target unique features of each phenotype can be developed, and ultimately improve outcomes in this complex population. To date, there are many HFpEF phenotypes that have been described in the literature—some based on physiologic parameters, and some based on empiric clinical impression. Given the role of comorbid conditions in the pathophysiology of HFpEF and their association with adverse outcomes, there is strong rationale to incorporate comorbidity into the phenotyping of HFpEF. We accordingly aimed to identify and rank the importance of common comorbid conditions that predict mortality and rehospitalization among older adults hospitalized for HFpEF.
Methods
The study population included beneficiaries aged 66 to 110 years in the United States Medicare program hospitalized for HFpEF. Medicare provides medical and prescription drug insurance for older adults, as well as individuals with disabilities and end-stage renal disease. For this study, we first identified individuals in the national Medicare 5% sample who had a hospitalization between January 1, 2007 and December 31, 2014 with a discharge diagnosis of heart failure with diastolic dysfunction (International Classification of Diseases, Ninth Revision [ICD-9] 428.31 or 428.33, acute diastolic heart failure). This definition has been used in previous studies, and individuals identified using this approach have characteristics similar to populations with HFpEF studied in randomized controlled trials and population-based studies. We included hospitalizations that were at least overnight and up to 30 days in duration. Similar to previous studies examining data from Medicare, we included hospitalizations for beneficiaries that live in the United States and have continuous fee-for-service medical and prescription drug coverage (Medicare Part A, B, and D) in the 365 days previous to the HFpEF hospitalization, have consistent demographic data, and were hospitalized at an institution with data reported in the 2014 Medicare Hospital Compare and American Hospital Association survey datasets. We selected the first eligible hospitalization for each individual. The study sample included 4,605 Medicare beneficiaries with a hospitalization for HFpEF ( Figure 1 ). This study was reviewed by the University of Alabama at Birmingham Institutional Review Board and Center for Medicare and Medicaid Services Privacy Board.

We ascertained beneficiary demographics (age, sex, race/ethnicity, low income status based on eligibility for Medicaid or Medicare Part D subsidies, and region of residence) from Medicare enrollment information. We examined comorbid conditions and geriatric conditions based on Medicare claims during the 365 days before the HFpEF hospitalizations. We opted to examine several comorbid conditions (coronary heart disease, stroke, peripheral vascular disease, diabetes, obesity, anemia, sleep apnea, hypertension, dyslipidemia, atrial fibrillation, chronic obstructive pulmonary disease, chronic kidney disease, cancer, liver disease, rheumatologic disease, previous diagnosis of heart failure, tobacco use, osteoporosis, and depression) given their hypothesized role in the pathophysiology of HFpEF ; and geriatric conditions given the link between aging processes and HFpEF. For geriatric conditions, we examined frailty, dementia, and history of falls. Frailty was defined based on claims using the deficit accumulation approach with scores on a continuum between 0 and 1—this approach has been validated in Medicare. We also examined previous healthcare utilization (nursing home residence using a previously validated algorithm, number of outpatient visits, cardiologist care, hospitalization, and skilled nursing facility stay) using Medicare claims during the 365 days before the index HFpEF hospitalization. We used Medicare claims data to identify characteristics of the hospitalization which included calendar year, intensive care unit stay, and length of stay. We used the American Hospital Association 2014 survey to determine hospital ownership, size, region, urban/rural location, teaching status, and presence of a cardiac critical care unit. Lastly, we used the Center for Medicare and Medicaid Services 2014 Hospital Compare datasets to determine hospital-level heart failure 30-day readmission and mortality rates, overall rating, and patient star rating. Further details are provided in the Supplemental Methods.
Using Medicare claims, beneficiaries were followed from hospital discharge for the index HFpEF hospitalization for up to 1 year; outcomes collected included mortality, all-cause rehospitalization, rehospitalization for heart failure, and the composite outcome of all-cause rehospitalization or mortality. Mortality was based on Medicare enrollment information; rehospitalization was defined as a claim for any inpatient care; and rehospitalization for heart failure was defined as a claim for inpatient care with a primary discharge diagnosis of heart failure. Follow-up time for beneficiaries was censored if they moved out of the United States or lost Medicare fee-for-service coverage.
