, Dilip R. Karnad2 and Snehal Kothari3
(1)
Cardiac Safety Services Quintiles, Durham, North Carolina, USA
(2)
Research Team, Cardiac Safety Services Quintiles, Mumbai, India
(3)
Cardiac Safety Services Global Head, Cardiac Safety Center of Excellence Quintiles, Mumbai, India
Our interest in the discipline of Statistics is a pragmatic one since it provides the best way currently available to conduct clinical development programs (Turner 2010).
4.1 Introduction
When faced with the myriad challenges in drug development, the discipline of Statistics (recognized by the use of an upper case “S”) is “the knight in shining armor” that rides to our assistance and facilitates the collection, analysis, and interpretation of optimal-quality data as the basis for rational decision-making at all stages of the process (Durham and Turner 2008). In this and the following two chapters, we have resisted the temptation to provide exhaustive discussion of subtle nuances of statistical analysis: our interest in the discipline of Statistics is a pragmatic one since it provides the best way currently available to conduct clinical development programs.
We noted in Chap. 1 that, since no biologically active drug is free from the possibility of causing adverse reactions in certain individuals who are genetically and/or environmentally susceptible, regulators have to balance a drug’s therapeutic benefit with its toxicity to assess the drug’s benefit–risk balance. Therefore, it is important to have a fundamental understanding of assessments of benefit, which is called efficacy in clinical trials. Quantitative information concerning both efficacy and safety provides the rational basis for evidence-based decision-making, both by sponsors throughout their drug development programs and by regulators when sponsors apply for marketing approval.
When planning clinical trials, two considerations are of critical importance. First, the statistical analyses that will eventually be conducted must be planned at the design stage of the study. Second, the desired goal, i.e., approval of a new drug by regulatory agencies, is known from the outset. Regulatory agencies provide enormous amounts of detailed guidance for the conduct and reporting of drug development research. Guidance documents should therefore be studied before starting the drug development program.
4.2 Categorization of Clinical Trials
Clinical trials are often categorized into four phases, with a given trial being identified as belonging to one of them. Traditional descriptions are as follows:
Phase I: These are pharmacologically oriented studies that typically look for the best dose(s) to employ in subsequent trials. Comparison with other treatments is not the first priority, although a small control group is often included in trial designs. The focus here is on safety.
Phase II: These trials are usually performed in individuals with the clinical condition of interest, and look for evidence of biological activity, efficacy, and safety.
Phase III: Comparison with another treatment (which can be a placebo or a different active drug) is a fundamental component of the design of these trials. They are conduced if a sponsor believes that Phase I and Phase II trials have provided preliminary evidence that the new treatment is safe and effective. If the sponsor believes that the entire data set from Phase I, II, and III trials provide compelling evidence of the drug’s safety and efficacy, a marketing application will be submitted.
Phase IV: Phase IV trials are conducted following a drug successfully receiving marketing approval. As the Institute of Medicine of the National Academies (IOM) has stated, “The [marketing] approval decision does not represent a singular moment of clarity about the risks and benefits associated with a drug – preapproval clinical trials do not obviate continuing formal evaluations after approval” (IOM 2007). Along with postmarketing surveillance, a topic addressed in Chap. 14, postmarketing trials are therefore of great importance.
A more informative alternative system of categorization was provided by the ICH: their nomenclature, presented in Table 4.1, is much more descriptive of the nature of a given trial.
