In 2006, there were >1 million hospital admissions for heart failure (HF), and the estimated cost to the United States in 2009 was >$37.2 billion. Better models to target aggressive therapy to patients at the highest risk for readmission are clearly needed. We studied 3,413 consecutive admissions for HF based on discharge diagnosis codes from October 2007 to August 2011 from a single academic center. We randomly generated derivation and validation sets in a 3:1 ratio. We used generalized estimating equations to develop our models, accounting for repeated hospitalizations and the Hosmer-Lemeshow test to examine model calibration. The 30-day readmission rate was 24.2% in the derivation set. Of 25 candidate variables, the best fitting model included creatinine, troponin, hematocrit, and hyponatremia at discharge; race; zip code of residence; discharge hour; and number of hospitalizations in the previous year. Insignificant variables included intravenous diuretic use on day of discharge, discharge service, diabetes, atrial fibrillation, age, and gender. The risk of 30-day readmission increased with increasing decile of predicted risk in both the validation and derivation cohorts. The area under the receiver operating characteristic curve for the model was 0.69 in the derivation set and 0.66 in the validation set. In conclusion, we derived and validated a simple model relating discharge-specific characteristics at risk of 30-day readmission. Application of this approach may facilitate targeted intervention to reduce the burden of rehospitalization in patients with HF, but our results suggest that the best readmission models may require incorporation of both clinical and local system factors for optimal prediction.
Highlights
- •
We examined a real-world cohort of patients discharged with heart failure.
- •
From this data, we derive and validate a heart failure readmission model.
- •
Our model discriminates other widely used models with fewer data points.
- •
Local factors, including zip code, remain important in patients’ readmission risk assessments.
Using a large single-center registry of patients with heart failure (HF), with an index admission from 2007 to 2011, we sought to derive and validate a prediction model for readmission that included sociodemographic, admission, and discharge clinical characteristics.
Methods
All patients discharged with a primary discharge diagnosis for HF based on International Classification of Diseases (ICD)-9 codes (ICD-9 402.01, 402.11, 402.91, 404.01, 404.03, 404.11, 404.91, 404.93, 428.xx) at Beth Israel Deaconess Medical Center in Boston, Massachusetts, are followed in a prospective registry. Data elements taken from the electronic medical record and billing records include demographic, clinical, and dispositional variables. The primary outcome for our analyses was 30-day readmission. Our study population consisted of 3,413 index admissions for HF from October 1, 2007, to August 30, 2011. The data have been used to guide internal quality initiatives. The Institutional Review Board at Beth Israel Deaconess approved the study.
Demographic variables included age, gender, education level (eighth grade or less, some high school, high school graduate or equivalent, some college, college graduate, postgraduate studies, or unknown), race (white, black, Hispanic, Asian, or other), language (English or other), zip code (as a proxy for distance to the hospital), do-not-resuscitate status, and payer group (Medicare, Medicaid, and free care and other private insurances). Dispositional variables included season, day of the week (Monday to Thursday, Friday, or weekend day) and hour of discharge (11 a.m. to 7 p.m. or other), number of discharge medications, and discharge service (internal medicine, surgery, or cardiology). Clinical variables included discharge creatinine, blood urea nitrogen, hematocrit, glucose, and n-terminal pro-brain natriuretic peptide (BNP); change in blood urea nitrogen, creatinine, and BNP from admission to discharge; left ventricular ejection fraction; intravenous diuretic administration within 24 hours of discharge; presence of diabetes or atrial fibrillation (based on discharge diagnosis codes); and discharge medications including HF guideline-based therapies (angiotensin-converting enzyme inhibitor, angiotensin receptor blockers, β blocker, aldosterone antagonist, warfarin, and digoxin). Variables with a skewed distribution (number of discharges, number of medications, and length of stay) were analyzed using a logarithmic scale or winsorized at the ninety-fifth percentile (i.e., higher values set to the ninety-fifth percentile) to reduce the influence of the tails of the distribution.
We randomly assigned hospitalizations in a 3:1 ratio to derivation and validation subsets using computer-generated random numbers. We first determined the best fitting form for the variables. In variables with p <0.10, we used backward selection using cutoff of p <0.05 for inclusion. We included an interaction term for gender and marriage based on previous data suggesting that single men may have disproportionately worse outcomes than single women. After combining the best fitting categorical and continuous variables, backward selection was used to identify significant predictors at a threshold p <0.05. Generalized estimating equations (a semiparametric technique to account for possible unknown correlation between outcomes), with an exchangeable covariance structure, were used to account for clustering of patients with multiple admissions.
To assess model accuracy, we then tested it in our validation cohort. We tested model discrimination with the C-statistic, using methods derived for clustered data. We examined model calibration using Hosmer-Lemeshow tests (i.e., observed risk vs predicted risk among deciles of predicted risk); in Hosmer-Lemeshow tests, significant findings represent poor calibration of observed and predicted risks. We also tested the widely available Centers for Medicare and Medicaid Services model in our validation set to compare its discrimination with our model.