We first calculated means and standard deviations or number and percentages for continuous and categorical variables, respectively, to describe beneficiary and hospital characteristics. To identify the most important characteristics associated with mortality, all-cause rehospitalization, rehospitalization for heart failure, and the composite outcome of mortality or all-cause rehospitalization, we developed ensembles of boosted decision trees, a machine learning algorithm that approximates the importance of predictors based on how much they contribute to the algorithm’s prediction function, using the extreme gradient boosting algorithm, which is implemented in the xgboost R package (version 0.82.1; https://CRAN.R-project.org/package=xgboost ). Ensembles of boosted decision trees combine predictions across multiple decision trees, where each tree is developed to correct errors made by its predecessor, resulting in predictions that generalize to external populations more accurately than those from a single decision tree. These models can incorporate non-linear associations and complex interactions between characteristics. Parameters were tuned by repeated (20 times) 10-fold cross-validation, using the negative log-likelihood as the tuning criteria. Because of their high correlation with other included predictors, the variables hospital region, hospital star rating, hospital cardiac critical care unit, non-profit hospital, and hospital mortality rate were excluded from the modeling procedures. To rank the importance of the beneficiary and hospital characteristics for predicting outcomes following HFpEF hospitalization, we calculated the gain, which is defined as the degree to which the characteristics reduce the loss function when included in the decision tree. To describe the magnitude and direction of associations, we calculated hazard ratios (HR) and associated 95% confidence intervals (CI) using Cox proportional hazards models that included the variables with the highest gain values using the XGBoost algorithm. While the HR and CI have the benefit of interpretability, the characteristics associated with the largest gain in ensembles of boosted decision trees may not always be those with the narrowest CI in Cox proportional hazards models. There are two primary reasons for this. First, gain incorporates both the strength of the association between the characteristic and the outcome, and how commonly the characteristic is observed. Second, unlike the ensembles of boosted decision trees, the Cox models only examined linear associations and assumed no interaction between characteristics. Data management and analyses were conducted using SAS version 9.4 and R version 3.6.1.
Data needed to replicate these analyses are available from the Center for Medicare and Medicaid Services and the American Hospital Association. Statistical code is available from the authors.
Results
