Number of patients with disease
Number of patients without disease
Total
Test positive
a (10)
b (1000)
a + b (1010)
Test negative
c (0)
d (998,990)
c + d (998,990)
Total
a + c (10)
b + d (999,990)
a + b + c + d (1,000,000)
Two-by-two tables (Table 8.1) focus on the sensitivity and specificity of the test. The test sensitivity [a/(a + c)] is the probability of a positive test given a disease. The test specificity [d/(b + d)] is the probability of a negative test in the absence of a disease. However, the question of interest is not the test sensitivity or specificity, but rather the positive predictive value of a test [a/(a + b)], which is the probability of disease given a positive test result. This probability depends on the prevalence of the disease in the population [(a + c)/(a + b + c + d)]. The main difficulty some students had in understanding the two-by-two table presentation of Bayes’ rule was the need to translate sensitivity and specificity data, which are commonly presented as proportions, into absolute numbers of patients with and without the disease under consideration. Therefore, I have not found such tables useful in explaining Bayes’ rule to students.
Natural frequencies have been claimed to be the most effective format for teaching test properties because it deals with numbers of patients rather than with proportions [9]. According to this format, in a hypothetical population of (a + b + c + d), there are (a + c) persons with the disease of interest and (b + d) without the disease. Of the (a + c) persons with disease, (a) will test positive and (c) will be false negatives. Of the (b + d) persons without disease (d) will test negative, while (b) will be false positives. The probability of disease given a positive test result is [(a/a + b)].
Likelihoods or odds are a third way to present Bayes’ rule, and this presentation seems to be the best approximation of diagnostic reasoning because it offers a single-step calculation of the post-test probability of disease. “Odds” are the ratio of the probability (p) of an event occurring over the probability of the event not occurring

The term pretest odds refers to the estimated likelihood of the disease before the application of the test. For example, in the case of the hypothetical disease presented earlier, the pretest odds of the disease are 1:100,000. The term post-test odds refers to the likelihood of the disease after the application of the test. Post-test odds are calculated by using the equation,