Because zip code reflects local factors such as, distance to the hospital or socioeconomic status, which might limit the generalizability of a model, we performed a secondary analysis that did not include zip code. For each zip code, we also evaluated the distance of its centroid to the medical center and its median income based on census data.
Results
Baseline clinical and demographic variables were similar in the derivation and validation sets ( Table 1 ), and the risk prediction model is presented in Table 2 . As in other models, lower sodium and hematocrit levels were associated with greater readmission risks. Creatinine was associated with risk in a log-linear fashion, consistent with its association with estimated glomerular filtration rate. Variables that were not significant in the derivation set included intravenous diuretic use on the day of discharge, discharge service, diabetes, atrial fibrillation, age, and gender.
Characteristic | Derivation Cohort (n = 2566) | Validation Cohort (n = 847) |
---|---|---|
30-Day readmission | 620 (24%) | 227 (27%) |
Age (years) | 74 (14) | 74.6 (14) |
Men | 1311 (51%) | 453 (54%) |
White | 1855 (72%) | 608 (72%) |
Black | 464 (18%) | 164 (19%) |
Asian | 49 (2%) | 18 (2%) |
Hispanic | 118 (5%) | 30 (4%) |
Other race | 77 (3%) | 26 (3%) |
English-speaking | 2168 (85%) | 716 (85%) |
Highest level of education | ||
Less than high school | 336 (13%) | 114 (14%) |
Some college or college graduate | 952 (37%) | 307 (36%) |
Insurance | ||
Medicare | 1696 (66%) | 571 (67%) |
Other insurance | 870 (34%) | 276 (33%) |
Discharge features | ||
Day of discharge | ||
Weekday | 837 (63%) | 533 (63%) |
Friday | 441 (17%) | 148 (18%) |
Weekend | 518 (20%) | 166 (20%) |
Discharge hour between 11A–7P | 2415 (94%) | 802 (95%) |
Number of discharges (past 6 months) | 2 (1, 3) | 2 (1, 3) |