Table 4.1
The ICH categorization of clinical trials
Objective(s) of study | Study examples |
---|---|
Human pharmacology trials | |
Assess tolerance Describe or define pharmacokinetics (PK) and pharmacodynamics (PD) Explore drug metabolism and drug interactions Estimate [biological] activity | Dose tolerance studies Single- and multiple-dose PK and/or PD studies Drug interaction studies |
Therapeutic exploratory studies | |
Explore use for the targeted indication Estimate dosage for subsequent studies Provide basis for confirmatory study design, endpoints, methodologies | Earliest trials of relatively short duration in well-defined narrow patient populations using surrogate of pharmacological endpoints or clinical measures Dose–response exploration studies |
Therapeutic confirmatory | |
Demonstrate/confirm efficacy Establish safety profile Provide an adequate basis for assessing benefit–risk relationship to support licensing [marketing approval] Establish dose–response relationship | Adequate and well-controlled studies to establish efficacy Randomized parallel dose–response studies Clinical safety studies Large simple trials Comparative studies |
Therapeutic use | |
Refine understanding of benefit–risk relationship in general or special populations and/or environments. Identify less common adverse reactions Refine dosing recommendation | Comparative effectiveness studies Studies of mortality–morbidity outcomes Studies of additional endpoints Large simple trials Pharmacoeconomics studies |
4.3 Statistical Significance
For a drug to be given marketing approval by a regulatory agency, regulators must decide that the sponsor has provided compelling evidence of its efficacy as well as its safety. Efficacy is assessed in two ways: the drug must be demonstrated to show statistically significant efficacy and also demonstrated to show clinically significant efficacy. The discipline of Statistics has provided methodologies suitable for both assessments. Assessment of statistical significance is addressed via formalized hypothesis testing requiring the creation of a research question, a research hypothesis, and a null hypothesis. Assessment of clinical significance is addressed via the employment of confidence intervals. In the latter case, the process is less formulaic in that it requires the employment of clinical judgment in conjunction with statistical methodology. However, it is the more important determination. Demonstration of statistically significant evidence of efficacy is a necessary condition for regulatory approval, but it is not a sufficient one: it is perfectly possible for a drug’s efficacy to attain statistical significance but not to be considered of clinical significance.
4.3.1 The Role of Probability in Efficacy Assessment
Efficacy evaluations are facilitated by the conduct of a randomized clinical trial that allows the comparison of responses to the drug under development (the test drug) with those to a control drug (a placebo or another active drug). An active control drug is used when it is considered unethical to deny participants in the control drug treatment arm some form of therapy, and in such cases the control drug is often the current standard of care for the indication of interest. The drug’s treatment effect, the measure of efficacy, is defined as the mean response of participants who received the test drug minus the mean response of participants who received the control. Imagine a hypothetical clinical trial comparing an antihypertensive medication with placebo. The mean response to the test drug is a reduction of 10 mmHg in systolic blood pressure (SBP), the endpoint of interest in the trial, and the mean response to the placebo is a mean reduction in SBP of 2 mmHg. The calculation conducted is “10 – 2,” yielding a value of 8. The test drug’s treatment effect is therefore 8 mmHg.
A very reasonable question arises here. By definition, a placebo is a substance that is not biologically active, and it therefore cannot have had any pharmacological influence on SBP. However, it is a truism of clinical trials that the mean response to a placebo is often a smaller response in the same direction as the response to the test drug. That is, simply being in the clinical trial environment can lead to a small “improvement” in the biological endpoint of interest (the Hawthorne effect). It is therefore accepted practice to define and calculate the test drug’s treatment effect in this manner rather than to regard the mean change produced by the test drug as the treatment effect with no consideration being paid to the mean response to the control drug.
Determination of the drug’s treatment effect is the first step of the analytical procedure to determine whether the drug’s efficacy attains statistical significance. The next step concerns determining the likelihood that a treatment effect of that magnitude (or greater) could have been obtained in the clinical trial by chance alone. If our determination is that it could have occurred by chance alone, we have little faith that we would get a similar result if we were to conduct a similar trial again, and therefore we do not have any degree of reasonable assurance that the drug would be likely to provide that degree of therapeutic benefit to patients if it were to be given regulatory approval. If our determination is that it is sufficiently unlikely that it could have occurred by chance alone, we have a much greater degree of assurance that the drug would likely provide that degree of therapeutic benefit to patients if given regulatory approval.
These considerations lead directly to the realm of probability. Probability is an important component of the analytical strategies utilized in the assessment of statistical significance. One commonly used level of probability in drug development is the 5 % level, a percentage version of odds of one in 20: if the odds of something occurring are one in 20, there is a 5 % chance that it will occur. Consider the following example. You toss a coin and it lands heads up repeatedly: what is the likelihood the coin has heads on both sides? Mathematically, if you toss a normal coin (one side is heads and the other side is tails) once, the chance of it landing heads is 50 %. If you toss it again, the chance of it landing heads up twice in succession is 1 in 4 (25 %). Similarly, the chance of it landing heads up three times in a row is 1 in 8 (12.5 %), four times in a row is 1 in 16 (6.25 %), five times in a row is 1 in 32 (3.13 %), six times is 1 in 64 (1.56 %), and so on. At what stage would you stop the experiment and say that this coin is definitely not normal and the continued occurrence of the coin landing heads up is not due to chance? The greater the number of tosses, the greater your certainty of being correct. However, based on the arbitrarily chosen but widely accepted convention in statistics, you would stop after 5 tosses, since the probability of getting 5 heads in a row is now 1 in 32, i.e., <5 %. This example also highlights that the level of probability selected to decide if the observations are due to chance can be moved in either direction if such a move can logically be justified.
