Biomarkers that focus on lung cancer risk assessment, detection, prognosis, diagnosis, and personalized treatment are in various stages of development. This article provides an overview of lung cancer biomarker development, focusing on clinical utility and highlighting 2 unmet clinical needs: selection of high-risk patients for lung cancer screening and differentiation of early lung cancer from benign pulmonary nodules. The authors highlight biomarkers under development and those lung cancer screening and nodule management biomarkers post-clinical validation. Finally, trends in lung cancer biomarker development that may improve accuracy and accelerate implementation in practice are discussed.
Key points
- •
Biomarkers can be used for risk assessment, detection, diagnosis, and prognosis and to personalize treatment in lung cancer.
- •
Clinically useful biomarkers for selection of high-risk patients for lung cancer screening and to differentiate early lung cancer from benign pulmonary nodules are needed.
- •
Biomarkers for nodule management and determination of high-risk groups for lung cancer screening are at all phases of development, from discovery to clinical utility studies.
- •
Current trends in lung cancer biomarker development include the integration of clinical and radiologic features with molecular biomarkers, the application of artificial intelligence to molecular and imaging biomarker development, the use highly sensitive technologies such as next-generation sequencing for molecular exploration, and a commitment to high-quality clinical validation and utility studies.
Introduction
Lung cancer is diagnosed in more than 1.8 million people yearly and remains the leading cause of cancer deaths in both developing and developed countries, with survival at 5 years a disappointing 19%. , This poor survival rate is attributable to many factors, including lung cancer diagnosis at a late stage, when cure is uncommon with currently available therapies. Biomarkers that accurately predict lung cancer risk may aid in targeting intensive preventative interventions to those at the highest risk of lung cancer development. Lung cancer screening (LCS) by low-dose computed tomography of the chest (LDCT) decreases mortality by diagnosing lung cancers at an early stage, when treatment is potentially curative. The population for whom LCS would be most beneficial is not definitively identified. A biomarker that better identifies patients likely to benefit from LCS, particularly healthy patients at high lung cancer risk not currently offered LCS, would be beneficial. In addition, LDCT identifies both cancerous and noncancerous lung nodules. A biomarker that distinguishes between benign and malignant nodules can help to avoid potentially dangerous procedures for those without cancer. , Particularly in non–small cell lung cancer (NSCLC), much progress has been made to classify lung cancers by molecular markers, some of which can be used to personalize therapy. Now many are actively developing and testing the utility of predictive, diagnostic, and prognostic lung cancer biomarkers ( Fig. 1 ). This article focuses on the development, current utilization, and future trends of lung cancer biomarkers and provides specific examples of how these can impact management.

Biomarker development
Biomarkers to predict, diagnose, and prognosticate lung cancer are being developed at a rapid rate, fueled by an increasing knowledge of normal and malignant genetic, epigenetic, and immunologic signatures and increasingly available samples, big data, and methods with which to analyze them. The Institute of Medicine has developed a framework by which -omics-based tests can be developed and evaluated for scientific rigor. The Centers for Disease Control and Prevention ACCE model of biomarker development includes 5 distinct phases: discovery, analytical validation, clinical validation, clinical utility, and associated implementation factors (including ethical, legal, and social implications, such as cost-effectiveness) ( Fig. 2 ). These concepts have been applied to the development, implementation, and evaluation of biomarkers for lung cancer diagnosis and screening.

Discovery
There is an accelerated pace to lung cancer biomarker discovery. In this phase, potential biomarkers are identified, confirmed, and prioritized for validation. The initial identification can use cell culture or human samples, available databases, or a combination of these resources. It is important at this very early stage of biomarker development to consider several factors. First, the biospecimen used for the assay must be easily accessible, simple to prepare and store, and available in sufficient amounts for biomarker measurement. Lung cancer biomarkers have been developed from blood components, sputum, exhaled breath, urine, and oronasal and bronchial epithelium to measure molecular targets, such as tumor and immune antigens, autoantibodies, messenger RNA (mRNA) and microRNA (miRNA), DNA methylation, circulating free tumor DNA, and circulating tumor cells, among others. The discovery phase has 3 components : Component 1: quality control determines the accuracy and reproducibility of the measurement, including optimizing operating procedures (collection, storage, methods) and meeting minimally acceptable variations in results; Component 2: a training set is used to develop the modeling and fitting of the biomarker for its intended use; Component 3: confirmation of the computational modeling with a separate testing set. Although more fastidiously required during the later stages of biomarker development, use of specimens from the intended use population is favored during the biomarker discovery phase, because the use of appropriate samples for test development and validation may prevent failure of test validation. For instance, a serum biomarker that uses a cancer antigen to differentiate between patients with stage IV NSCLC and those without cancer in a test cohort may not be able to detect earlier stage NSCLC. Similarly, a lung nodule biomarker able to detect different serum miRNA signatures in a training set containing specimens from patients with lung cancer who are smokers compared with a control population comprising mostly non-smokers may select for a biomarker able to differentiate between smokers and non-smokers, but may be unable to differentiate between cancerous and noncancerous nodules.
