There is conflicting evidence regarding the impact of improving quality-of-care measures on patient outcomes. From July 2002 through June 2008, compliance with 3 in-hospital acute myocardial infarction quality-of-care measures (administration of aspirin and β blockers on arrival, timely reperfusion) and mortality were assessed in consecutive patients eligible for ≥1 of the measures at 8 hospitals (n = 6,826). Adjusted odds ratios for in-hospital and 30-day postadmission mortality and rate ratios for compliance with the 3 quality-of-care measures were calculated using marginal structural models to assess differences over time. Compliance with the 3 in-hospital quality-of-care measures improved significantly over the 6-year period. Adjusted odds ratios (95% confidence intervals) revealed significant decreases in in-hospital mortality in cohorts eligible for aspirin at arrival (year 6 vs baseline 0.37, 0.22 to 0.65), β blockers at arrival (year 6 vs baseline 0.24, 0.11 to 0.52), and an “all-eligible” measure comprising aspirin at arrival, β blockers at arrival, and timely reperfusion (year 6 vs baseline 0.41, 0.24 to 0.69). Significant decreases in 30-day postadmission mortality followed the same pattern (aspirin at arrival 0.53, 0.35 to 0.80; β blockers at arrival 0.43, 0.26 to 0.73; all-eligible measure 0.54, 0.36 to 0.81). In conclusion, over the 6-year study period, the health care system’s compliance with the 3 in-hospital quality-of-care measures and 30-day mortality improved significantly.
There is conflicting evidence regarding the impact of improving quality-of-care measures on patient outcomes. We sought to determine changes in compliance with 3 in-hospital quality-of-care measures (administration of aspirin and β blockers on arrival, timely reperfusion) and short-term mortality for acute myocardial infarction (AMI) over a 6-year period in a large integrated health care system that invested substantial time and resources in measuring, reporting and improving compliance with quality-of-care indicators and mortality.
Methods
Baylor Health Care System (BHCS) is an integrated health care delivery system in north Texas comprising 14 owned, leased, and affiliated hospitals and >100 ambulatory primary care, speciality care, and senior health care centers. Centers for Medicare and Medicaid Services core-measures data for all patients with AMI is required to be entered into MIDAS (Tucson, Arizona), the health care system’s core-measures vendor. In recent years, BHCS has engaged in several quality-improvement initiatives targeting compliance with these measures and/or mortality including exposure of these measures to the administrator pay-for-performance program, implementation of a clinical champion model, and introduction of regular audit and feedback on measures performance to leaders and frontline clinical staff.
For this study, we included all patients discharged from July 1, 2002 through June 30, 2008 from 1 of the 8 hospitals that were part of BHCS in July 2002 (ranging in size and location from an urban 1,002-bed tertiary care academic hospital with >40,000 admissions per year to a 69-bed community hospital with approximately 4,000 admissions per year outside the urban metropolis) and eligible for ≥1 in-hospital AMI quality-of-care measure—timely reperfusion (percutaneous coronary intervention within 90 minutes or thrombolytic therapy within 30 minutes of arrival), aspirin at arrival, and β blockers at arrival—as defined in the Specifications Manual for National Hospital Quality Measures . This “eligibility” requires an International Classification of Diseases, Ninth Revision, Clinical Modification principal diagnosis code of 410.00 to 410.91 and patient age ≥18 years. Patients admitted through the emergency department were included, but patients transferred from other facilities were not.
The study obtained institutional review board approval and waiver of informed consent from Baylor Research Institute in May 2010.
Primary measurements of interest were performance on the 3 AMI measures, i.e., in-hospital mortality, defined as an encounter with a discharge disposition equal to expired, and 30-day postadmission mortality, defined as an encounter with in-hospital death and length of stay ≤30 days or postdischarge death occurring within 30 days of admission. A composite quality-of-care measure was also examined: the “all-eligible” measure that for each patient comprised all 3 AMI measures considered (timely reperfusion, aspirin at arrival, and β blockers at arrival) for which the patient was eligible. Only AMI quality-of-care measures with potential to impact in-hospital mortality were considered.
Patient demographic and quality-of-care measure data were obtained using MIDAS, the core-measures vendor used in all health care system hospitals, and vital status was determined from the Social Security Administration Death Master File.
An analytic data set with AMI discharges from July 1, 2002 through June 30, 2008 was created from these sources. MIDAS data were used to create variables related to patient demographics, patient medical history, cardiac risk factors, and cardiac severity factors ( Table 1 ). All variables related to a co-morbid condition were derived using the method of Elixhauser et al.