The average age of Medicare beneficiaries with a HFpEF hospitalization in this cohort was 80.3 years (SD 8.4 years), 70.7% were women, 84.6% were white, and 39.4% had low income as measured by eligibility for Medicare or Medicare Part D subsidies ( Table 1 ). Comorbidities were common; 73.6% had hypertension, 51.1% had coronary heart disease, 46.1% had atrial fibrillation, 45.0% had chronic kidney disease, 42.0% had anemia, and 40.9% had diabetes. Regarding geriatric conditions, the mean frailty score was 0.2 (SD 0.1) and 41.0% had a frailty score of at least 0.25 indicating presence of frailty; 12.8% had dementia, and 1.1% had a history of falls. The average length of stay was 7.3 days and more than a third (37.2%) of hospitalizations included time spent in an intensive care unit ( Table 2 ). Of the 4,605 beneficiaries included in this cohort, 11.6% (n = 536) experienced mortality, 53.9% (n = 2,484) experienced rehospitalization for any cause, 16.9% (n = 777) experienced rehospitalization for heart failure, and 56.1% (n = 2,582) experienced rehospitalization or mortality in the year following discharge.
N = 4,605 | |
---|---|
Variable | |
Age (years) mean (SD) | 80.3 (8.4) |
Women | 3255 (70.7%) |
Race/ethnicity | |
White | 3895 (84.6%) |
Black | 410 (8.9%) |
Asian | 107 (2.3%) |
Hispanic/Latino | 100 (2.2%) |
Other | 93 (2%) |
Low income based on Medicaid/Medicare subsidies eligibility | 1815 (39.4%) |
Comorbid conditions | |
Coronary heart disease | 2351 (51.1%) |
Stroke | 254 (5.5%) |
Peripheral vascular disease | 474 (10.3%) |
Diabetes | 1884 (40.9%) |
Obesity | 855 (18.6%) |
Anemia | 1932 (42%) |
Sleep apnea | 427 (9.3%) |
Hypertension | 3387 (73.6%) |
Dyslipidemia | 2639 (57.3%) |
Atrial fibrillation | 2124 (46.1%) |
Chronic obstructive pulmonary disease | 1779 (38.6%) |
Chronic kidney disease | 2073 (45%) |
Cancer | 859 (18.7%) |
Liver disease | 119 (2.6%) |
Rheumatologic disease | 244 (5.3%) |
Prior diagnosis of heart failure | 1675 (36.4%) |
Tobacco use | 1039 (22.6%) |
Osteoporosis | 547 (11.9%) |
Depression | 1379 (29.9%) |
Geriatric Conditions | |
Frailty score, mean (SD) | 0.2 (0.1) |
Dementia | 588 (12.8%) |
History of falls | 51 (1.1%) |
Region of residency | |
New England | 414 (9%) |
Middle Atlantic | 781 (17%) |
East North Central | 834 (18.1%) |
West North Central | 364 (7.9%) |
South Atlantic | 866 (18.8%) |
East South Central | 222 (4.8%) |
West South Central | 457 (9.9%) |
Mountain | 154 (3.3%) |
Pacific | 513 (11.1%) |
Healthcare utilization in the year prior to hospitalization | |
Nursing home residence | 378 (8.2%) |
Number of outpatient evaluations, mean (SD) | 12.3 (9.1) |
Hospitalization for any cause | 226 (4.9%) |
Cardiologist care | 1891 (41.1%) |
Skilled nursing facility stay | 1116 (24.2%) |
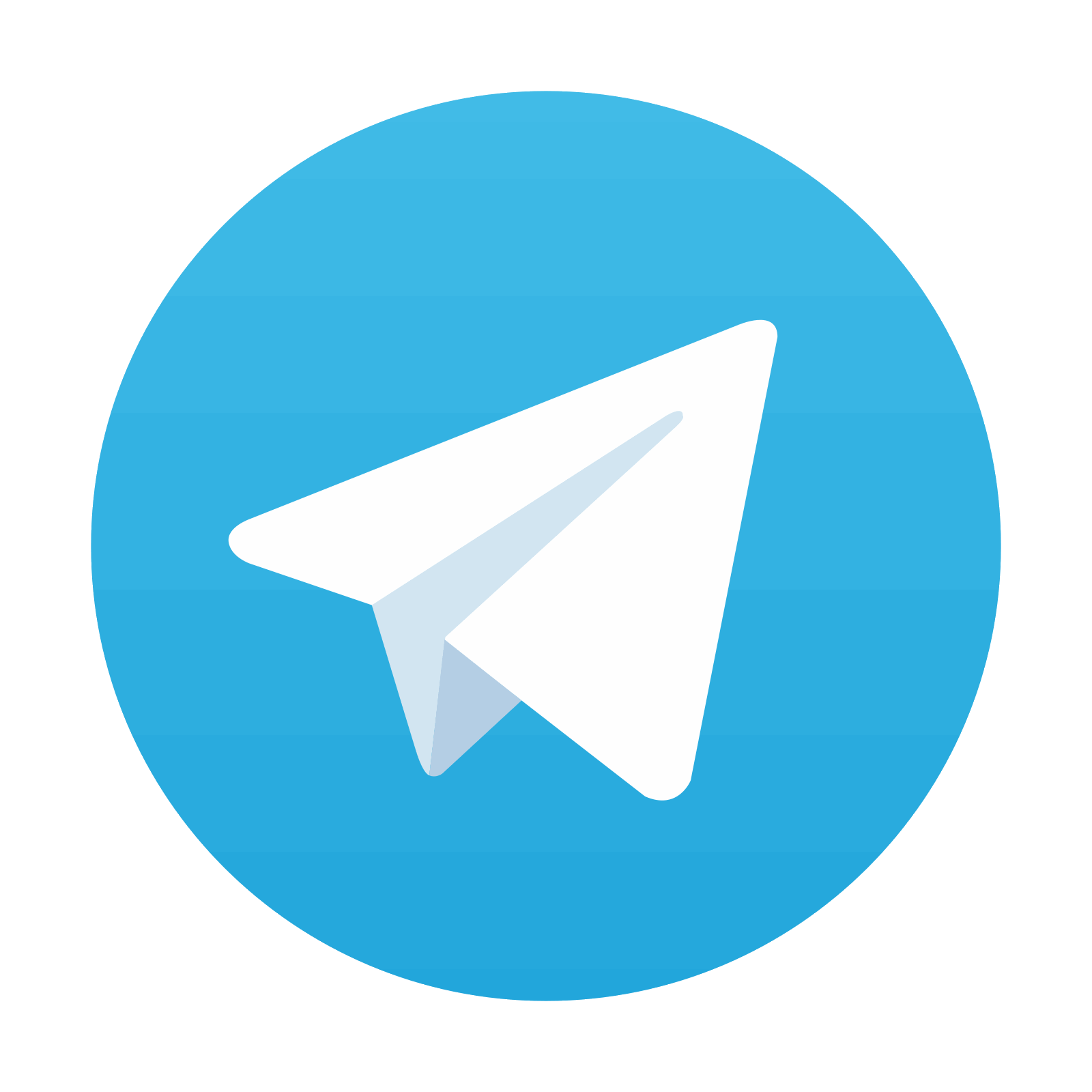
Stay updated, free articles. Join our Telegram channel

Full access? Get Clinical Tree
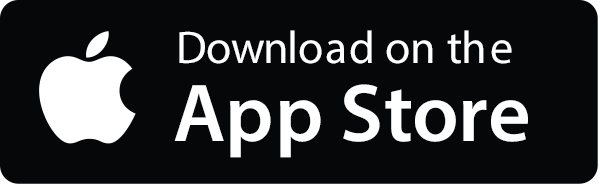

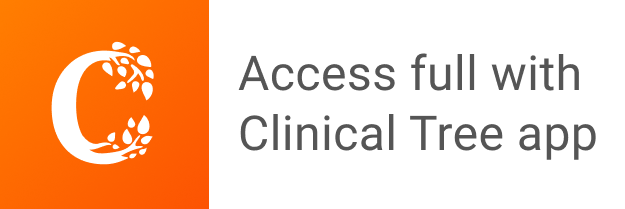