Background
The aim of this study was to compare the feasibility, accuracy, and reproducibility of automated quantification of mitral inflow and aortic stroke volumes (SVs) using real-time three-dimensional volume color-flow Doppler transthoracic echocardiography (RT-VCFD), with cardiac magnetic resonance (CMR) imaging as the reference method.
Methods
In 44 patients (86% of the screened patients) without valvular disease, RT-VCFD, CMR left ventricular short-axis cines and aortic phase-contrast flow measurement and two-dimensional (2D) transthoracic echocardiography (TTE) were performed. Dedicated software was used to automatically measure mitral inflow and aortic SVs with RT-VCFD. CMR total SV was calculated using planimetry of short-axis slices and aortic SV by phase-contrast imaging. SVs by 2D TTE were computed in the conventional manner.
Results
The mean age of the included patients was 40 ± 16 years, and the mean left ventricular ejection fraction was 61 ± 9%. Automated flow measurements were feasible in all study patients. Mitral inflow SV by 2D TTE and RT-VCFD were 85.0 ± 21.5 and 94.5 ± 22.0 mL, respectively, while total SV by CMR was 95.6 ± 22.7 mL ( P < .001, analysis of variance). On post hoc analysis, mitral inflow SV by RT-VCFD was not different from the CMR value ( P = .99), while SV on 2D TTE was underestimated ( P = .001). The respective aortic SVs were 82.8 ± 22.3, 94.2 ± 22.3, and 93.4 ± 24.6 mL ( P < .001). On post hoc analysis, aortic SV by RT-VCFD was not different from the CMR value ( P = .99), while SV on 2D TTE was underestimated ( P = .006). The interobserver variability for SV measurements was significantly worse for 2D TTE compared with RT-VCFD.
Conclusions
RT-VCFD imaging with an automated quantification algorithm is feasible, accurate, and reproducible for the measurement of mitral inflow and aortic SVs and is superior to manual 2D TTE–based measurements. The rapid and automated measurements make this technique practical in the clinical setting to measure and report SVs routinely where the acoustic window will allow it, which was 86% in our study.
Stroke volume (SV) and cardiac output measurements are important parameters in the assessment of cardiac function, shunt fractions, and the severity of valve disease. With two-dimensional (2D) transthoracic echocardiography (TTE), pulsed-wave (PW) Doppler of the left ventricular (LV) outflow tract (LVOT) and mitral inflow can be used to measure SV. However, this technique is limited by inaccuracies in measurements of the diameter of the LVOT and the mitral annulus, assumptions of uniform orifice geometry and flow profile, and angle dependency of the spectral Doppler measurements.
Recently, three-dimensional (3D) color-flow Doppler (CFD) has been used to measure SV and cardiac output. This technique uses retained velocity assignments in the CFD data within an operator-defined area, over a cardiac cycle, to calculate SV. Although initially described using reconstructive 3D techniques, recent work has demonstrated feasibility with real-time 3D CFD echocardiography. Previous studies have shown that the use of hemispheric flow sampling planes to measure flows at the mitral annulus or LVOT overcomes the need for Doppler angle correction, assumptions about orifice geometry are not required because the entire color flow area is used, and sampling over the entire orifice area eliminates the need for assumption of uniform flow profile within the orifice. Furthermore, various dealiasing methods have been used to overcome the intrinsic limitation of color Doppler imaging. SV measurements using the 3D CFD technique have been shown to correlate well with those made by thermodilution and cardiac magnetic resonance (CMR) imaging. However, all these previous studies have used gated 3D CFD data, which can be tedious to acquire and prone to errors and cannot be obtained in patients with arrhythmias. Furthermore, gated 3D CFD consists of data acquired over 7 to 14 cardiac cycles, so the SV obtained is not truly a single-beat SV. Also, none of the previous studies measured mitral and aortic SVs simultaneously in a single 3D volume. These factors and the fact that the postprocessing has required time-consuming and often irreproducible manual interaction with the data have impeded the clinical adoption of 3D CFD for SV measurements in routine practice.
Real-time 3D volume CFD TTE (RT-VCFD) is a new technique in which full-volume, B-mode, and color Doppler can be obtained simultaneously and continuously with up to 90° × 90° volume sector in every heartbeat for any number of beats. The velocity information encoded in the volume color Doppler data combined with simultaneous 3D B-mode imaging of the mitral annulus and LVOT make it possible to directly quantify mitral inflow and aortic SVs in the same cardiac cycle. An automated algorithm can be used to detect anatomic landmarks and compute flow through the mitral valve and LVOT using the real-time 3D B-mode and CFD data.
The aims of this study were to (1) assess the feasibility of an automated technique with RT-VCFD imaging to quantify mitral inflow and aortic SVs and (2) compare the accuracy and reproducibility of automated 3D SVs with those of manually calculated SVs using 2D TTE and PW Doppler at the mitral valve and LVOT. CMR was the independent reference standard.