Year | ||||||
---|---|---|---|---|---|---|
1 (July 2002–June 2003) (n = 1,196) | 2 (July 2003–June 2004) (n = 1,139) | 3 (July 2004–June 2005) (n = 1,158) | 4 (July 2005–June 2006) (n = 1,140) | 5 (July 2006–June 2007) (n = 1,044) | 6 (July 2007–June 2008) (n = 1,149) | |
Patient characteristics | ||||||
Age (years), mean ± SD | 67.1 ± 14.6 | 65.2 ± 13.8 | 65.2 ± 14.2 | 64.9 ± 14.6 | 65.1 ± 14.2 | 65.3 ± 14.7 |
Men | 60.0% | 65.4% | 61.1% | 60.9% | 63.8% | 61.2% |
White | 76.2% | 73.8% | 73.4% | 72.0% | 73.8% | 68.5% |
Black | 12.0% | 12.8% | 13.2% | 14.3% | 12.5% | 14.5% |
Hispanic | 6.5% | 7.8% | 9.8% | 8.4% | 9.9% | 11.8% |
Other | 5.3% | 5.5% | 3.6% | 5.3% | 3.9% | 5.1% |
Payer type | ||||||
Medicare | 54.0% | 47.8% | 46.1% | 46.1% | 42.9% | 46.2% |
Medicaid | 2.2% | 3.2% | 2.7% | 1.9% | 1.3% | 2.5% |
Insured | 36.2% | 35.6% | 40.9% | 38.8% | 43.9% | 38.6% |
Uninsured | 7.6% | 13.4% | 10.3% | 13.2% | 11.9% | 12.7% |
Medical history ⁎ | ||||||
Anemia | 9.4% | 11.6% | 16.7% | 17.8% | 16.7% | 21.2% |
Arthritis | 1.7% | 0.9% | 1.5% | 1.8% | 2.1% | 1.5% |
Coagulopathy | 3.3% | 2.9% | 5.4% | 5.6% | 5.3% | 5.0% |
Depression | 3.2% | 3.2% | 6.4% | 6.4% | 6.6% | 8.1% |
Liver disease | 0.7% | 1.6% | 1.4% | 1.9% | 1.0% | 1.5% |
Fluid disorders | 17.8% | 20.7% | 21.6% | 19.4% | 22.0% | 25.0% |
Peripheral vascular disease | 8.2% | 9.9% | 12.3% | 14.2% | 14.1% | 12.4% |
Renal failure | 4.8% | 4.9% | 5.1% | 11.1% | 15.7% | 18.7% |
Diabetes | 23.9% | 28.0% | 26.9% | 29.2% | 28.5% | 31.9% |
Hypertension | 50.0% | 57.9% | 60.8% | 62.0% | 65.9% | 70.7% |
Obesity † | 7.0% | 9.5% | 11.2% | 9.4% | 9.5% | 13.1% |
Smoker | 30.0% | 37.0% | 36.4% | 33.2% | 35.8% | 32.6% |
Left ventricular systolic dysfunction ‡ | 26.7% | 24.7% | 24.0% | 24.9% | 25.0% | 22.5% |
Heart failure § | 6.8% | 2.5% | 0.9% | 0.9% | 0.8% | 1.1% |
Chronic pulmonary disease | 16.4% | 17.4% | 17.0% | 15.9% | 18.2% | 18.5% |
Other | ||||||
Admitted from emergency room | 79.2% | 78.6% | 84.8% | 83.5% | 85.2% | 89.4% |
⁎ All variables related to co-morbid conditions were derived using the method of Elixhauser et al as applied in the AHRQ co-morbidity software. The method of Elixhauser et al identifies co-morbidities based on the combination of the presence of certain International Classification of Diseases, Ninth Revision, Clinical Modification codes and absence of certain diagnosis-related group codes. Other variables considered in the statistical model but not presented because of very low prevalence (<1%) and little change over the 6 years were acquired immunodeficiency syndrome, drug abuse, alcohol abuse, peptic ulcer disease, valvular disease, lymphoma, metastatic cancer, neoplasm without metastasis, other neurologic disorders, and pulmonary circulation disorders.
† Chart documentation of overweight or obesity ( International Classification of Diseases, Ninth Revision, Clinical Modification code 278.0) in the absence of diagnosis-related group codes indicating an obesity procedure (code 288) or nutrition/metabolic diagnosis (codes 296 to 298).
‡ Chart documentation of left ventricular ejection fraction <40% or narrative description of left ventricular systolic function consistent with moderate or severe systolic dysfunction.