Discharged from cardiology | 1314 (51%) | 437 (52%) |
Length of stay (days) | 3 (2, 6) | 3 (1, 6) |
Number of discharge medications | 13 (10, 17) | 13 (10, 17) |
Case mix index | 1.6 (1.4) | 1.5 (1.3) |
Baseline ejection fraction (%) | 50 (30, 60) | 50 (30, 60) |
Sodium (mEq/L) | 139 (137, 142) | 139 (137, 142) |
Blood urea nitrogen (mg/dL) | 30 (21, 47) | 31 (21, 46) |
Hematocrit (%) | 33.0 (29.5, 37.0) | 33.4 (29.9, 37.2) |
Creatinine (mg/dL) | 1.3 (1, 2) | 1.3 (1.0, 2.0) |
NT-proBNP (pg.mL) | 4722 (2030, 11009) | 4978 (2017, 11445) |
Glucose (mg/dL) | 105 (91, 134) | 107 (93, 131) |
Variable | Odds Ratios | 95% Confidence Limits | p Value |
---|---|---|---|
Race | 0.06 | ||
White | Reference | ||
Black | 1.10 | 0.82, 1.47 | 0.55 |
Asian | 0.42 | 0.14, 1.25 | 0.13 |
Hispanic | 1.36 | 0.90, 2.10 | 0.20 |
Other race | 0.63 | 0.33, 1.19 | 0.16 |
Discharge between 11 AM and 7 PM | 1.89 | 1.14, 3.13 | 0.01 |
Number of discharges | 1.24 | 1.17, 1.30 | <0.001 |
Zip code | 0.01 | ||
Other | Reference | ||
Zip 02119 | 1.72 | 1.04, 2.82 | 0.02 |
Zip 02446 | 0.61 | 0.32, 1.15 | 0.11 |
Zip 02130 | 1.64 | 1.08, 2.49 | 0.02 |
Zip 02125 | 1.68 | 1.06, 2.66 | 0.03 |
Zip 02131 | 1.19 | 0.63, 2.25 | 0.53 |
Log creatinine (per 1 mg/dL) | 1.42 | 1.18, 1.71 | <0.001 |
Hematocrit (per 1%) | 0.98 | 0.96, 0.99 | 0.01 |
Sodium (per 1mEq/L) | |||
Normal | Reference | ||
Low (<130) | 1.45 | 1.12, 1.86 | 0.01 |
Troponin T = 0 (ng/mL) | Reference | 0.02 | |
Troponin T <0.1 (ng/mL) | 1.31 | 1.02, 1.69 | 0.03 |
Troponin >0.1 (ng/mL) | 1.21 | 0.86, 1.68 | 0.27 |
No troponin ordered | 0.83 | 0.59, 1.17 | 0.29 |
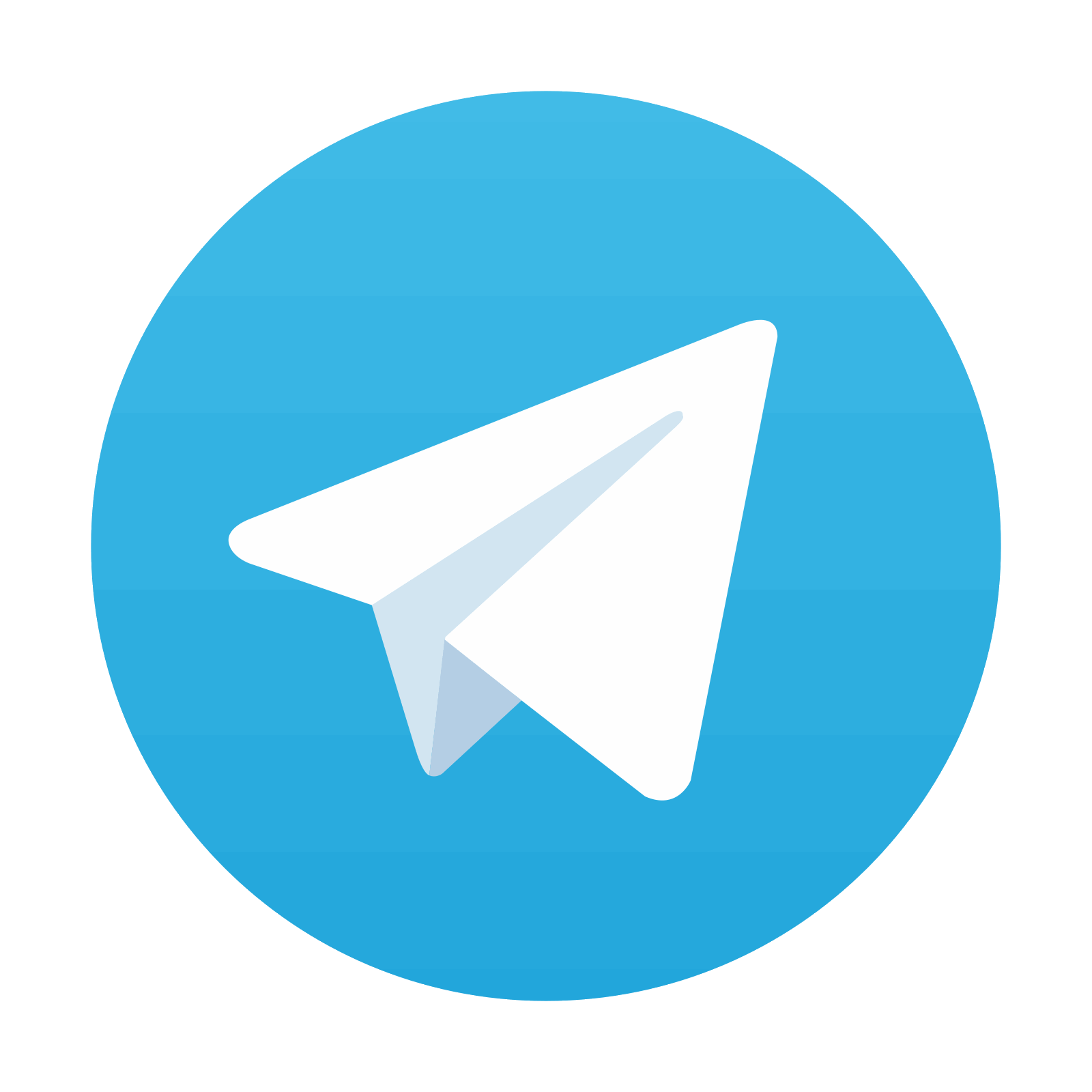
Stay updated, free articles. Join our Telegram channel

Full access? Get Clinical Tree
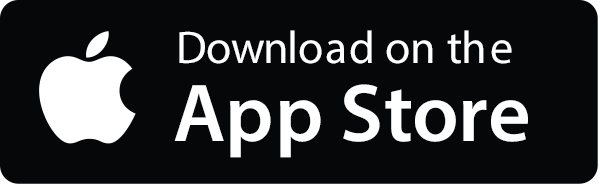

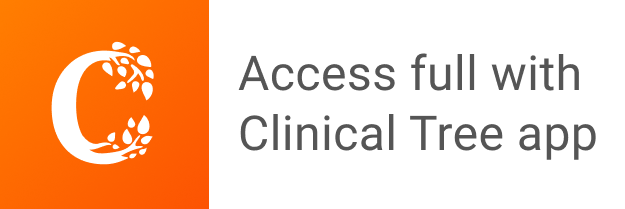