where the LR after a positive test result (LR positive) equals [sensitivity/(1−specificity)], and the LR after a negative test finding (LR negative) equals [(1 − sensitivity)/specificity]. For example, in the case of the hypothetical disease presented earlier, the pretest odds of the disease, 1:100,000, are extremely low. Therefore, even a superb test of 100 % sensitivity and 99.9 % specificity would increase the post-test odds of the disease to [1:100,000]*[1000:1] = 1:100 only. The test is not helpful in making a definitive diagnosis. However, if the hypothetical disease is a serious treatable condition, then the test may identify people who should be further examined.
Assessment of the Pretest Probability of a Disease
The application of Bayes’ rule requires an assessment of the pretest probability of the disease under consideration. In the example presented in the previous section, the pretest probability of the disease was its prevalence in the population. However, in clinical settings, doctors attend to specific patients. In such cases, the pretest probability of disease is subject to a doctor’s estimate.
The point of departure for the doctor’s estimate of the pretest probability of disease is its prevalence in the population. This probability is then readjusted using relevant data such as the setting of the encounter, the patient’s age and gender, and the presence of risk indicators for disease , of symptoms , and of the findings. For example, the LR positive of ischemic heart disease (gold standard: coronary angiography) is 5.8 for typical angina, 1.2 for atypical angina, and 0.1 for non-anginal chest pain; 3.8 for prior myocardial infarction; and 2.3 for diabetes [10].
Doctors vary in their subjective assessments of the pretest probability of disease [11], and appear to need guidance in probability estimation [12]. To provide such guidance and thereby reduce the variability of doctor assessments of disease probability, an effort has been made to develop “clinical prediction rules” that combine findings derived from the patient’s history, physical examination, and other easily available tests to provide an assessment of the probability of disease and the need for further testing.
Clinical Prediction Rules
The objective of clinical prediction rules is to help physicians identify patients who require either further diagnostic testing or treatment. These rules are derived from systematic clinical observations. Some clinical prediction rules assign relative weights to specific clinical data found to be associated with specific outcomes. Other prediction rules consist of algorithms or more complex mathematical models [13]. However, the derivation of a prediction rule is just the first step [14]. Similar to any diagnostic test, prediction rules require prospective validation that the predictions are indeed consistent with observed outcomes; that they are applicable to patients in specific clinical settings; and that their use improves a doctor’s decisions [15]. Similar to other diagnostic tests , the value of prediction rules is affected by differences in disease prevalence in different settings, and the failure to recognize and adjust for these differences may either thwart decision-making or lead to the premature dismissal of valid rules [16].
The number of published studies on prediction rules suggests that their popularity has been increasing since the 1990s [14]. For example, clinical prediction rules have provided three-level scores that distinguished between patients with a low, intermediate, and high probability of pulmonary emboli [17] and bacteremia [18]. Other clinical prediction rules have attempted to estimate the probability of streptococcal tonsillitis in adults with a sore throat. Its prevalence at a primary-care hospital clinic in New York was 24 %; it increased to 83 % if associated with all of the following: tonsillar exudates, cervical lymphadenitis, exposure to streptococcal contact, fever above 36.1 °C, and no history of cough [19]. Therefore, although a 2012 systematic review of the literature concluded that symptoms and signs, either individually or combined into prediction rules, cannot be used to definitively diagnose or rule out streptococcal pharyngitis [20], they do have a value in assessing its probability.
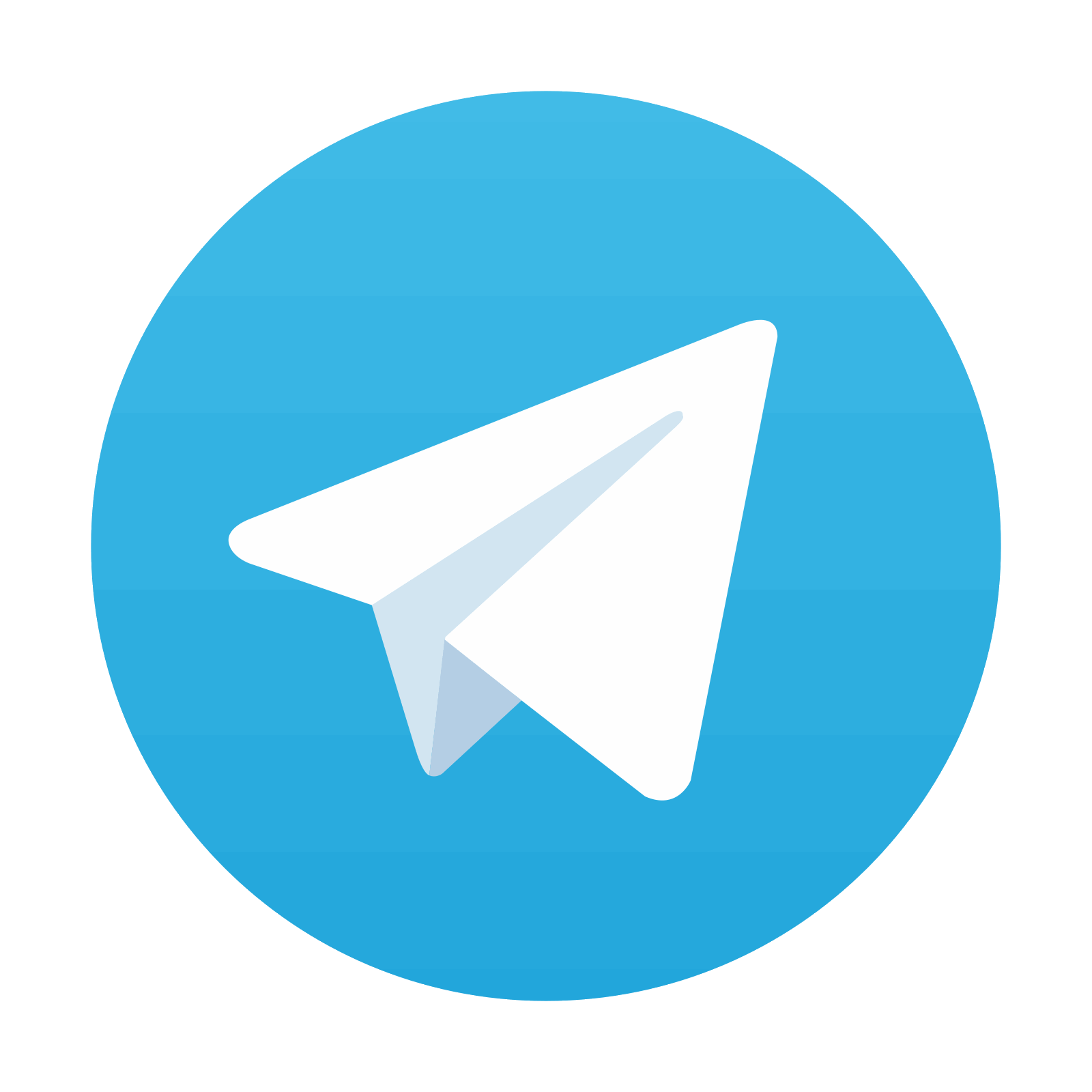
Stay updated, free articles. Join our Telegram channel

Full access? Get Clinical Tree
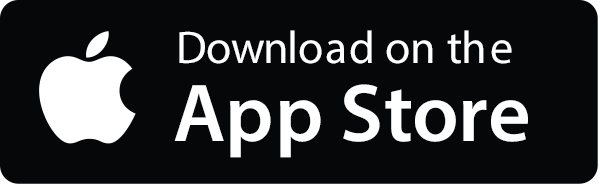