§ Chart documentation of rheumatic heart failure ( International Classification of Diseases, Ninth Revision, Clinical Modification code 398.91); benign hypertensive heart disease with heart failure (code 402.11); unspecified hypertensive heart disease with heart failure (code 402.91); hypertensive heart and chronic kidney disease, benign, with heart failure and with chronic kidney disease stages I through IV, or unspecified (code 404.11); benign hypertensive heart and renal disease with heart failure and renal failure (code 404.13); unspecified hypertensive heart and renal disease with heart failure (code 404.91); unspecified hypertensive heart and renal disease with heart failure and renal failure (code 404.93); heart failure (codes 428.0 to 428.9); or in the absence of diagnosis-related group codes indicating cardiac disease (103 to 108, 110 to 112, 115 to 118, 120 to 127, 129, 132 to 133, 135 to 143).
AMI quality-of-care measures data were used to determine treatments received and to further subset the data into analytic cohorts of interest. To rigorously account for the possible confounding effect of time at admission, time was scaled in a continuous fashion over the span of 1 week with 0 hour referring to Monday 12.00 a.m. and 167.98 hours to Sunday 11.59 p.m. We linked patient data to the Social Security Administration Death Master File to identify postdischarge deaths using a 2-stage process: (1) using Social Security Number and date of birth and (2) for records that did not match in this first step, using patient’s first name, last name, and date of birth. All patients with a second-stage match were manually reviewed for potential false-positive findings.
Marginal structural models were used to calculate adjusted odds ratios for in-hospital and 30-day postadmission mortality and rate ratios for compliance to the 3 quality-of-care measures to assess differences over time. Marginal structural models were fitted as follows. Propensity scores were estimated from a multinomial logistic regression model with fiscal year as the outcome and possible confounding factors (including those listed in Table 1 , admitting hospital and time at admission) as covariates. To ensure rigorous adjustment for confounding, covariates in the analytic dataset included all elements considered in validated models and several additional clinical elements. Continuous covariates were not forced into categories; smoothing techniques (restricted cubic splines ) were used instead. Weights were then calculated as the inverse of the propensity score for the actual fiscal year of the encounter and applied to the final outcome models, which contained only fiscal year as a covariate. For in-hospital and 30-day postadmission mortality, logistic regression models were fitted to the weighted data. Odds ratios derived from these propensity-weighted models represent the relative change in odds of the outcome between the reference fiscal year and each subsequent fiscal year after adjustment. Because quality-of-care measure compliance outcome is more prevalent, rate ratios, derived from a log-binomial model, were used to assess the effect of time. Effect measurements (odds and rate ratios) reported in this study are commonly known as inverse probability-of-treatment weighted estimators. The 95% confidence intervals reported for the odds/rate ratios are based on robust (“sandwich”) variance estimates.
All analyses were executed using the Genmod procedure in SAS 9.1.3 (SAS Institute, Cary, North Carolina).
Results
In total 6,826 patients met the eligibility criteria and were considered for the present investigation. Patient characteristics including demographic and clinical details are presented in Table 1 . During the 6 years considered, >1,000 patients a year were admitted with AMI and were eligible for ≥1 of the AMI quality-of-care measures of interest. Some substantial changes in patient characteristics were observed: the proportion of patients who were white decreased by 8 percentage points, as did the proportion insured through Medicare, whereas the uninsured proportion increased by 5 percentage points; the proportion of patients admitted from the emergency room increased by 10 percentage points; and the prevalence of several co-morbidities (depression, fluid and electrolyte disorders, obesity, diabetes, and diabetes with long-term complications) increased by 5 to 10 percentage points, whereas deficiency anemias, renal failure, and hypertension increased by 10 to 20 percentage points. However, prevalence of congestive heart failure and of solid tumor without metastasis each decreased 5 percentage points ( Table 1 ).
Yearly unadjusted in-hospital mortality rates for the cohorts ranged from 1.7% to 7.5% and 30-day postadmission mortality rates from 3.5% to 9.9% ( Table 2 ). Table 2 also lists unadjusted compliance with the 3 AMI quality-of-care measures for each year, which ranged from 48% for timely reperfusion in the baseline year to 98.3% for β blockers at arrival in year 4.
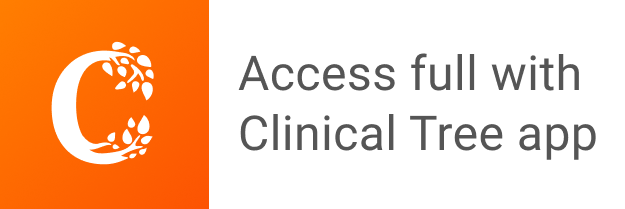