Analytical Validation
After a biomarker has completed the discovery phase, the test must go through analytical validation, which establishes acceptable performance characteristics of a biomarker in the intended use population. At this point, the established methods and modeling are applied to a new validation cohort, separate from that used in the discovery phase, containing samples representing the intended use population. The analytical validation evaluates the reproducibility of a biomarker (low interlaboratory variability, repeatability over time, and from sample to sample). Accuracy and precision of the assay, analytical sensitivity/specificity, linearity, limits of detection, cutoff values, and intraassay and interassay coefficients of variation are assessed in this phase. For these measurements, recommendations for minimally acceptable standards are available. Analytical validation requires collection of samples from patients for a specific purpose and requires institutional review board approval. Most biomarker developers will have consulted with the Food and Drug Administration (FDA) by this point. ,
Clinical Validation
The clinical validation phase, sometimes referred to as the biological validation phase, is used to determine the diagnostic accuracy of a biomarker in the intended use population. Accuracy is compared with a relevant reference standard and must improve upon currently available assessment tools, making evaluation in the appropriate population essential. For instance, for a lung nodule management biomarker, the clinical validation phase would use specimens from patients with lung nodules determined to be lung cancer as well as specimens from patients with benign lung nodules. Analyzing clinical validity requires a blinded, intended-use specimen set, unique to the samples used in the discovery and analytical validation training sets. These specimens are usually obtained from different institutions as well to eliminate geographic and associated population differences as a potential bias. Using locked-down biomarker thresholds developed in the discovery and analytical validation phases, clinical validation determines performance characteristics of a biomarker in this population, including sensitivity, specificity, positive predictive value, negative predictive value, likelihood and hazard ratios, and area under the curve or receiver operating characteristic curve analysis. Findings from a clinical validation study can be used to estimate the predicted clinical impact of using the test in the intended use population. For instance, the clinically validated accuracy of a biomarker used to stratify intermediate risk pulmonary nodules into a high- and low-risk category could be used to estimate the potential impact of the results if it were used to impact clinical decisions. However, clinical utility assessment is needed to confirm these estimates. Results from clinical validation studies have been used for FDA approval and coverage of a biomarker by public or private insurance sources.
Clinical Utility
Clinical utility is the ultimate determinant of a biomarker’s performance, because it reflects how the results affect clinical decision making and patient outcomes. For this reason, a highly reliable and accurate biomarker may not always be clinically useful. There are many possible reasons for this. For one, clinical utility takes into consideration both the benefits and the harms of using a biomarker, and use in the appropriate context is necessary for a favorable benefit-to-harm ratio. , In Fig. 3 , the authors provide an example of a highly accurate biomarker that is potentially harmful when applied to the wrong population. Clinical utility takes into consideration how biomarker results affect clinical decisions beyond estimates extrapolated from clinical validation studies. Misinterpretation of the results or mismanagement owing to inaccurate or incorrectly interpreted results can result in harm or increased costs to the patient. Finally, lung cancer biomarkers must improve upon currently available assessment tools to be clinically useful. There are several different ways to assess clinical utility. More detailed examples of lung cancer biomarker clinical utility, including trial design, are available.

Cost-Effectiveness, Implementation, and Policy Considerations
If a biomarker is determined to be clinically useful in a specific population for a defined purpose, the impact of widespread utilization is often evaluated before policy recommendations for implementation. One of the measurements to estimate societal impact is cost-effectiveness analysis (CEA). The measurement of biomarker cost-effectiveness is complex but important to determine the ultimate utility to society. Several groups have provided guidelines on the evaluation, reporting, and utilization of CEA. CEA is commonly measured as quality-adjusted life-years (QALY), which takes into account not only the lives saved by a certain intervention but also the quality of life during that time, or as an incremental cost-effectiveness ratio, which compares the cost-effectiveness of a biomarker to the existing standard of care. CEA compares the net cost of implementing a biomarker to a specific outcome, most commonly on a societal scale. The cost of a test should account for all net expenditures, including fixed costs (eg, equipment and materials needed to perform the test), direct costs (eg, physician and technician time to perform and interpret the test), and indirect costs (eg, cost of additional studies based on test results, potential complications of the testing).