A probability of 5 % can be expressed as p = 0.05. The statement “p < 0.05” (i.e., the probability is less than 0.05) means that the probability of something occurring is less than 5 %. Statistical analysis of the data from the hypothetical clinical trial just discussed will provide a probability value associated with the likelihood that a treatment effect of the magnitude attained (or greater) would have resulted by chance alone. Statistical convention states that if the probability value provided by the analysis is less than 5 %, i.e., p < 0.05, it is deemed that the magnitude of the treatment effect was not due to chance alone: rather, the size of the treatment effect was directly affected by a systematic influence (discussed further in the next section), and it attained statistical significance. That is, the test drug demonstrated a statistically significantly greater reduction in SBP than did the control drug.
The statement “p < 0.05” is likely the most recognized nomenclature in drug development. As an aside here, despite this prominence, the value of 0.05 was not ordained, but was conceived by the visionary statistician Sir Ronald Fisher. Had he decided, for example, that odds of 1 in 25, with an analogous p-value of 0.04, were more appropriate than odds of 1 in 20, modern science might be held to a different standard. Whether the value of 0.05 is “right” (whatever right means) is not the issue here: the important consideration is the acknowledgment that a particular value has been chosen and is honored by all stakeholders in the drug development endeavor.
An additional level of probability of interest is the 1 % level. A probability of 1 % can be expressed as p = 0.01. The statement “p < 0.01” (i.e., the probability is less than 0.01) means that the probability of something occurring is less than 1 %. If the probability value provided by an analysis is less than 1 %, i.e., p < 0.01, the result has attained a higher degree of statistical significance than the 5 % level.
4.3.2 Systematic Influence and Randomization
From the present perspective, therefore, a statistically significant result is regarded as a probabilistic statement that the result obtained was not a chance occurrence: rather, it was caused by a systematic influence on the data collected from the participants in the two treatment groups. The systematic influence of interest is that one group of participants received the drug, and the other group of participants received the control treatment. However, and very importantly, to be able to consider this potential source of influence as the systematic influence leading to the result obtained, it is necessary to mitigate to the greatest extent possible all other potential sources of systematic influence. This is achieved via two processes of key importance to discussions throughout this book: the process of randomization and the implementation of strict experimental control by treating participants in the two groups in an (ideally) identical manner with the single exception of receiving the test drug or the control drug.
Randomization involves randomly assigning participants to one of the treatment groups so that the many potential influences that cannot be controlled for (e.g., height, weight) or cannot be determined by observation (e.g., specific and relevant genetic influences) are likely to be as frequent in one treatment group as they are in the other. Randomization occurs after an individual’s eligibility for a clinical trial has been determined and before any experimental data are collected. Randomization facilitates the random assignment of trial participants to different treatment groups with the intent of avoiding any selection bias. That is, randomization means that potential sources of influence on the data other than receiving the drug treatment or receiving the control treatment have been randomly allocated to each treatment group and therefore cannot exert a systematic influence on the results of the trial.
In statistical nomenclature, the goal of randomization is to eliminate bias. This includes participant bias based on knowledge of which treatment group they have been assigned to and also investigator bias. Investigator bias is eliminated by preventing investigators from deliberately assigning participants to one treatment group or the other. The process of randomization is facilitated by the generation of a randomization list. This list is generated (often by a random-number generator) in advance of recruiting the first participant. The randomization list is generated under the direction of the trial statistician. To maintain the confidentiality necessary for a double-blind trial to be conducted, i.e., a trial in which neither the participants nor the investigators running the trial know which treatments participants are receiving, the list is not released to the trial statistician until the completion of the study.
