Background
A deep learning algorithm to detect low ejection fraction (EF) using routine 12-lead electrocardiogram (ECG) has recently been developed and validated. The algorithm was incorporated into the electronic health record (EHR) to automatically screen for low EF, encouraging clinicians to obtain a confirmatory transthoracic echocardiogram (TTE) for previously undiagnosed patients, thereby facilitating early diagnosis and treatment.
Objectives
To prospectively evaluate a novel artificial intelligence (AI) screening tool for detecting low EF in primary care practices.
Design
The EAGLE trial is a pragmatic two-arm cluster randomized trial ( NCT04000087 ) that will randomize >100 clinical teams (i.e., clusters) to either intervention (access to the new AI screening tool) or control (usual care) at 48 primary care practices across Minnesota and Wisconsin. The trial is expected to involve approximately 400 clinicians and 20,000 patients. The primary endpoint is newly discovered EF ≤50%. Eligible patients will include adults who undergo ECG for any reason and have not been previously diagnosed with low EF. Data will be pulled from the EHR, and no contact will be made with patients. A positive deviance qualitative study and a post-implementation survey will be conducted among select clinicians to identify facilitators and barriers to using the new screening report.
Summary
This trial will examine the effectiveness of the AI-enabled ECG for detection of asymptomatic low EF in routine primary care practices and will be among the first to prospectively evaluate the value of AI in real-world practice. Its findings will inform future implementation strategies for the translation of other AI-enabled algorithms.
Heart failure (HF) is a progressive chronic disease affecting approximately 6.2 million Americans. Identifying patients during the pre-symptomatic stages and implementing effective treatment strategies can reduce the risk of progression to clinically overt HF. Asymptomatic left ventricular systolic dysfunction (ALVSD), i.e., low ejection fraction (EF) without symptoms, affects 3% to 6% of the population and confers a 6.5-fold increased risk of symptomatic HF and 1.6-fold increased risk of all-cause mortality. Fortunately, there are guideline-recommended therapies (eg, angiotensin-converting enzyme inhibitors [ACEi] and β-blockers) that can effectively reduce the risk of HF and mortality.
However, identifying patients with ALVSD is difficult due to the lack of symptoms. Transthoracic echocardiography (TTE) to determine EF has been used as the standard method for diagnosing ALVSD. However, TTE is costly (~$200-$1000), time-consuming, and inconvenient for patients. An alternative strategy has been proposed to screen first with an inexpensive test and target individuals with a positive screening result for further diagnostic testing with TTE. Screening based on brain natriuretic peptide (BNP), N-terminal proatrial natriuretic peptide (NT-proBNP), and clinician-identified electrocardiogram (ECG) abnormalities have been proposed, but their performance was all insufficient to justify widespread screening.
A deep learning algorithm was developed to predict low EF based on ECG using 441,959 patients from a large health system. ECG is a noninvasive, painless, and nearly universally available test with quick results and low costs ($10-$50). It is ubiquitously performed in large populations for a variety of screening, diagnostic, and monitoring purposes, rendering this new ECG-based artificial intelligence (AI) algorithm a widely available and potentially cost-effective screening tool for ALVSD. In a prospective validation, the model demonstrated a c statistic of 0.92, a sensitivity of 82.5%, a specificity of 86.8%, and an accuracy of 86.5%. The performance was also similar across age, sex, and race subgroups.
A screening report was designed using a user-centered approach via an iterative process during which a series of prototypes were developed and tested. The AI algorithm was embedded in the electronic health records (EHR) to automatically generate a screening report every time an ECG is ordered. The report was included in a linked “data-in-brief” publication.
The objective of this study is to evaluate whether the new ECG AI-guided screening tool improves the diagnosis of LV systolic dysfunction in routine clinical practice. Secondary objectives include assessing the implementation strategies, the management of patients with newly diagnosed LV dysfunction, and the cost-effectiveness of using ECG to screen for low EF.
Methods
Study design
The EAGLE is a cluster randomized controlled trial (RCT) that will be conducted in 48 Mayo Clinic’s primary care practices in Rochester, rural Minnesota, and Wisconsin, including over 500 clinicians from both the academic medical center and the community practices. The primary care setting was selected because it is the site of care for most asymptomatic patients, where patients undergo ECGs for a wide number of indications, and where the need for timely diagnosis and early management of HF with reduced EF (HFrEF) is strongest.