Methods
Patient Population
Consecutive patients aged > 18 years referred to the CMR laboratory for assessment of ventricular function without valvular disease or other structural heart disease were recruited to participate in this institutional review board–approved study. Patients with atrial fibrillation, intracardiac shunts, or poor echocardiographic windows were excluded. Poor echocardiographic windows were defined as the inability to image the entire ventricle without dropouts or artifacts or the inability to visualize more than two myocardial wall segments. Patients with atrial fibrillation and intracardiac shunts were excluded because both these conditions would invalidate the use of CMR as a reference standard.
Two-Dimensional Transthoracic Echocardiographic Acquisition and Analysis
Two-dimensional transthoracic echocardiographic images in the parasternal long-axis and apical views were obtained using the Siemens Acuson SC2000 ultrasound system (Siemens Medical Solutions USA Inc., Mountain View, CA), using the 4V1c transducer (4.5 MHz). This was followed by PW Doppler measurements at the mitral annulus (four-chamber view) and LVOT (five-chamber view) to obtain velocity-time integrals. The Doppler measurements were made at the same anatomic location at which the LVOT and mitral annular measurements were obtained. Mitral annular diameter was measured in the four-chamber view, while the LVOT diameter was measured in the parasternal long-axis view. The mitral annular and LVOT diameters and the PW Doppler velocity-time integral were all measured for three consecutive cardiac cycles and averaged for calculations ( Figure 1 ). Two-dimensional and velocity-time integral measurements and calculations of SVs were performed per American Society of Echocardiography guidelines by reviewers blinded to CMR and RT-VCFD data.

RT-VCFD Acquisition
RT-VCFD images were obtained using the Siemens Acuson SC2000 imaging system with a 4Z1c real-time volume imaging transducer (2.8 MHz). The transducer allows 3D acquisition with up to a 90° × 90° pyramid of B-mode and CFD data simultaneously. B-mode and CFD data of the left ventricle were acquired in every cardiac cycle for three to five consecutive cycles in the apical three-chamber or five-chamber view, incorporating the LVOT and mitral valve in a single volume ( Video 1 ; view video clip online). During acquisition, three 2D reference planes are simultaneously displayed to ensure that the regions of interest (ROIs) are contained within the volume. The color Doppler velocity scale was maximized before data acquisition to minimize aliasing. Color bleeding was minimized by adjusting the B-mode gain setting to optimize structure delineation and then by adjusting the color gain to optimally fill these structures with blood flow. Depth setting was optimized to ensure highest volume rates while ensuring that at least one third of the atrium was in the imaging volume. The total acquisition time was 3 to 5 sec, depending on the patient’s heart rate. After acquisition, the Digital Imaging and Communications in Medicine data were transferred to a desktop computer for offline analysis using prototype software (Siemens Medical Solutions USA Inc.) that has been previously validated.
RT-VCFD Automated SV Measurement
A detailed mathematical basis of the automated flow quantification algorithm has been previously described. The essential steps are outlined here. The first step was the automated detection of the 3D LV endocardial surface, the mitral annulus, and the LVOT in the first frame of the volume data ( Figure 2 A and 2B). Automation is achieved by learning a model of the LV anatomic landmarks from a large training set of expert annotated example images using a marginal space learning approach. Marginal space learning is a computationally efficient way of learning the specific unique 3D image features that are characteristic of the anatomic landmark parameters. Instead of learning the features in the full anatomy parameter space, marginal space learning simplifies the problem by incrementally learning in projected (marginal) subspaces. These anatomic landmarks were then dynamically tracked by efficient computer algorithms throughout the cardiac cycle for each of the acquired cycles using information from multiple cues, such as optical flow, boundary detection, and motion prior. Optical flow is a method of image intensity displacement tracking between two frames, boundary detection identifies the learned 3D image features associated with the LV border, and motion prior is a learned statistical motion model of the LV border. These cues are fused on the basis of their confidence scores by computer algorithms to determine the tracked location of the anatomic landmarks.