There are several potential confounders to CEA, including cost variability, type of outcome measured, demographics, geography, and resource availability. It is important to use large, well-designed trials that include the appropriate intended use population for CEA. For instance, the cost-effectiveness of LCS by LDCT is estimated at $81,000/QALY gained, within the range typically considered cost-effective. However, the confidence interval ranges from $52,000 to $186,000/QALY gained, depending on the lung cancer risk of the population by variation of sex, age, and smoking status at the time of screening. These calculations highlight how defining a particular population may alter cost-effectiveness measures. Biomarkers may serve as adjunctive tools to further improve cost-effectiveness of LCS by LDCT by (1) defining a higher-risk population for screening or (2) improving the management of lung nodules, thereby limiting additional workup, including procedural, surgical, or radiologic follow-up. Examples of calculations to assess favorable CEA in lung cancer biomarkers have been previously outlined. ,
In addition, other less quantifiable factors should be considered in biomarker implementation, including resources needed to perform and interpret the analysis and the impact on the individual (eg, pain, distress, time, and loss or gain in productivity). On a larger scale, policy decisions may consider allocation of resources (eg, in the distribution of research funds for biomarker development) and implementation considerations, which are not directly addressed in most CEA, but are important when determining the impact of the biomarker in an intended use population.
Clinical utility evaluation
Lung Cancer Prevention
There have been extensive efforts to determine those at high risk for lung cancer development. Several risk calculators for lung cancer development are available that use clinical data. , All major lung cancer risk calculators include age and tobacco smoking history, and variably other risk factors, including asbestos exposure, family history, presence of chronic obstructive pulmonary disease, prior cancers, prior pneumonia, low level of education, and race/ethnicity, with some models including sex/gender. The impact of other exposures and diseases associated with increased lung cancer risk, including exposure to radon, polycyclic aromatic hydrocarbons, heavy metals, human immunodeficiency virus infection, and the presence of interstitial lung disease, are not routinely included in risk calculators. Although no single biomarker has been found that accurately predicts the risk of developing lung cancer, specific genetic polymorphisms, including those in the nicotinic acetylcholine receptor gene, are strongly associated with nicotine addiction and increased risk of lung cancer. Intervention on this finding is cessation of tobacco smoking. However, tobacco smoking is one of the 2 strongest risk factors for lung cancer development (age being the other). Because of this factor alone, in addition to the high morbidity associated with other tobacco-related diseases, aggressive tobacco cessation efforts should be offered to all smokers regardless of biomarker results, making this a poor target for development of a preventative biomarker development when used alone. However, a biomarker that could improve upon currently available clinical risk prediction tools may be beneficial in the selection of cohorts for chemoprevention studies or as a surrogate endpoint in prevention intervention studies.