Study participants will be randomized at the care team level, with each care team serving as a cluster. This is because primary care at Mayo Clinic is delivered on the care-team level, with physicians, advanced practice providers (APPs; nurse practitioners and physician assistants), nurses, and other health care professionals organized into discrete functional units. Patients are paneled on a clinician level, but to maximize patient access and continuity of care, most care is delivered at the care team level.
Study population
The study participants will be primary care clinicians (physicians, nurse practitioners, and physician assistants) who are part of a team that cares for adult patients (≥18 years) and have the ability to order ECG and TTE. We excluded pediatric care teams, resident care teams, acute and urgent care teams, and nursing home care teams.
There will be no contact with patients and patient data will be collected from the EHR, therefore, the informed consent will be obtained from clinicians, not patients. A patient’s data will be included in the analysis if the patient is ≥18 years old and receives an ECG during the trial period. Patients’ data will be excluded if they have known reduced EF, i.e., an EF ≤50%.
Randomization
Care teams will be randomized to intervention or usual care, stratified by region (Rochester, South East Minnesota [SEMN], South West Minnesota [SWME], North West Wisconsin [NWWI], and South West Wisconsin [SWWI]), and patient volume (i.e., the number of potential trial-eligible patients within the first 6 months of 2019). We will send clinicians in the eligible care teams a recruitment email. The email will explain that participation is voluntary and choosing to participate or not will not affect any aspect of their employment or work performance evaluation. We will send additional two follow-up email reminders to clinicians who do not respond, after which the study personnel will contact them via phone/pager or in person if necessary. If members of a care team decline to participate, the care team will still be eligible for randomization if others on the same team consent to participate. However, those clinicians who decline to participate will have not access to the report nor be contacted by the investigative team.
Intervention
During the trial period, clinicians in the intervention group will have access to a screening report in the EHR system. If a positive screening result occurs, clinicians will receive an email alert suggesting a TTE should be considered. The email alert will also contain a link where clinicians can document a reason for choosing not to proceed with TTE if that is their decision. A sample email alert was included in a linked “data-in-brief” publication. Follow-up reminders will be sent if no TTE is ordered and no reason is documented. A monthly report will be generated at the care team level to track the proportion of patients with a positive screening result, the proportion of patients receiving a TTE, and the proportion of patients receiving guideline-recommended therapies. The report will be sent to study participants at each care team randomized to the intervention. Clinicians in the usual care group will not receive any contact from the investigators.
Baseline measurements
At a provider level, the information will be collected using a survey administered at the time of enrollment, which will include demographic information, specialty, percent time in direct patient care, and comfort level with managing patients with LV dysfunction. We will also collect information on how many TTEs they ordered on patients with ECGs during the 6 months prior to the start of the trial as a measure of baseline practice pattern.
Patient-level baseline data will be collected from EHR ( Table I ). All data will be ascertained at the time of their index date, defined as the date when the screening result is available for a newly ordered ECG which will happen at the same time as the screening result becomes available. The time period prior to the index date (including the index date) is defined as the baseline period.