The next step involved a placement of 3D hemispheric flow sampling planes ( Figure 2 C and 2 D) for color Doppler velocity measurements along the ultrasound beam direction. The locations of these planes were dynamically adjusted throughout the cardiac cycles on the basis of tracking of the anatomy of the mitral annulus and the LVOT. The use of a hemispheric 3D sampling plane ( Figure 2 E and 2 F) eliminated the need for flow direction–based angle correction of color Doppler velocities, while automated frame-by-frame adjustment of the sampling plane ensured spatially consistent measurement of velocities. At each location, although only one sampling plane was displayed ( Video 2 ), the flow sampling was performed using three parallel sampling planes spaced 1 to 2 mm apart ( Figure 2 E and 2 F). The mitral inflow and LVOT flow rate were computed as an average of the flow rates at the three planes to minimize the impact of the variation in SV due to the location of sampling. The resolution of the automated technique was dependent on the aperture of the transducer and user-selectable settings such as frequency, imaging depth, color ROI size, and the scan-line density, which was a trade-off between spatial and temporal resolution. For a typical system setting with 15 to 17 cm depth, color ROI size covering both the aortic and mitral valves, and temporal resolution of 14 to 33 volumes/sec, the acquired color Doppler voxel dimension at the sampling plane was 4.5 × 5.0 × 0.6 mm in the azimuthal, elevational, and range directions. After interpolation and scan conversion, the rendered voxel dimension was about 0.4 mm isotropic ( Figure 3 A) corresponding to approximately 1,800 to 3,600 voxels at each of the aortic and mitral sampling planes. The cross-sectional sizes of the sampling planes are determined by the detected LV anatomy (i.e., opening in the LV endocardial mesh), the position of the planes, and the cross-sectional area of the color Doppler flow. The algorithm aggregates instantaneous flow velocities sampled at each voxel in the sampling planes to compute flow rates. Next, end-diastole and end-systole are determined using electrocardiographic information in the Digital Imaging and Communications in Medicine data. The beginning and end of mitral and aortic flows were detected using the electrocardiogram as well as the timing of the spatial presence of color Doppler flows across these locations. These timings determined the time interval over which temporal integration of the flow-time curve was performed to calculate the mitral and aortic SVs.

Automated dealiasing was performed at each frame as necessary to correct for Doppler velocity ambiguity. Dealiasing was determined on the basis of the principle of conservation of mass, which states that the change in LV volume and the change in flow volume (across either the mitral annulus or the LVOT) describe the same volume of blood flowing through the left ventricle at a given instant in time. A dealiasing factor was calculated as a ratio of the instantaneous LV volume change between two neighboring frames to flow volume change at the mitral annulus (during diastole) or the LVOT (during systole) during the same two frames. This dealiasing factor was then automatically applied to each frame if aliased flow was detected by the algorithm.
The mitral inflow and aortic outflow rate curves were then displayed as a function of time (or frames) for every consecutive cardiac cycle in the acquisition ( Figure 3 B). The flow volumes were computed as areas under the curves for both the mitral inflow and the aortic outflow flow rate curves. The mitral inflow and aortic outflow SVs were displayed individually and as averages of all the cardiac cycles.
The entire automated process is illustrated dynamically in Video 2 . The time required to obtain mitral and aortic SVs for three to five cardiac cycles in a fully automated manner is 20 to 40 sec. The most common additional adjustment needed was operator-defined additional automated dealiasing on the basis of the profile of the automatically generated flow curves, especially for the LVOT ( Figure 4 A–D). Even with minor adjustments, the total time taken to compute 3D SV for three to five cycles was 40 to 60 sec.

CMR Data Acquisition and Analysis
The CMR study was performed using a 1.5-T magnet (Magnetom Avanto; Siemens Medical Solutions USA Inc.) using a 12-channel phased-array coil. Short-axis cines covering the left ventricle were acquired using a segmented balanced steady-state free precession sequence (repetition time, 3 msec; echo time, 1.3 msec; bandwidth, 930 Hz/pixel; flip angle, 65°; effective temporal resolution, 20–25 frames/cardiac cycle; 8-mm short-axis slices; interslice distance, 20%). LV end-diastolic and end-systolic volumes were quantified by manual contouring of endocardial borders (Argus, Siemens Medical Solutions, USA, Inc.) and Simpson’s method as per guidelines ( Figure 5 ). LV total SV was calculated by subtracting LV end-systolic volume from LV end-diastolic volume. The total SV by CMR was used for comparison with mitral inflow SV by echocardiography, because this is likely a more accurate measure of mitral inflow SV than aortic SV given that trivial mitral regurgitation is common in many patients. Aortic SV was obtained using through-plane phase-contrast imaging with the imaging plane prescribed 2 to 3 cm above the aortic valve in an orientation orthogonal to the aortic root (repetition time, 53.3 msec; echo time, 2.1 ms; bandwidth, 401 Hz/pixel; flip angle, 25°; slice thickness, 6 mm; 20 frames/cardiac cycle; aliasing velocity, 150 cm/sec). An ROI was drawn to cover the flow area and propagated to all the frames ( Figure 5 ). Each frame was evaluated individually to ensure that the ROI accurately encompassed the flow region. An ROI was also placed on the chest wall closest to the aorta and propagated through all slices and used for phase offset error correction. The aortic SV was recorded.