Lung Cancer Screening
The National Lung Screening Trial (NLST) randomized more than 53,000 patients (30 pack-year current or former smokers who quit within the last 15 years, aged 55–74) to either LDCT or chest radiographs yearly for 3 years. Those who were randomized to LDCT had a 20% relative risk reduction of lung cancer death. This finding has led to the recommendation for LCS in high-risk smokers by several specialty- and disease-specific societies and led to the decision by the Centers for Medicare and Medicaid Services (CMS) to cover LCS services. Another large randomized controlled trial in Europe suggests an even greater survival benefit from LCS using LDCT extending beyond 3 years. However, LCS is associated with both direct and indirect risks, many of which are associated with a large number of benign nodules (false positives) identified by LDCT. Nodules were identified on 24% of LDCTs, most of which (96%) are ultimately discovered to be benign, but may lead to invasive surgical and nonsurgical diagnostic procedures, associated with morbidity and less likely death. , Additional risks and concerns raised have included patient quality of life, overdiagnosis, risks and costs of unnecessary procedures, the quality, compliance, and cost of widespread implementation, and secondary malignancies from radiation exposure from imaging. , Furthermore, there is also concern about patient selection for LCS: (1) that the NLST criteria may exclude populations at high risk for lung cancer development, which may benefit but are excluded from LCS; and (2) that certain patient populations included in NLST criteria may be less likely to benefit and more likely to have complications of LCS. ,
A biomarker may therefore benefit LCS in several ways. First, a biomarker of lung cancer risk may aid in discussions of individual risk-to-benefit ratio for LCS. In order to do this, the biomarker would need to be more accurate at identifying those at high or low risk of having/developing potentially curative lung cancer than currently available risk stratification criteria (eg, current LCS enrollment criteria or available clinical risk assessment tools), either alone or in combination with these tools. Compared with the standard of care, that biomarker must either (a) lead to fewer lung cancer deaths in a screened population without increasing harms or expense or (b) lead to a similar number of lung cancer deaths in a screened population but decrease harms or expense. For instance, a biomarker may be able to select for a population at high risk of lung cancer development not included in the currently screened population, who would benefit from LCS. Similarly, a biomarker that predicted those at highest risk for lung cancer could select a population within those currently offered LCS, which will most benefit while excluding, and thereby reducing potential risks, to those unlikely to benefit. Alternatively, a biomarker that would predict those at lowest risk of lung cancer may identify a patient, currently considered for LCS but with an unfavorable benefit-to-risk ratio, reducing potential harms and costs associated with LCS. Specific ways in which an LCS biomarker can be tested against a standard of care to determine clinical utility are available.
Nodule Management
The increased use of chest computed tomography (CT) scans, for both LCS and diagnostic indications, has led to an increase in the identification of pulmonary nodules. Although some of these nodules are immediately identified as high or low risk of lung cancer based on imaging characteristics, many are considered intermediate risk. Subsolid nodules have a higher risk of malignancy than solid nodules, but typically behave in a more indolent fashion. For this reason, they can be followed radiologically for growth or development of a solid component, at which time surgical resection is the preferred treatment. For solid, noncalcified nodules measuring 8 to 30 mm in diameter without benign characteristics, a biomarker that could differentiate benign from malignant would be beneficial. Several lung nodule risk calculators have been developed for risk assessment of these intermediate-risk nodules, with utility variable by cohort. , Malignancy risk, determined by nodule risk calculators or physician estimation, is then used to determine initial management strategies based on malignant risk stratification into very low (≤10%), intermediate (∼11%–64%), and high risk (>65%), with cutoffs variable depending on patient preference and comorbidities. , Very low-risk nodules are typically managed by close radiologic follow-up. High-risk nodules are managed by definitive treatment (surgical resection or stereotactic radiotherapy). Intermediate-risk nodules require further evaluation, which can include additional imaging (eg, PET) or biopsy (percutaneous or bronchoscopic). PET combined with CT, although highly sensitive (∼90%), has a lower specificity (61%–77%), which is even lower in areas with a high incidence of endemic mycoses. Percutaneous nodule biopsies have a high diagnostic yield, but are associated with a risk of complications, including pneumothorax, particularly in this patient population that may have tobacco-associated emphysematous changes. , Bronchoscopic biopsy, although having a lower risk of pneumothorax, is performed in selected individuals based on nodule characteristics and has variable diagnostic yields even when performed by highly experienced proceduralists. , It is these intermediate-risk nodules that would most benefit from further risk stratification by a pulmonary nodule biomarker.
A few considerations are important in a nodule management biomarker. In order to be clinically useful, a lung nodule management biomarker must be more accurate than existing pulmonary nodule risk assessment tools (nodule risk calculators, PET) or improve accuracy when combined with these risk assessment tools. Although theoretically a biomarker could identify a nodule as either high or low likelihood of malignancy, most current nodule management biomarkers are classified as either rule-in (high likelihood of malignancy) or rule-out (low likelihood of malignancy). As such, a potentially useful rule-in lung nodule biomarker will increase the probability of malignancy in an intermediate-risk pulmonary nodule above the threshold for recommending definitive therapy (surgery or stereotactic body radiation therapy [SBRT]). The benefit of this biomarker would be to avoid possible delay or complications associated with diagnostic procedures. A potentially useful rule-out lung nodule biomarker decreases the probability of a malignancy in an intermediate-risk pulmonary nodule low enough to warrant radiologic surveillance. The potential benefit of such a biomarker would be to avoid complications and costs of invasive diagnostic procedures in those with benign nodules. In both of these scenarios, it is important to stress that clinical validity does not equate to clinical utility. Clinical utility in a nodule management biomarker requires either (a) that lung cancers are diagnosed earlier without significantly increasing procedures performed on benign nodules or (b) that fewer procedures are performed on benign nodules without a clinically significant delay in the diagnosis of lung cancer in those with malignant pulmonary nodules. However, even if those criteria are fulfilled, a lung nodule biomarker may have limited clinical utility. For instance, a patient with a very early or slow-growing lung cancer, correctly identified as malignant by a lung nodule biomarker, may have a complication during a surgery or intervention for a cancer that would have remained indolent (ie, never cause the patient’s death). Alternatively, a false negative biomarker result on a very small, early lung cancer that is followed by close radiologic surveillance may result in a delay in diagnosis that is not associated with any increase in mortality. For this reason, it is important that these biomarkers are applied to the intended use population and trials to address clinical utility are designed with these specific outcomes in mind. Another publication gives detailed examples of such clinical utility trial designs.