In many trials, participants have an equal chance of receiving either the drug or control treatment. In these cases, randomization is described as occurring in a 1:1 ratio. Using statistical nomenclature, this ratio provides the most powerful method of determining whether the drug is indeed more effective than the control, and it is typically used in Phase II (therapeutic exploratory) and Phase III (therapeutic confirmatory) trials. However, in other settings, it is legitimate and more informative with regard to the specific aims of a given trial, to use other randomization ratios. For example, a ratio of 2:1 for treatment vs. control means that two-thirds of the participants would be randomized to the treatment group and one-third to the control group. While the statistical power to detect a difference between the groups is not as high as it would be if the number of participants in each group were equal, there is a salient advantage of such a randomization ratio: more safety data concerning the drug will be gained, since two-thirds of the total number of participants in the study will receive this treatment, instead of one-half in the case of a 1:1 ratio. Ratios such as 2:1 and 3:1 for treatment vs. control are often seen in Phase I (human pharmacology) trials. Other randomization ratios are also possible, such as in cases where several doses of a drug are being employed along with a control treatment: in this setting, a ratio of 1:1:1:1 indicates that participants are randomly assigned to one of four groups, e.g., three drug treatment groups (perhaps 10, 20, and 30 mg of the drug) and a control group.
4.3.3 A Case Study: The United Kingdom Medical Research Council’s Streptomycin Trial
Credit for conducting the first pharmaceutical randomized clinical trial is often given to a trial that was conducted before the Kefauver–Harris Amendments. The UK Medical Research Council’s Trial of Streptomycin for Pulmonary Tuberculosis was conducted by Sir Austin Bradford Hill and his colleagues (the Streptomycin in Tuberculosis Trials Committee, chaired by Dr Geoffrey Marshall) in the late 1940s (MRC Streptomycin in Tuberculosis Trials Committee 1948). The control treatment arm of the trial consisted of the standard of care at the time, which was bed rest. The streptomycin treatment arm consisted of bed rest plus intramuscular administration of 2 g⁄day of streptomycin, given in four injections at 6-h intervals. While it is true that control groups had been used in medical research prior to this trial, the method of allocating participants to one of two treatment groups had been alternate allocation, simply placing the next individual entering the trial in the alternate treatment group to the one entered by the previous individual (Yoshioka 1998). In this trial, participants were randomized to one of the two treatment arms via reference to a statistical series based on random sampling numbers: details of the series were unknown to any of the investigators or to the study coordinator. Compelling evidence of efficacy was provided, and streptomycin subsequently became the first antibiotic treatment for this disease.
4.3.4 An Illustrative Example of an Efficacy Analysis to Determine Statistical Significance
Clinical trials are conducted to answer a research question. ICH Guideline E8 comments as follows (ICH E8 1997):
Clinical trials should be designed, conducted, and analyzed according to sound scientific principles to achieve their objectives; and should be reported appropriately. The essence of rational drug development is to ask important questions and answer them with appropriate studies. The primary objectives of any study should be clear and explicitly stated.
For the sake of continuity with the hypothetical trial introduced in Sect. 4.3.1, imagine a sponsor is developing a new drug to lower SBP, i.e., an antihypertensive drug. A clinical trial to investigate the antihypertensive effects of the drug requires a research question. A general question such as “Is this drug good for people’s blood pressure?” is not useful in this context. A better research question (which will be refined further in due course) is “Does the new drug alter SBP more than placebo?” Once this research question has been formulated, two hypotheses are created, the research hypothesis and the null hypothesis.
A good research hypothesis has traditionally included four important elements: population, intervention, control, and outcome (PICO for short). More recently, the question “When?” has also been added on to the outcome, if appropriate. The new research question could be reframed as “Does the new drug alter SBP more than placebo in individuals with mild essential hypertension after 7 days of treatment?” The research hypothesis typically reflects what is “hoped for,” which in this case is that the drug undergoing testing will indeed alter SBP. In strict scientific terms, hope has no place in experimental research: the goal is to discover the truth, whatever it may be, and one should not start out hoping to find one particular outcome. In the real world, this ideologically pure stance is not common for many reasons (financial reasons being not the least of them). The research hypothesis would therefore be stated as follows: the new drug alters SBP more than placebo in individuals with mild essential hypertension after 7 days of treatment.