Patient Characteristics |
1. Socio-demographic |
Age |
Sex |
Race |
Ethnicity |
Rural/urban status |
Marital Status |
Insurance |
Income |
Education |
Limited English Proficiency |
2. Medical History |
Hypertension |
Diabetes |
BMI |
MI |
PAD |
Other ischemic heart disease |
Ischemic stroke or TIA |
Atrial fibrillation |
Other arrhythmias |
Valvular heart diseases |
Presence of ICD |
Chronic kidney disease |
Cancer |
Smoking |
Alcohol use |
Previous echo |
HbA1c |
NT-proBNP |
3. Medication Use |
ACEi |
ARB |
Beta Blocker |
Other hypertension medication |
Antidiabetic medications |
Statins |
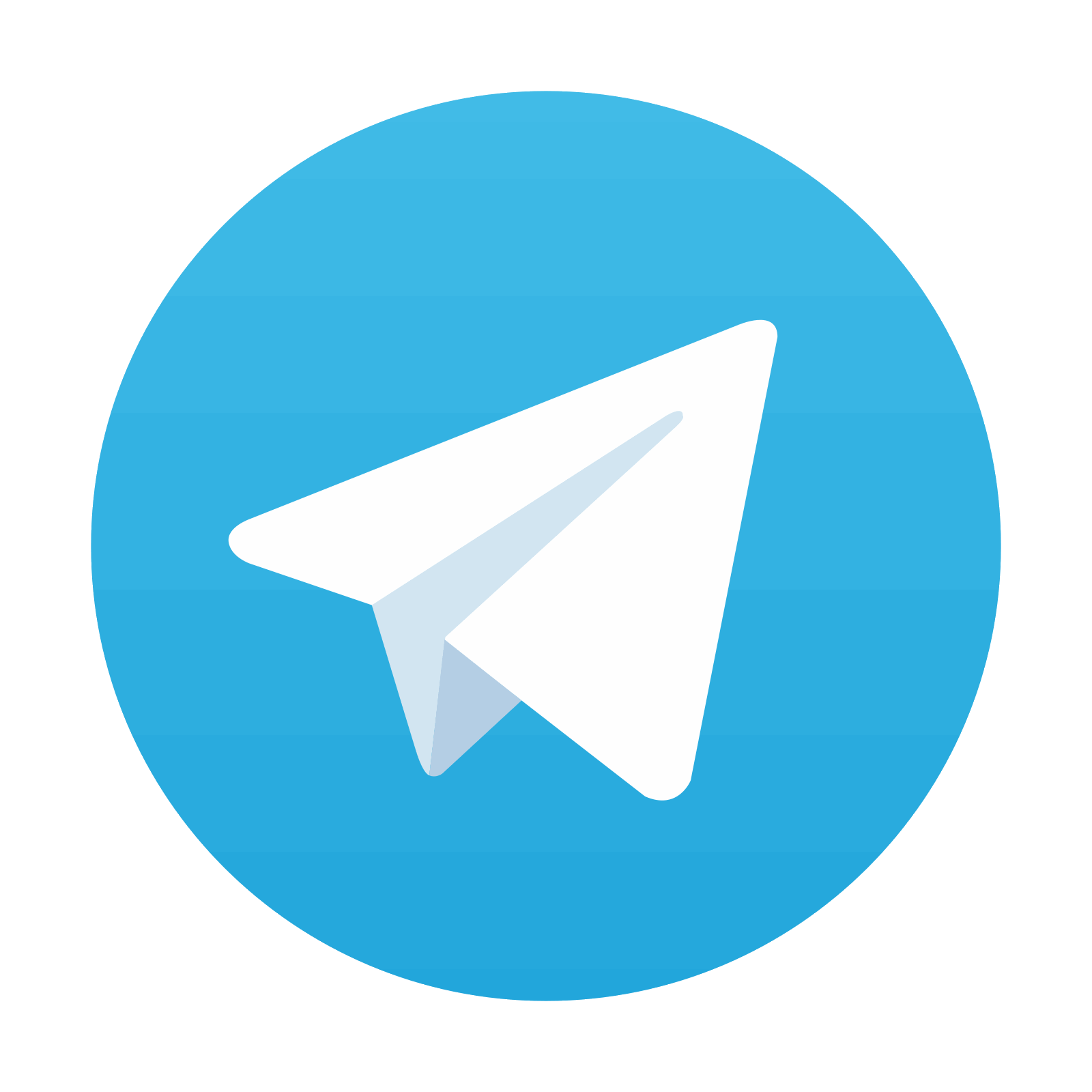
Stay updated, free articles. Join our Telegram channel

Full access? Get Clinical Tree
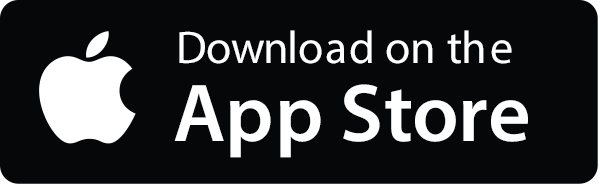

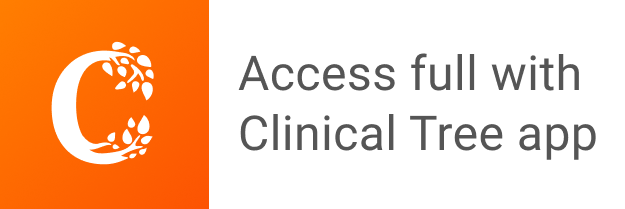