Lung Cancer Prognosis and Prediction of Response to Therapy
In patients already diagnosed with lung cancer, there are several ways in which a biomarker may be of benefit. It can be used to predict tumor behavior, often measured by the surrogate of patient survival. The best prognostic marker in NSCLC is TMN stage, which takes into consideration tumor size and metastasis. Other tumor characteristics are associated with poor survival, including gross and microscopic evidence of lymphatic vessel invasion. Additional tumor characteristics, particularly expression levels of oncogenes and tumor suppressors, have been associated with favorable ( EGFR mutant and ERCC1 , RRM1 overexpression) and unfavorable ( Kras , p53 mutations and p53 , Her2 overexpression) prognostic value in early NSCLC. A rapidly growing list of biomarkers can be used to predict response to therapy, particularly because the available therapies are tailored to specific tumor mutations and characteristics expand. Among this list is expression of immune antigens and ligands (such as PD-1/PD-L1 and CTLA-4 expression), tumor characteristics such as mutational burden, and presence of tumor infiltrating lymphocyte. Characterization and quantification of circulating tumor cells, which increase with advancing stages of lung cancer, have been used to evaluate for response to therapy and to diagnose early, preclinical recurrence. , Similarly, circulating tumor DNA (ctDNA) has been used to diagnose and predict response to targeted therapies. , Many of these biomarkers are covered in another article in this issue.
Current utilization of biomarkers
Many lung cancer biomarkers are currently in development, most in the early stages ( Table 1 ), with few progressed beyond clinical validation studies ( Table 2 ). Most biomarkers designed for use as risk prediction and diagnostic biomarkers have been developed using histologically agnostic cohorts. However, most available specimens represent NSCLCs, particularly adenocarcinomas and squamous cell carcinomas as the most common histologic classifications, with small cell and other less common lung cancers less commonly the focus of biomarker development or utilization. Many lung cancer biomarkers have been used to guide therapy, such as when genetic alterations driving tumor growth can be targeted by available drug therapies (EGFR, ALK, ROS1, HER2, BRAF/MEK, MET, and RET mutation and aberrancies), to prognosticate tumor aggressiveness or response to treatment, or to predict response to immune checkpoint inhibition (PD-L1, CTLA-4 expressions, genomic alterations). , Most other molecular biomarkers, which have reached the clinical validation phase of development, have been designed for patient selection in LCS or to guide lung nodule management (see Table 2 ). These biomarkers measure different molecules, often in panels that include proteins, autoantibodies, methylated DNA, mRNA, miRNA, and single nucleotide polymorphisms alone or in combination with clinical factors. Most of the early biomarkers were developed using blood components, collected at the time of routine blood sampling and preserved, relying on systemic changes caused by lung cancer. , Two assays were developed for use in bronchial epithelial specimens obtained during diagnostic bronchoscopy performed to evaluate pulmonary nodules, which rely on altered genetic and epigenetic characteristics of noncancerous bronchial epithelial cells in patients with lung cancer (field cancerization effect). Some of these tests are commercially available, and a few are approaching clinical utility testing. , ,
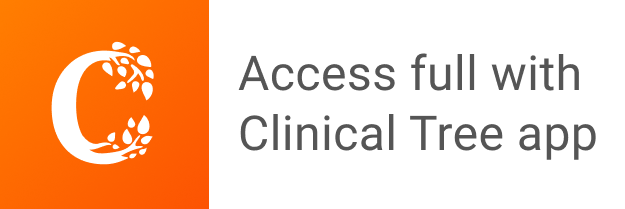