The second hypothesis created is called the null hypothesis, which is the crux of hypothesis testing (it is sometimes presented first for this reason, followed by the research hypothesis, but order is not critical). The null hypothesis states that the new drug does not alter SBP more than placebo in individuals with mild essential hypertension after 7 days of treatment.
The process of hypothesis testing now takes place. Hypothesis testing revolves around two actions following an appropriate statistical analysis: rejecting the null hypothesis and failing to reject the null hypothesis. Statistical methodology necessitates a choice being made here, i.e., it is a forced choice paradigm. One of these two actions—rejecting the null hypothesis or failing to reject the null hypothesis—will occur at the end of all hypothesis testing. The action taken is determined by the statistical significance attained by the test statistic obtained in the statistical analysis.
Consider these results from a randomized, placebo-controlled Phase III trial of an antihypertensive drug. Participants were randomized in a 1:1 ratio to drug or placebo. The first step is to calculate the mean change score for each treatment group: in our ongoing example, these values are a mean decrease in SBP of 10 mmHg for the drug treatment group and a mean decrease in SBP of 2.00 mmHg for the placebo treatment group. The second step is to calculate the drug’s treatment effect. As first presented in Sect. 4.3.1, a drug’s treatment effect is defined as the mean response to the test drug (here, the antihypertensive) minus the mean response to the control (here, the placebo), and the treatment effect is therefore 8.00 mmHg.
The first point of interest is now established: the drug led to a mathematically greater reduction in SBP than did the control. The important question now becomes whether or not the drug led to a statistically significantly greater reduction in SBP. One form of analysis that is appropriate here is the independent-group t-test, an analysis that derives its name from the fact that the participants receiving the test drug were different individuals from the participants receiving the control drug. Analysis of the data will result in a value associated with the test statistic in this particular statistical test, which is called t. The magnitude of the test statistic will be associated with a p-value, and the magnitude of t will have to reach a certain size for the result to attain statistical significance. Imagine that the p-value associated with the test statistic is less than 5 %, i.e., p < 0.05. We therefore reject the null hypothesis and declare that the result attained statistical significance: this allows us to state that the test drug led to a statistically significantly greater reduction in SBP than the control treatment.
4.3.5 Factors Influencing the Attainment of Statistical Significance
As noted in Sect. 4.2, comparison with another treatment (a placebo or an active control) is a fundamental component of the design of Phase III (therapeutic confirmatory trials). In the previous section, we compared data from participants receiving the antihypertensive drug to those from participants receiving placebo. The treatment effect was a reduction in SBP of 8 mmHg, and this result attained statistical significance. Three fundamental aspects of the data set resulting from this trial govern whether or not statistical significance was obtained:
- 1.
Between-groups variation in the data. This is an overall measure of how different participants’ reductions in SBP in the drug treatment group are from those for the control group.
- 2.
Within-groups variation in the data. This is a measure of how different the individual reductions in SBP in the drug treatment group are from each other and how different the individual reductions in SBP in the control group are from each other.
- 3.
The total number of SBP reduction values collected in the trial. Since one SBP reduction value was obtained for each participant, this is effectively the total number of participants in the trial.
Consider the basic task being performed by the statistical analysis in these simple terms: we want to know if one group of numbers (SBP reductions in the drug treatment group) is different from a second set of numbers (SBP reductions in the control group). Consider a hypothetical example presented by Turner and Thayer (2001). Group A consists of five numbers and Group B consists of a second set of five numbers:
Group A: 47, 56, 44, 53, and 50
Group B: 54, 60, 66, 63, and 57
There are also two other groups of five numbers each:
Group C: 100, 70, 10, 20, and 50
Group D: 10, 90, 60, 95, and 45
Let’s compare Group A with Group B and also compare Group C with Group D. Simply from visual inspection, do you get the feeling that the group of numbers (data) in Group A is meaningfully different from the data in Group B? Similarly, do you get the feeling that the group of numbers (data) in Group C is meaningfully different from the data in Group D? Looking at Group A and Group B, you may think that numbers in both groups are very close to each other and that, overall, the numbers in Group B tend to be greater than the numbers in Group A since there is little overlap between the two groups. Looking at Group C and Group D, you may think differently, i.e., that there is a lot of overlap, and it is therefore difficult to get a good visual impression of to what degree the two groups differ from each other.