Statistical Analysis
Continuous data are expressed as mean ± SD and categorical data as frequencies or percentages. Correlations between variables were assessed using linear regression analysis and Pearson’s correlation coefficient. Mean SVs computed by 2D TTE, RT-VCFD, and CMR were compared using one-way repeated measures analysis of variance with post hoc t tests with Bonferroni’s correction. Interobserver and intraobserver variability was tested using Bland-Altman analysis and as a quotient of the summed absolute value of the difference and summed means of the SV values calculated by the two blinded observers. MedCalc version 11.0.1.0 (MedCalc Software, Mariakerke, Belgium)) was used for statistical analysis. Statistical significance was set at a two-tailed P value < .05
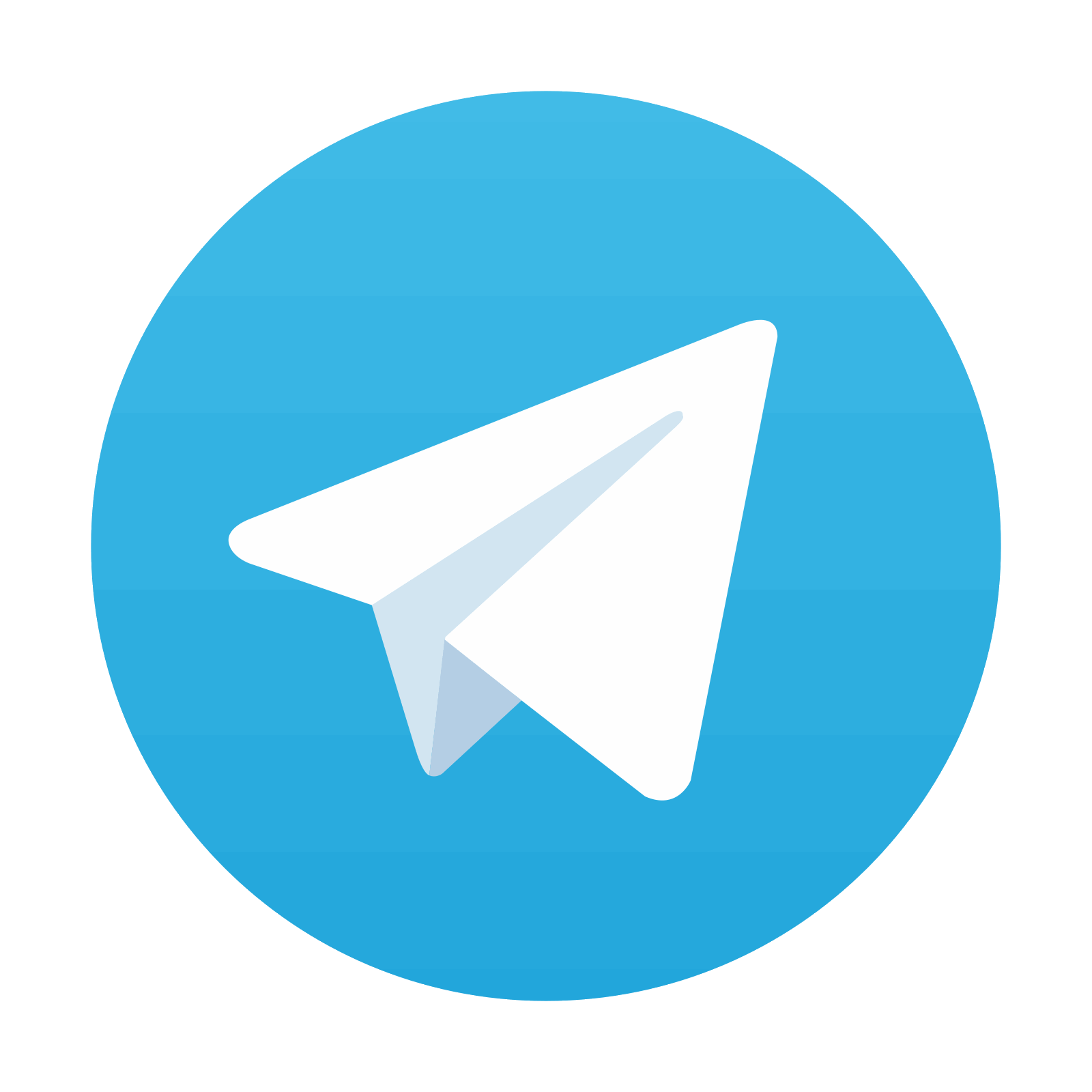
Stay updated, free articles. Join our Telegram channel

Full access? Get Clinical Tree
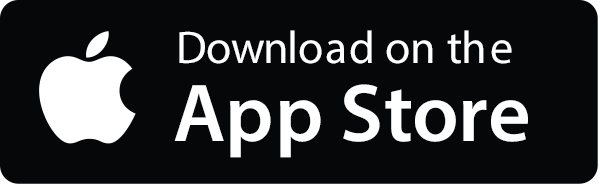