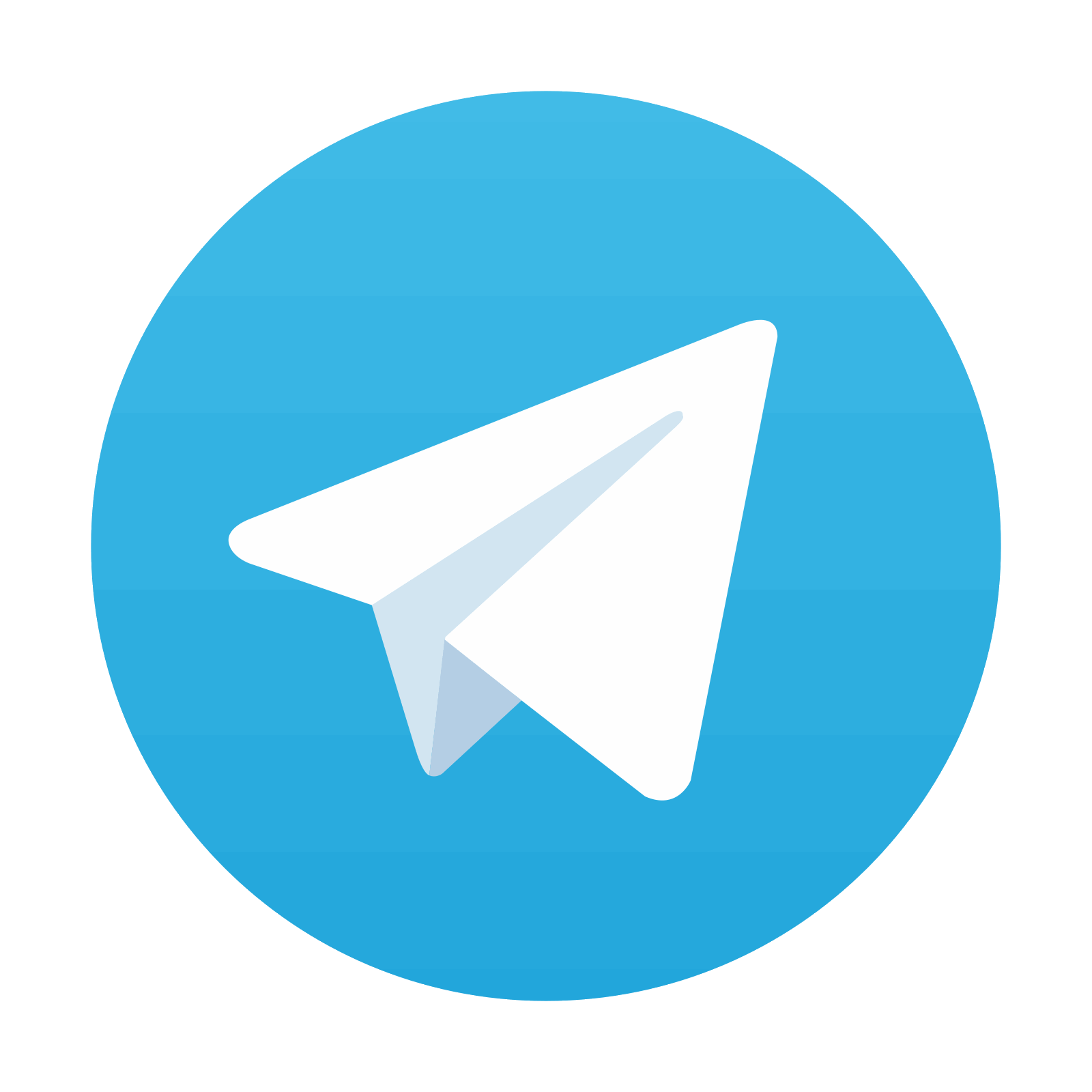
Stay updated, free articles. Join our Telegram channel

Full access? Get Clinical Tree
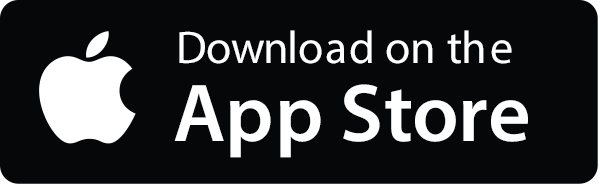
